Abstract
Purpose
To optimize the psychometric properties of age-related macular degeneration (AMD) quality of life (QoL) item banks (IBs), and evaluate their performance using computerized adaptive testing (CAT) simulations.
Design
Cross-sectional, clinical study.
Methods
261 AMD patients answered 219 items within seven IBs: Activity Limitation (AL); Lighting (LT); Mobility (MB); Emotional (EM); Concerns (CN); AMD Management (AM); and Work (WK), referred to collectively as “MacCAT”. The psychometric properties of each IB (e.g. measurement precision; item “fit”; differential item functioning (DIF)) were assessed using Rasch analysis. The mean number of items required for “high” and “moderate” measurement precision was determined using CAT simulations.
Results
Of the 261 participants (mean age 70.5 ± 7.6 years), 69 (26.4 %), 35 (13.4 %), 80 (30.7 %) and 77 (29.5 %) had no, early, intermediate and late AMD (better eye), respectively. AL, EM, CN and AM displayed good psychometric properties overall after collapsing response categories and deleting items for misfit and/or DIF. Despite similar reengineering efforts, LT and MB had suboptimal measurement precision but were retained due to otherwise good psychometric performances. Owing to unresolvable psychometric issues, WK was not considered further. Targeting for all IBs was suboptimal. In CAT simulations on the six remaining IBs, the mean number of items required per IB ranged from 8 (AL) to 13 (MB) for moderate, and 13 (AL) to19 (MB) for high measurement precision.
Conclusions
Six IBs demonstrated acceptable psychometric properties and potential CAT efficiency, suggesting MacCAT provides a comprehensive measurement of the QoL impact of AMD and associated treatments. Further testing in larger clinical cohorts is needed.
1
Introduction
Age-related macular degeneration (AMD) accounts for almost 10 % of blindness worldwide and can substantially affect quality of life (QoL), causing reduced visual functioning, mobility and emotional well-being. Anti–vascular endothelial growth factor agents administered via intravitreal injections for the treatment of neovascular AMD can improve visual outcomes with associated increases in QoL. However, anxiety over eye injections and the long-term nature of treatment may also negatively impact patients’ psychological well-being, leading to low adherence and compliance to therapy.
A comprehensive understanding of the impact of AMD and the effectiveness of treatment therapies on QoL outcomes is crucial, particularly in the context of the US Food and Drug Administration requirements for pharmaceutical companies to include patient-centered endpoints in clinical trials ; and value-based care models that require healthcare institutes to incorporate patient-reported outcomes into clinical care. QoL measurement is usually done using patient-reported outcome measures (PROMs). To date, there are only three PROMs specific to AMD, and these either have psychometric limitations or focus on specific subgroups of AMD (e.g. early/intermediate AMD or geographic atrophy ). They are also fixed-length, such that patients must be offered all items (questions) in the scale even if they are too hard or too easy, which can increase the test-taking burden.
Item banking and computerized adaptive testing (CAT) provide a promising solution to these issues. An item bank (IB) is a repository of items measuring a patient-reported construct (e.g. activity limitation) that have been calibrated on an interval-level scale using psychometric methods such as Rasch analysis. Items that are most informative at that particular point in the test are chosen by the CAT algorithm, and items continue to be offered until a stopping criterion (e.g. measurement precision, based on standard error of measurement [SE] or an item cap) is reached. Through this targeted process, CATs usually require fewer items than most fixed-length PROMs to obtain a precise score, offering an efficient means of estimating patients’ level of QoL.
While a QoL IB for AMD was earmarked for development as part of the Eye-tem Bank study in Australia, it is not yet available. We recently described the development of a 7-domain, 219-item AMD-specific QoL PROM (“MacCAT”) based on qualitative interviews in patients with AMD. Here, we aim to optimize the psychometric properties of the MacCAT IBs in a clinical sample of AMD patients and evaluate their performance using CAT simulations.
