CHAPTER 5 Outcomes Research
Outcomes research (clinical epidemiology) is the scientific study of treatment effectiveness. The word effectiveness is a critical one, because it pertains to the success of treatment in populations found in actual practice in the real world, as opposed to treatment success in the controlled populations of randomized clinical trials in academic settings (“efficacy”).1,2 Success of treatment can be measured using survival, costs, and physiologic measures, but frequently health-related quality of life (QOL) is a primary consideration.
Therefore, to gain scientific insight into these types of outcomes in the observational (nonrandomized) setting, outcomes researchers need to be fluent with methodologic techniques that are borrowed from a variety of disciplines, including epidemiology, biostatistics, economics, management science, and psychometrics. A full description of the techniques in clinical epidemiology3 is beyond the scope of this chapter. This chapter provides the basic concepts in effectiveness research and a sense of the breadth and capacity of outcomes research and clinical epidemiology.
History
In 1900, Dr. Ernest Codman proposed to study what he termed the “end results” of therapy at the Massachusetts General Hospital.4 He asked his fellow surgeons to report the success and failure of each operation and developed a classification scheme by which failures could be further detailed. Over the next 2 decades, his attempts to introduce systematic study of surgical end results were scorned by the medical establishment, and his prescient efforts to study surgical outcomes gradually faded.
Over the next 50 years, the medical community accepted the randomized clinical trial (RCT) as the dominant method for evaluating treatment.5 By the 1960s, the authority of the RCT was rarely questioned.6 However, a landmark 1973 publication by Wennberg and Gittelsohn spurred a sudden re-evaluation of the value of observational (nonrandomized) data. These authors documented significant geographic variation in rates of surgery.7 Tonsillectomy rates in 13 Vermont regions varied from 13 to 151 per 10,000 persons, even though there was no variation in the prevalence of tonsillitis. Even in cities with similar demographics and similar access to health care (Boston and New Haven, Conn.), rates of surgical procedures varied 10-fold. These findings raised the question of whether the higher rates of surgery represented better care or unnecessary surgery.
Researchers at the Rand Corporation sought to evaluate the appropriateness of surgical procedures. Supplementing relatively sparse data in the literature about treatment effectiveness with expert opinion conferences, these investigators argued that rates of inappropriate surgery were high.8 However, utilization rates did not correlate with rates of inappropriateness, and therefore did not explain all of the variation in surgical rates.9,10 To some, this suggested that the practice of medicine was anecdotal and inadequately scientific.11 In 1988, a seminal editorial by physicians from the Health Care Financing Administration argued that a fundamental change toward study of treatment effectiveness was necessary.12 These events subsequently led Congress to establish the Agency for Health Care Policy and Research in 1989 (since renamed the Agency for Healthcare Research and Quality, or AHRQ), which was charged with “systematically studying the relationships between health care and its outcomes.”
Key Terms and Concepts
Bias and Confounding
Bias occurs when “compared components are not sufficiently similar.”3 The compared components may involve any aspect of the study. For example, selection bias exists if, in comparing surgical resection to chemoradiation, oncologists avoid treating patients with renal or liver failure. This makes the comparison unfair because, on average, the surgical cohort will accrue more ill patients. Treatment bias occurs when comparing, for example, standard stapedotomy with laser stapedotomy, but one procedure is performed by an experienced surgeon, and the other is performed by resident staff.
Assessment of Baseline
Definition of Disease
In addition, advances in diagnostic technology may introduce a bias called stage migration.13 In cancer treatment, stage migration occurs when more sensitive technologies (such as CT scans in the past, and positron emission tomography scans now) may “migrate” patients with previously undetectable metastatic disease out of an early stage (improving the survival of that group), and place them into a stage with otherwise advanced disease (improving this group’s survival as well).14,15 The net effect is that there is improvement in stage-specific survival, but no change in overall survival.
