Outcomes Research and Evidence-Based Medicine
Michael G. Stewart
INTRODUCTION AND HISTORY
Outcomes research can be defined as the scientific study of the outcomes of disease therapies used for a particular disease, condition, or illness (1). While all clinical research measures some type of outcome, such as mortality, morbidity, or some other objective measure, in “outcomes research,” the patient’s perception of their outcome is assessed.
Historically, the movement toward outcomes-based research was started by Dr. Paul Ellwood, who in the 1980s suggested that in the future physicians would assess outcomes by measuring what the patient experienced (2). Subsequently, tools to assess these outcomes were developed and applied across many diseases. However, outcomes research also includes other types of studies in addition to patient-based outcomes studies. Today, outcomes researchers study all aspects of the health care system—from the status of the patient or population at entry, to the organization, delivery, regulation and financing of the health care system, to the status of the patient or population after treatment.
To be inclusive of other aspects of health services research, some have divided outcomes research into record-based outcomes research and patient-based outcomes research. Examples of these different types of studies are shown in Table 8.1.
DEFINITION OF OUTCOME
Traditional clinical outcomes, such as survival and complication rate, are still assessed in outcomes research. However, emphasis is placed on expanded measures of outcome, which primarily are assessed from the patient’s perspective. These expanded outcomes include quality of life, global health status, and disease-specific health status. In addition, assessment of other factors that might impact outcome, such as comorbid disease, should also be assessed. Outcomes assessment is discussed in more detail later in the chapter.
Another significant difference between traditional clinical research and outcomes research is that outcomes studies are often performed in “real-world” settings using larger groups of patients versus traditional clinical research where typically smaller groups of patients are studied under very controlled environments.
TYPES OF STUDIES
Prospective, retrospective, or cross-sectional study designs can be used in outcomes research. However, many outcomes studies use an observational prospective design, where outcomes are assessed after diverse treatments—rather than an experimental prospective design, where treatments are carefully controlled or randomized. The differences between these study designs are an important point for discussion.
In experimental or controlled trials, particularly randomized trials, the ideal design uses two groups of patients that are nearly identical in every aspect—except the treatment received. So therefore, any difference in outcome between groups must be due only to the different treatments, since otherwise the groups were the same. Of course, acquiring patient groups that are actually identical is rarely achieved, but nevertheless, that is the basis behind the rigorous design and methodology of controlled trials. In addition, to account for the inherent differences between treatment groups, the randomization process should theoretically distribute those differences (say, in demographics or disease severity) equally between the two groups. Carefully controlled experimental studies can be said to measure the efficacy of treatment, under ideal circumstances. These studies usually yield very reliable results concerning the effects of treatment—in the group that was studied.
TABLE 8.1 TYPES OF OUTCOMES RESEARCH | |||||||||||||||||||||||||||||||||||||||
---|---|---|---|---|---|---|---|---|---|---|---|---|---|---|---|---|---|---|---|---|---|---|---|---|---|---|---|---|---|---|---|---|---|---|---|---|---|---|---|
|
However, there are questions about the generalizability of results from rigorously controlled trials to larger populations of patients with a disease because the larger group of patients will not be so carefully controlled and homogeneous. In addition, patient compliance and other factors may lead to different results than in tightly controlled trials. Furthermore, clinical trials are very expensive to perform, especially when considered from the standpoint of cost per patient studied.
Observational study designs are commonly used in outcomes research. All patients with a disease are included, and they are studied in the actual setting in which they receive their health care. This naturally introduces many other factors, which may impact outcome after treatment, including potential selection bias for different treatments. However, many would argue that results from large-scale outcome studies are more applicable to the general population because of their setting and scope. Studies that assess the actual (“real world”) results of treatment are said to measure the effectiveness of treatment versus efficacy measured from controlled trials (3). Furthermore, in addition to their “real-world” setting, the expanded outcome measured (quality of life, etc.) might be more relevant and important to patients than other clinical or biologic outcomes.
STEPS IN PERFORMING OUTCOMES RESEARCH
There are several basic steps involved in performing patient-based outcomes research (3,4,5). The fundamental steps are as follows: (a) identify and define the disease of interest, (b) create a staging system (clinical-severity index) for disease severity, (c) identify comorbid conditions, and (d) establish the outcomes to be measured (4). Studies can perform only one of those steps or some or all. Therefore, development and validation of an outcomes instrument is one type of outcomes research, and identification of comorbid conditions is another type. Each step is discussed in more detail below.
Define the Disease of Interest
This may be a straightforward step if the disease has clear and widely agreed upon diagnostic criteria. However, many diseases are difficult to rigorously define, such as gastroesophageal reflux or chronic rhinosinusitis, and research may be needed to develop clear reproducible diagnostic criteria.