2
Methods
2.1
Sample population
Patients aged ≥ 55 years (English- or Mandarin-speaking) with a primary diagnosis of AMD in at least one eye were recruited from the Singapore National Eye Center (N = 114), National University Hospital (N = 111) and Tan Tock Seng Hospital (N = 36) between January 2021 and June 2023. Patients were excluded if they had other ocular comorbidities including severe glaucoma or diabetic retinopathy, clinically significant cataract, neurological conditions affecting vision (e.g. stroke), history of intraocular surgery for other retinal conditions (e.g. retinal detachment, endophthalmitis or glaucoma), and/or hearing or cognitive impairment (assessed using the six-item cognitive impairment test [6-CIT] questionnaire ). We utilized target quotas for ethnicity, gender, age and AMD severity to ensure our calibrations were applicable to a diverse sample.
The study protocol was approved by the SingHealth Centralized Institutional Review Board (CIRB #2018/2803) and written informed consent was obtained from participants. The study was conducted in accordance with the Declaration of Helsinki.
Assessment of AMD and visual acuity
Visual acuity (VA) data (both eyes) were extracted from patients’ files. Definitions of vision impairment (VI) are provided in Supplementary Digital Content (SDC) 1, Materials and Methods. AMD was graded from fundus images and classified according to the Beckman criteria into none, early, intermediate and late (neovascular, polypoidal choroidal vasculopathy or geographic atrophy). If the fundus image was unclear, severity grading was instead extracted from participant case notes.
2.2
Development of the AMD IBs
The development of domains and items for MacCAT has been described previously and more information is provided in SDC Table 1 . In summary, the final MacCAT instrument comprised 219 items under seven QoL domains: Activity Limitation ( AL ; n = 66); Lighting ( LT ; n = 19); Mobility ( MB ; n = 23); Emotional ( EM ; n = 34); Concerns ( CN ; n = 42); AMD Management ( AM ; n = 20); and Work ( WK ; n = 15). Items were rated on a 4–5 Likert-type scale with a non-appliable option available when indicated.
2.3
Psychometric evaluation of the IBs
Rasch analysis was conducted separately on each IB using Winsteps software (version 4.7.0.0; Chicago, IL, US) using the Andrich single rating scale model. Rasch analysis estimates the relative difficulty of items (item measures) and relative abilities of respondents (person measures) and links them on a common scale. This process transforms ordinal data into estimates of interval-level data, expressed in log of the odds units (logits). Rasch analysis also enables the psychometric properties of a scale to be evaluated and optimized, and subsequently enables threshold calibrations for each item (i.e. their “difficulty” level) to be calculated, which is an essential component of the CAT algorithm. Descriptions of the Rasch “fit statistics” are described in brief below, with more detailed information provided in SDC 1, Materials and Methods ). Detailed results are also summarized in SDC Table 2-8 . The research team, comprising content development experts and psychometricians (EF, RM, EL), and clinical experts (AT, GC) used the following analytic performance criteria to guide decision-making regarding retaining or dropping domains and items: for domains, criteria included key fit statistics (i.e. rating scale assessment, precision, unidimensionality), applicability, ceiling effects, test information function [TIF], item separation index [ISI], measurement range and clinical importance. For items, criteria included item fit, differential item functioning [DIF], applicability, discrimination, clinical importance and patient importance rating [ SDC 1, Materials and Methods ]).
2.3.1
Rating scale assessment
It is important to assess whether the participants are using the response categories (e.g. None, A little, Quite a bit, A lot, Unable to do) as expected (i.e. that there is a greater probability of patients with lower “ability” levels of the construct being measured selecting the more “difficult” response options for any given item). This is observed by a distinct peak for each response option on the category probability curve graphs ( SDC Figs. 9–15 ).
2.3.2
Precision
Measurement precision refers to the reproducibility of person measures and how sensitive the IBs are in distinguishing between patients with high and low levels of the construct under assessment (e.g. activity limitation). It is determined by person separation indices, namely person separation index (PSI) and person reliability (PR). PSI and PR cut-off values of > 2.0 and > 0.8, respectively, were used in this study. Extreme minimum or maximum scores (i.e. those reporting the worst or best response for all items, respectively) were removed from the analysis a priori (N ranging between 28 [Lighting] to 133 [Mobility]) as they are not informative for establishing item measures.