Disease Severity
Recent progress has been made in sinusitis. Kennedy identified prognostic factors for successful outcomes in patients with sinusitis and has encouraged the development of staging systems.16 Several staging systems have been proposed, but most systems rely primarily on radiographic appearance.17–20 Clinical measures of disease severity (symptoms, findings) are not typically included. Although the Lund-Mackay staging system is reproducible,21 often radiographic staging systems have correlated poorly with clinical disease.22–26 As such, the Zinreich method was created as a modification of the Lund-Mackay system, adding assessment of osteomeatal obstruction.27 Alternatively, the Harvard staging system has been reproducible21 and may predict response to treatment.28 Scoring systems have also been developed for specific disorders such as acute fungal rhinosinusitis,29 and clinical scoring systems based on endoscopic evaluation have likewise been developed.30 The development and validation of reliable staging systems for other common disorders, as well as the integration of these systems into patient care, is a pressing challenge in otolaryngology.
Comorbidity
Comorbidity refers to the presence of concomitant disease unrelated to the “index disease” (the disease under consideration), which may affect the diagnosis, treatment, and prognosis for the patient.31–33 Documentation of comorbidity is important, because the failure to identify comorbid conditions such as liver failure may result in inaccurately attributing poor outcomes to the index disease being studied.34 This baseline variable is most commonly considered in oncology, because most models of comorbidity have been developed to predict survival.32,35 The Adult Comorbidity Evaluation 27 (ACE-27) is a validated instrument for evaluating comorbidity in cancer patients and has shown the prognostic significance of comorbidity in a cancer population.36,37 Because of its impact on costs, utilization, and QOL, comorbidity should be incorporated in studies of nononcologic diseases as well.
Assessment of Treatment
Control Groups
Reliance on case series to report results of surgical treatment is time-honored. It is also inadequate for establishing cause and effect relationships. A recent evaluation of endoscopic sinus surgery reports revealed that only 4 of 35 studies used a control group.38 Without a control group, the investigator cannot establish that the observed effects of treatment were directly related to the treatment itself.3
It is also particularly crucial to recognize that the scientific rigor of the study varies with the suitability of the control group. The more fair the comparison, the more rigorous the results. Therefore a randomized cohort study in which subjects are randomly allocated to different treatments is more likely to be free of biased comparisons than observational cohort studies in which treatment decisions are made by an individual, a group of individuals, or a health care system. Within observational cohorts, there are also different levels of rigor. In a recent evaluation of critical pathways in head and neck cancer, a “positive” finding in comparison with a historical control group (a comparison group assembled in the past) was not significant when compared to a concurrent control group.39
Assessment of Outcomes
Effectiveness
An efficacious treatment that retains its value under usual clinical circumstances is effective. Effective treatment must overcome a number of barriers not encountered in the typical trial setting. For example, disease severity and comorbidity may be worse in the community, in that healthy patients tend to be enrolled in (nononcologic) trials. Patient adherence to treatment may also be imperfect. Consider continuous positive airway pressure (CPAP) treatment for patients with obstructive sleep apnea. Although the CPAP is efficacious in the sleep laboratory, the positive pressure is ineffective if the patients do not wear the masks when they return home.40 A different challenge is present for surgical treatments, because community physicians learning a new procedure cannot be expected to perform it as effectively as the surgeon investigator who pioneered its development.
Fundamentals of Study Design
A variety of study designs are used to gain insight into treatment effectiveness. Each has advantages and disadvantages. The principal tradeoff is complexity versus rigor, because rigorous evidence demands greater effort. An understanding of the fundamental differences in study design can help interpret the quality of evidence, which has been formalized by the evidence-based medicine (EBM) movement. EBM is the “conscientious, explicit, and judicious use of current best evidence in making decisions about the care of individual patients.”41 EBM is discussed in detail elsewhere in this textbook. The following paragraphs summarize the major categories of study designs, with reference to the EBM hierarchy of levels of evidence (Table 5-1).41,42
Case-Control Study
For example, a prospective study of an association between a proposed carcinogen (e.g., gastroesophageal reflux) and laryngeal cancer would require a tremendous number of patients and decades of observation. However, by identifying patients with and without laryngeal cancer, and comparing relative rates of carcinogen exposure, a case-control study can obtain a relatively quick answer.43 Because the temporal relationship between exposure and outcome is not directly observed, no causal judgments are possible, however. These studies are considered level 3 evidence.