Create a Staging System or Clinical-Severity Index
Grouping or stratification by disease severity is important in all types of clinical research. However, it is particularly important in outcomes research. This is because large numbers of patients may be studied without strict entry or exclusion criteria. Patients with more severe disease may receive more (or less) aggressive treatment, so it is therefore important to statistically adjust for disease severity when evaluating outcomes.
Staging systems already exist for many diseases, for example, the TNM staging system for cancer. It is important to distinguish between staging systems that are descriptive and systems that are prognostic. Descriptive staging systems simply group together patients who have similar characteristics. Prognostic systems are designed to predict an outcome; for example, the TNM staging system is designed to predict 5-year survival. In general, staging systems used for outcomes research should be prognostic (3). However, even if a prognostic staging system already exists, it may not contain all the important variables that predict outcome (5).
To develop a prognostic staging system, the researcher should first define the outcome of interest to be predicted by the staging system, which is defined as the dependent variable. Next, identify a group of variables that might predict outcome—those are the independent variables. Potential independent variables can be identified from prior literature, a prospective study, or expert opinion. Then next, perform a prospective study, identifying a heterogeneous group of subjects that is likely to contain patients with mild, moderate, and severe disease. In that group, measure the presence of all potential predictor variables and the outcomes after treatment.
Then, using data on both the outcome of interest and the presence of predictor variables, perform a multivariate analysis to identify which predictor variables actually impact outcome. Multivariate analysis is important in clinical studies because several different variables usually exert effects on each other, so it is preferable to study the effects of a large group of variables at the same time while controlling for the effects of the other potentially important variables.
Multivariate regression (linear or logistic) is one option for analysis; however, there are other options such as conjunctive consolidation (3,6,7). If regression is used, predictive factors are identified, and each can create a category with different outcomes. However, if multiple predictor variables are identified, the process of developing a single staging system can be difficult and at best requires multiple iterations of trial and analysis. The technique of conjunctive consolidation allows new clinical factors to be added to a staging system without necessarily increasing the number of groups or categories. Also, for development of a staging system, the data collection can be performed retrospectively, particularly if the outcome requires a significant time interval.
There are several potential “models” of staging systems from which to choose. Under any circumstances, developing a staging system is an iterative process in which patients are grouped by predictor variables, and the outcomes, by group, are assessed. If the groups are not sufficiently distinct, then another arrangement of predictor variables is used and outcomes by group are again compared. Ideally, the staging system should be organized so that patients are easily grouped into distinct strata, with clearly different outcomes, and such that all patients should be classifiable.
Identify Comorbid Conditions
The concept of a “comorbid” condition that seriously affected treatment outcome was first described by Dr. Alvan Feinstein. A comorbid condition is defined as a condition—distinct from the condition of interest—that affects the outcome being measured. For example, when measuring mortality from laryngeal cancer, if the patient has another serious condition causing potential mortality (i.e., unstable angina), then that condition is defined as a comorbid condition. Since the initial description, researchers in multiple specialties have identified the impact of comorbid disease on several different outcomes (6,7). Therefore, in any outcomes study, it is important to identify all potentially important comorbid conditions and to measure their presence and severity as part of the data collection process. Of course, that only applies if the comorbid condition actually affects the condition under study. Using the same example of unstable angina, if one were performing an outcomes study of hearing satisfaction 1 month after receiving different types of hearing aids, the presence of unstable angina would not necessarily be an important comorbid condition to consider.
Define the Outcomes to be Measured
The expanded, patient-based outcomes usually measured in outcomes research are quality of life, health status, and functional status. There are multiple potential definitions for each of those terms; however, “quality of life” has three key aspects: (a) it is more than the absence of disease, (b) it is subjective (assessed from the patient’s perspective), and (c) it is multidimensional. In addition, the overall quality of life depends on multiple aspects of life not directly related to disease, so most researchers studying treatment outcomes are actually assessing the “health-related quality of life.” Most outcomes instruments designed for use in patient care are designed to assess health-related quality of life. The term “health status” is self-explanatory, but again, it must be measured from the patient’s perspective. Functional status refers to the patient’s ability to perform daily activities. In most circumstances, researchers are only interested in the effect of a particular disease, so diseasespecific functional status is typically assessed.
To measure functional status or quality of life, the patient must answer several questions that have been validated for the purpose of measurement. Although these data can be gathered using interviews or other interactive techniques, under most circumstances patients complete a written questionnaire. In outcomes research, the questions are called “items” and the questionnaires are called “instruments.” Instruments must be validated, and the validation process uses the scientific principles of psychometrics. A full discussion of the process of instrument validation would require more than one chapter, although the basic concepts are reviewed.
A health status or quality of life instrument should be reliable, valid, and sensitive (8). Two types of reliability are usually assessed—test-retest reliability and internal consistency reliability. Test-retest reliability means that the results will be similar if the status of the patient has not changed, and internal consistency reliability means that responses on similar items will be correlated.