2.3.3
Unidimensionality
It is important that each IB measures a single latent construct (e.g. AL) as multidimensionality (i.e. measurement of multiple constructs) makes interpreting scores difficult. Principal components analysis of residuals was used to assess the unidimensionality of each IB. Possible multidimensionality was indicated if the eigenvalue of the first contrast was ≥ 3 and the raw variance explained by measures was < 50 %, and four other metrics were also considered if needed (see SDC 1, Materials and Methods for details).
2.3.4
Item fit statistics
“Item fit” indicates whether patients are responding to an item as expected based on the item’s difficulty and their own “ability” level, i.e. whether the item “fits” with the overall Rasch measurement model. Infit and outfit MnSq statistics determined item fit (acceptable range: 0.5–1.5). If the misfit was not resolved by this process, item deletion was considered.
Item discrimination was also considered when assessing item performance. Over-discriminating items (>1.0) tend to discriminate between high and low performers more than expected, while under-discriminating items (<1.0) tend to discriminate between high and low performers less than expected. As under-discrimination is a greater threat to measurement, we considered items with values substantially under 1.0 for deletion.
2.3.5
Local item dependency (LID)
LID refers to a situation where the response to one item is influenced by the response to another item. Ideally, there should be no correlation between the residuals of two items after the effect of the underlying construct is considered. LID between two items was deemed present in our IBs if the correlation of residuals was > 0.2.
2.3.6
Targeting
Targeting refers to how well the items in the IB target the ability level of the patients in the sample (i.e. are hard items available for patients with fewer visual issues and easier items for patients with a lot of visual issues?). Person-item maps were used to inspect scale targeting ( SDC Fig. 1-7 ). Two metrics were suggestive of poor targeting: 1) gaps in item coverage, as assessed visually using the person-item (Wright) map; and 2) a difference of > 1.0 logits between the mean item difficulty and person ability.
2.3.7
Differential item functioning (DIF)
DIF indicates if certain items operate differently for particular sample subgroups (e.g. men vs. women; or older vs. younger patients). If present, DIF indicates item bias for certain participant characteristics. A DIF contrast of > 1.0 logits with an associated Rasch-Welch probability of P < 0.05 suggested notable DIF. We examined uniform DIF for gender, age group (<70 vs. ≥70 years), binocular VI (none [≤0.3 logMAR] vs. yes [>0.3 logMAR]) and language (English vs. Mandarin).
2.3.8
Measurement range
A larger measurement range indicates the ability of the IB under assessment to measure a greater spectrum of the associated latent construct and was calculated as the difference in logits between the highest and lowest item locations (i.e. the “easiest” and “hardest” items).
2.3.9
Test information function (TIF)
The sum of information provided by all items in an IB bank is demonstrated by the TIF. A TIF of ≥ 10 is generally considered excellent. The TIF also identifies where the test has the highest/lowest standard error (SE). A higher level of test information indicates greater measurement precision (i.e. low SE) at that point on the scale.
2.3.10
Item separation index (ISI)
Item separation signifies construct validity. Low item separation (<3.00) suggests a low difficulty range of items and/or that the person sample is too small to confirm the item difficulty hierarchy.
2.3.11
Level of dependence between different IBs
Using the Pearson correlation coefficient on individual person measures from each IB, we determined the independence of each IB. A correlation coefficient of r < 0.8 provided supporting evidence that each IB was measuring an independent QoL construct.
2.4
CAT simulations
Once the psychometric properties of each item bank had been optimized using Rasch analysis, the item structure calibrations (i.e. the response category threshold information for each item) were exported from Winsteps software. Simulations were performed to assess the efficiency of our threshold calibrations (JMLE; Joint Maximum Likelihood Estimation method) and associated CAT algorithm in 1000 simulated respondents using R Statistical Computing Environment (“catR” package ). Simulations were based on a standard normal distribution (M = 0, SD = 1) and used the Rating Scale Model (RSM), the ML (maximum likelihood) estimator and the Maximum Fisher Information item selection criteria. The average number of items required was determined for two different stopping rules: SE of 0.30 “high precision” (reliability approximation 0.91) and SE of 0.387 “moderate precision” (reliability approximation 0.85). We assessed model fit using the root mean square error (RSME) and level of bias between true and estimated ability levels (low values are desirable). Using Pearson correlation coefficient, we assessed correlations between simulated person measure estimates from the IBs and CAT and hypothesized high ( r ≥ 0.85) and moderate-high (0.75 ≥ r < 0.85) correlations for the high and moderate precision stopping rules, respectively. Findings are summarized overall ( Table 3 ) and per decile ( SDC Tables 9–14 ) . Deciles D1-D10 included 100 simulated respondents each, and D1 and D10 represented simulated respondents at the lowest and highest “ability” level, respectively, across the latent trait.