Other Study Designs
There are numerous other important study designs in outcomes research, but a detailed discussion of these techniques is beyond the scope of this chapter. The most common approaches include decision analyses,44,45 cost-identification and cost-effectiveness studies,46–48 secondary analyses of administrative databases,49–51 and meta-analyses.52,53 Critiques of these techniques are referenced for completeness.
Grading of Evidence-Based Medicine Recommendations
EBM uses the levels of evidence described earlier to grade treatment recommendations (Table 5-2).54 The presence of high-quality RCTs allows treatment recommendations for a particular intervention to be ranked as grade A. If no RCTs are available, but there is level 2 or 3 evidence (observational study with a control group or a case-control study), then the treatment recommendations are ranked as grade B. The presence of only a case series would result in a grade C recommendation. If only expert opinion is available, then the recommendation for the index treatment is considered grade D.
Table 5-2 Grade of Recommendation and Level of Evidence
Grade of Recommendation | Level of Evidence |
---|---|
A | 1 |
B | 2 or 3 |
C | 4 |
D | 5 |
Measurement of Clinical Outcomes
Clinical studies have traditionally used outcomes such as mortality and morbidity, or other “hard” laboratory or physiologic endpoints,55 such as blood pressure, white cell counts, or radiographs. This practice has persisted despite evidence that interobserver variability of accepted “hard” outcomes such as chest x-ray findings and histologic reports are distressingly high.56 In addition, clinicians rely on “soft” data, such as pain relief or symptomatic improvement to determine whether patients are responding to treatment. But because it has been difficult to quantify these variables, these outcomes have until recently been largely ignored.
Psychometric Validation
An important contribution of outcomes research has been the development of questionnaires to quantify these “soft” constructs, such as symptoms, satisfaction, and QOL. Under the Classical Test Theory, a rigorous psychometric validation process is typically followed to create these questionnaires (more often termed scales, or instruments). These scales can then be administered to patients to produce a numeric score. The validation process is introduced herein; a more complete description can be found elsewhere.57–59 The three major steps in the process are the establishment of reliability, validity, and responsiveness; in addition, increasing consideration is also given to burden.
More recently, item response theory (IRT) has been used to create and evaluate self-reported instruments. A full discussion of IRT is beyond the scope of this chapter. In brief, Item Response Theory uses mathematic models to draw conclusions based on the relationships between patient characteristics (latent traits) and patient responses to items on a questionnaire. A critical limitation is that IRT assumes that only one domain is measured by the scale. This may not fit assumptions for multidimensional QOL scales. However, if this assumption is valid, IRT-tested scales have several advantages. IRT allows for the contribution of each test item to be considered individually, thereby allowing the selection of a few test items that most precisely measure a continuum of a characteristic. In other words, because each test item is scaled to a different portion of the characteristic being tested, the number of questions can be reduced.65–68 Therefore, IRT lends itself easily to adaptive computerized testing, allowing for significantly diminished testing time and reduced test burden.65 In the future, IRT will likely be the basis to more and more new questionnaires evaluating outcomes, including QOL.
