We appreciate the interest in our editorial. In their response to our editorial, Aristodemou and associates suggest we correctly state that the “absolute errors are not normally distributed,” then they go on to say that the editorial is therefore “implying that no statistical analysis is necessary.” This is contrary to what the editorial is stating and implying.
In the paragraph discussing how best to analyze bilateral eye data, generalized estimating equations (GEE) were suggested. This paragraph then went on to state: “When analyzing datasets that include subjects with 1 eye and others with 2 eyes, resampling techniques such as the bootstrap or GEE may be used. After using either of them, standard statistical tests such as t tests, regression, and the like may be performed.” The suggestion here was to use the bootstrapped estimates, specifically the bootstrap standard error rather than the traditional standard error, to perform statistical analyses.
Aristodemou and associates correctly state that transforming the data for normality or nonparametric tests would be the preferred approach, as the absolute errors are not normally distributed. However, transforming the data may or may not work (especially with a folded normal distribution) and older methods that are based on ranks (ie, Wilcoxon signed rank) tend to be underpowered; they tend to be less likely to detect a statistically significant difference. In addition, they are correct in stating that parametric methods are greatly affected by outliers. However, tests based on ranks suffer from the opposite problem in that they replace data points with ranks and therefore remove the effect of outliers. This may be problematic as outliers are often the most important data points in the study. In addition, tests based on ranks tend to be geared toward hypothesis testing rather than estimating parameters and it is not a straightforward exercise to obtain other useful statistics (eg, confidence intervals, etc).
The remedy we suggest is to use the bootstrapped estimates when performing t tests, confidence intervals, and the like. The bootstrap we suggest is a nonparametric bootstrap (there is a parametric version as well) and is based on a resampling of the data. The advantage of the bootstrap is that, along with being a distribution-free method (like the Wilcoxon signed rank test,) it is simple to implement even for complex estimators, and the results are easy to interpret.
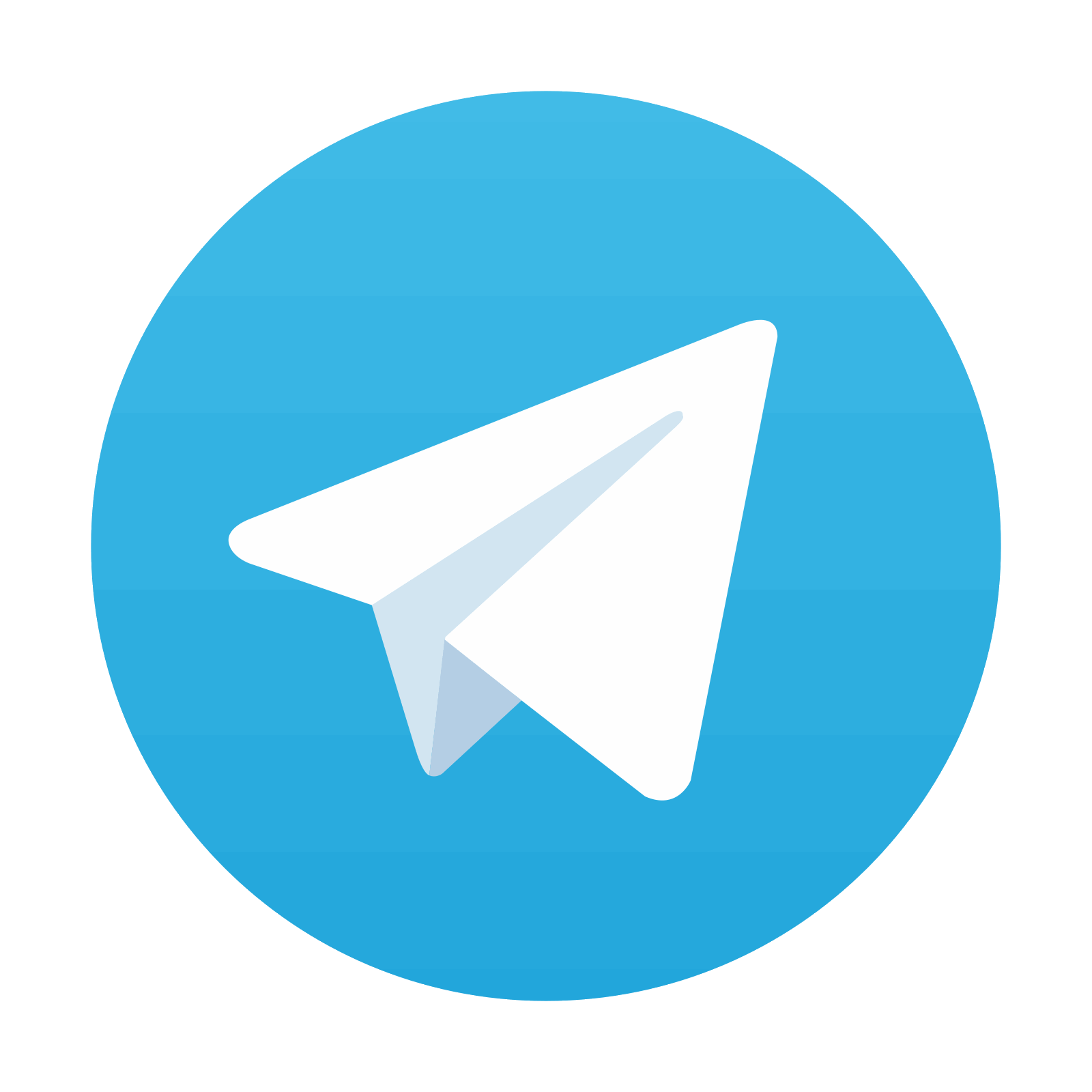
Stay updated, free articles. Join our Telegram channel

Full access? Get Clinical Tree
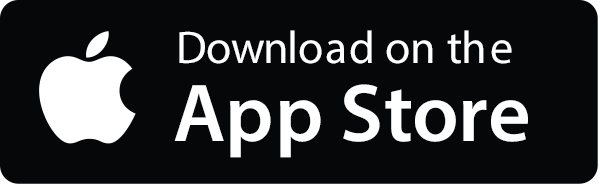