Validity means that the instrument is measuring what it is supposed to measure. Validity is confirmed by a combination of evidence: content validity, criterion validity (if scores on the instrument correlate with objective measurable external criteria), and construct validity (if scores on the instrument correlate with scores on other instruments measuring similar concepts).
Sensitivity (or responsiveness) means that the instrument is responsive to change in status. In other words, if the patient’s clinical status changes, then their score should also change. Sensitivity is assessed using statistical techniques measuring the degree of change against known standards, such as the standardized response mean and the effect size.
Another aspect of assessing sensitivity or change in status using an instrument has been called the “minimal significant difference” in score (3). For example, average scores on a health status instrument may change from 40 to 50 (on a scale of 0 to 100), and the difference might have a p-value less than 0.05. The question arises—is that 10-point difference a clinically
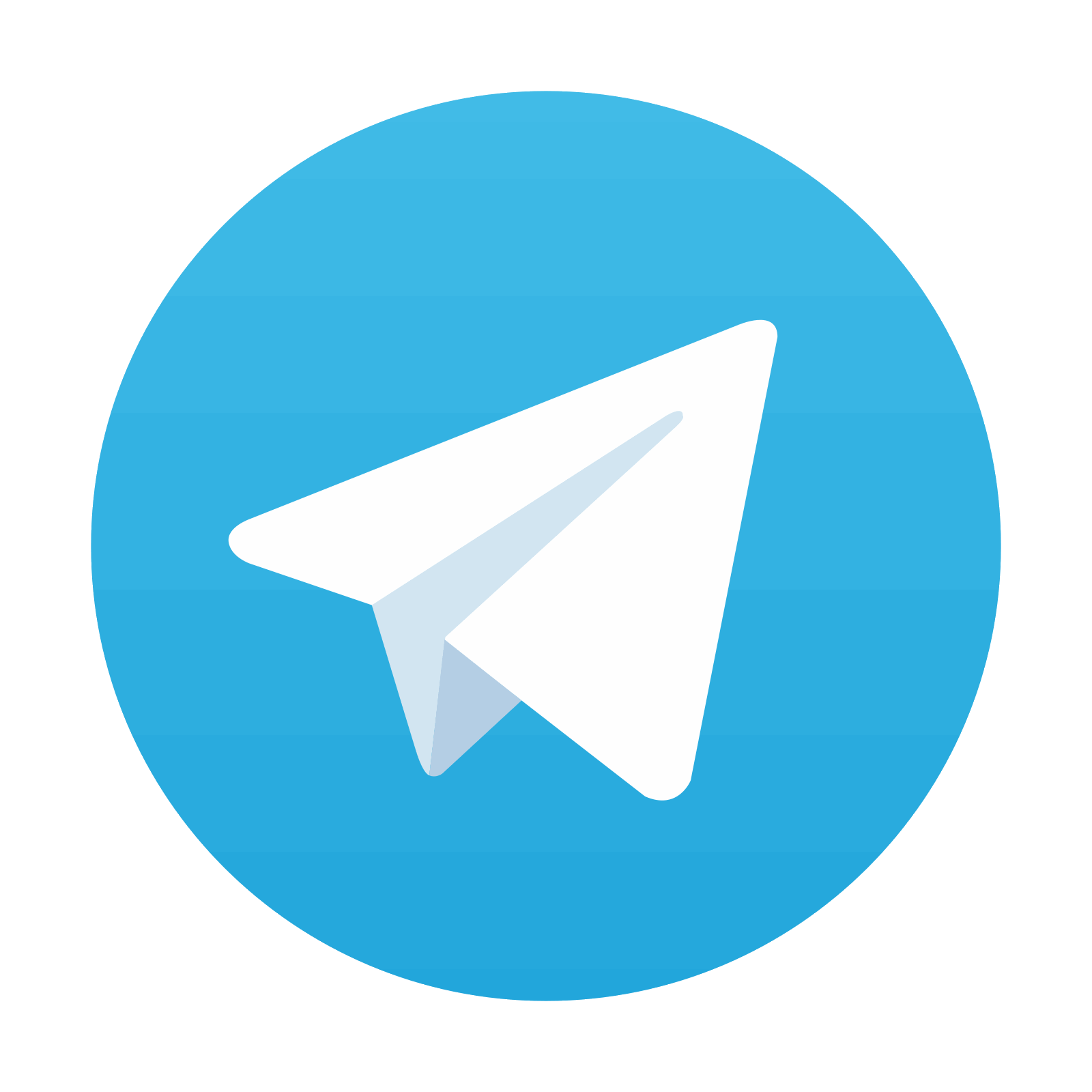
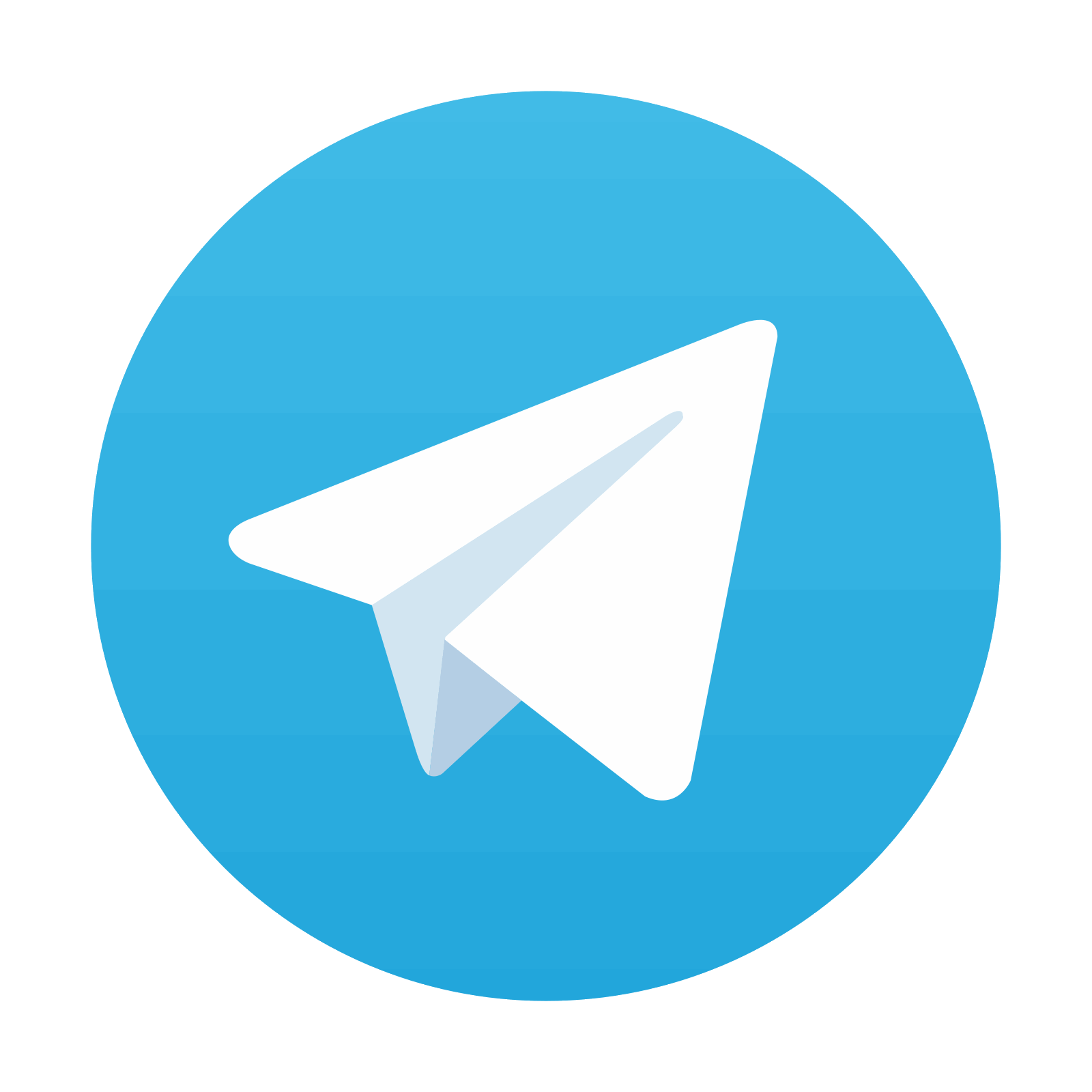
Stay updated, free articles. Join our Telegram channel

Full access? Get Clinical Tree
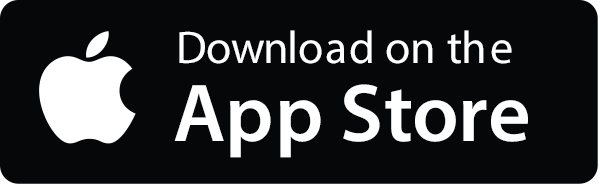

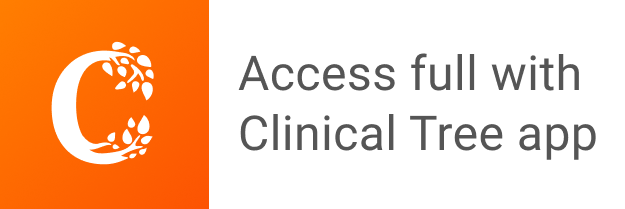