Categories of Outcomes
In informal use, the terms health status, function, and quality of life are frequently used interchangeably. However, these terms have important distinctions in the health services literature. Health status describes an individual’s physical, emotional, and social capabilities and limitations, and function refers to how well an individual is able to perform important roles, tasks, or activities.58 QOL differs because the central focus is on the value that individuals place on their health status and function.58
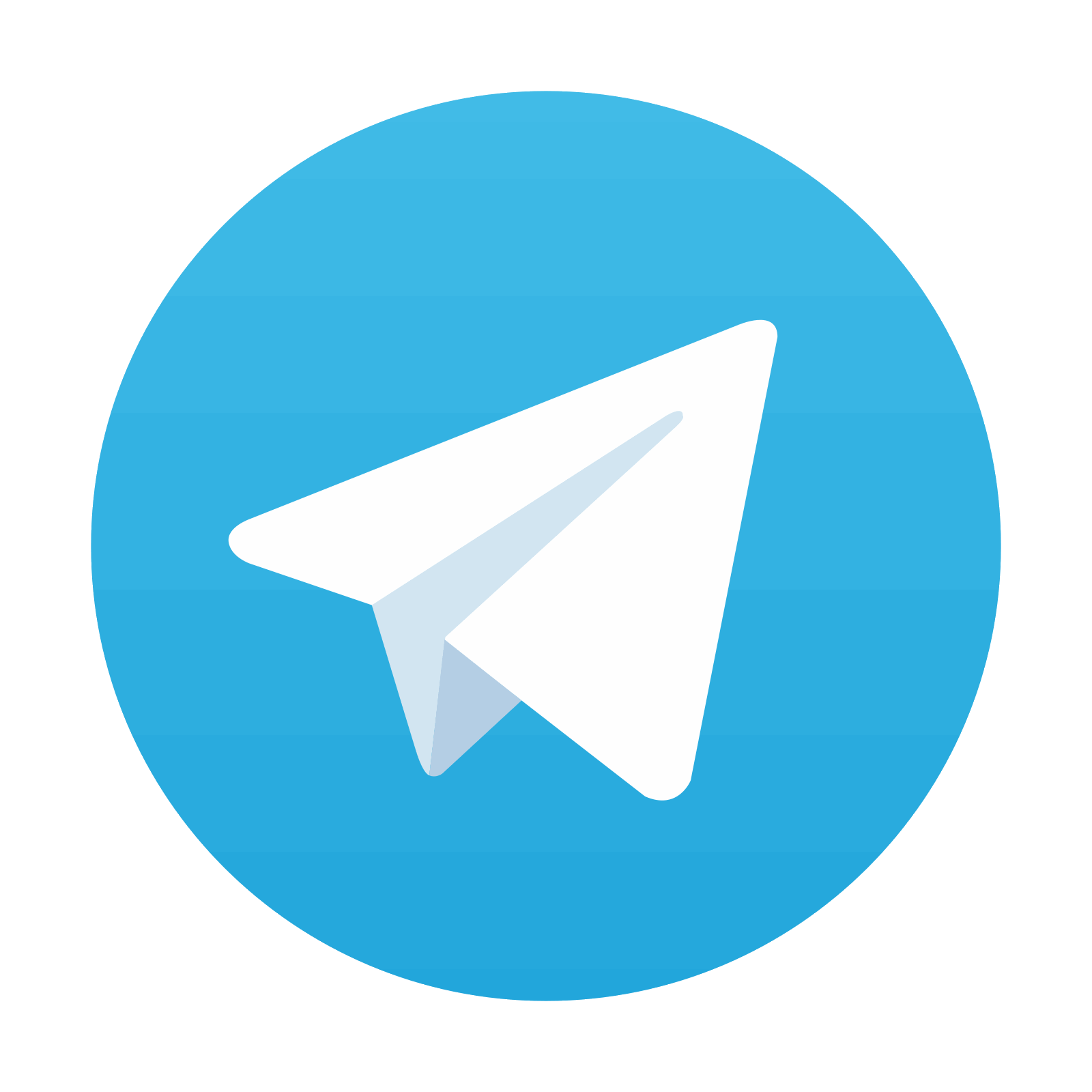
Stay updated, free articles. Join our Telegram channel

Full access? Get Clinical Tree
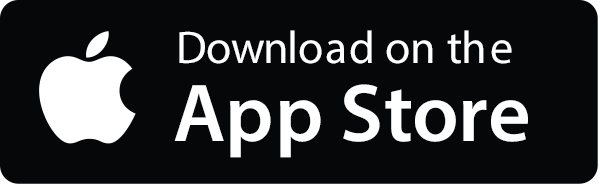