3
Results
3.1
Sociodemographic and clinical characteristics
Of the 261 participants (mean age 70.5 ± 7.6 years), 69 (26.4 %), 35 (13.4 %), 80 (30.7 %) and 77 (29.5 %) had no, early, intermediate and late AMD in the better eye, respectively ( Table 1 ). Patients had received a range of treatments (e.g. right eye: None [n = 55, 21.1 %]; Supplements [n = 37, 14.2 %]; Laser [n = 3, 1.2 %]; Intravitreal injection [n = 79, 30.3 %]; and Combination [n = 51, 19.5 %]). Most patients had no binocular VI (n = 204, 79.1 %), while 7.8 % (n = 20) and 13.2 % (n = 34) had mild and moderate/severe VI, respectively.
Variable | N | % * |
---|---|---|
Gender | ||
Male | 155 | 59.4 % |
Ethnicity | ||
Chinese | 235 | 90.0 % |
Malay | 16 | 6.1 % |
Indian | 10 | 3.8 % |
Marital status | ||
Single | 19 | 7.3 % |
Married | 221 | 84.7 % |
Divorced/separated/widowed | 21 | 8.0 % |
Low SES †‡ | ||
Yes | 14 | 5.4 % |
No | 177 | 92.7 % |
Employment status | ||
Working | 100 | 38.3 % |
Not working | 161 | 61.7 % |
Chronic health conditions † | ||
Hypertension | 153 | 58.6 % |
Dyslipidaemia | 142 | 54.4 % |
Diabetes | 59 | 22.6 % |
Heart attack | 17 | 6.5 % |
Stroke | 7 | 2.7 % |
AMD type | ||
Geographic atrophy | 16 | 6.1 % |
AMD Severity (better eye) | ||
None | 69 | 26.4 % |
Early | 35 | 13.4 % |
Intermediate | 80 | 30.7 % |
Late | 77 | 29.5 % |
AMD Severity (worse eye) | ||
Early | 16 | 6.1 % |
Intermediate | 56 | 21.5 % |
Late | 189 | 72.4 % |
AMD treatments (by eye; N = 522) | ||
No AMD | 70 | 13.4 % |
None | 124 | 23.87 % |
Supplement | 64 | 12.3 % |
Laser | 10 | 1.9 % |
Intravitreal injection | 159 | 30.5 % |
Combination * | 95 | 18.2 % |
Vision impairment (binocular) | ||
None (≤0.3 logMAR or ≤20/40 Snellen) | 204 | 79.1 % |
Mild (>0.3 logMAR ≤0.48 or >20/40 Snellen ≤20/60) | 20 | 7.8 % |
Moderate/severe (>0.48 logMAR or >20/60 Snellen) | 34 | 13.2 % |
Vision impairment (worse eye) | ||
None (≤0.3 logMAR or ≤20/40 Snellen) | 110 | 42.6 % |
Mild (>0.3 logMAR ≤0.48 or >20/40 Snellen ≤20/60) | 41 | 15.9 % |
Moderate/severe (>0.48 logMAR or >20/60 Snellen) | 107 | 41.5 % |
Use of assistive devices/low vision aids | ||
None | 36 | 13.8 % |
Corrective aids (glasses) | 218 | 83.5 % |
Magnifiers (handheld, lenses, electronic) | 32 | 12.3 % |
Adjusted lighting | 1 | 0.4 % |
Continuous variables | Mean/Median | SD/IQR |
Age (years) | 70.5/70 | 7.6/64–76 |
Presenting VA (binocular), logMAR; Snellen | 0.20; 20/32 / 0.14; 20/28 | 0.23; 20/34 / 0.04–0.30; 20/22–20/40 |
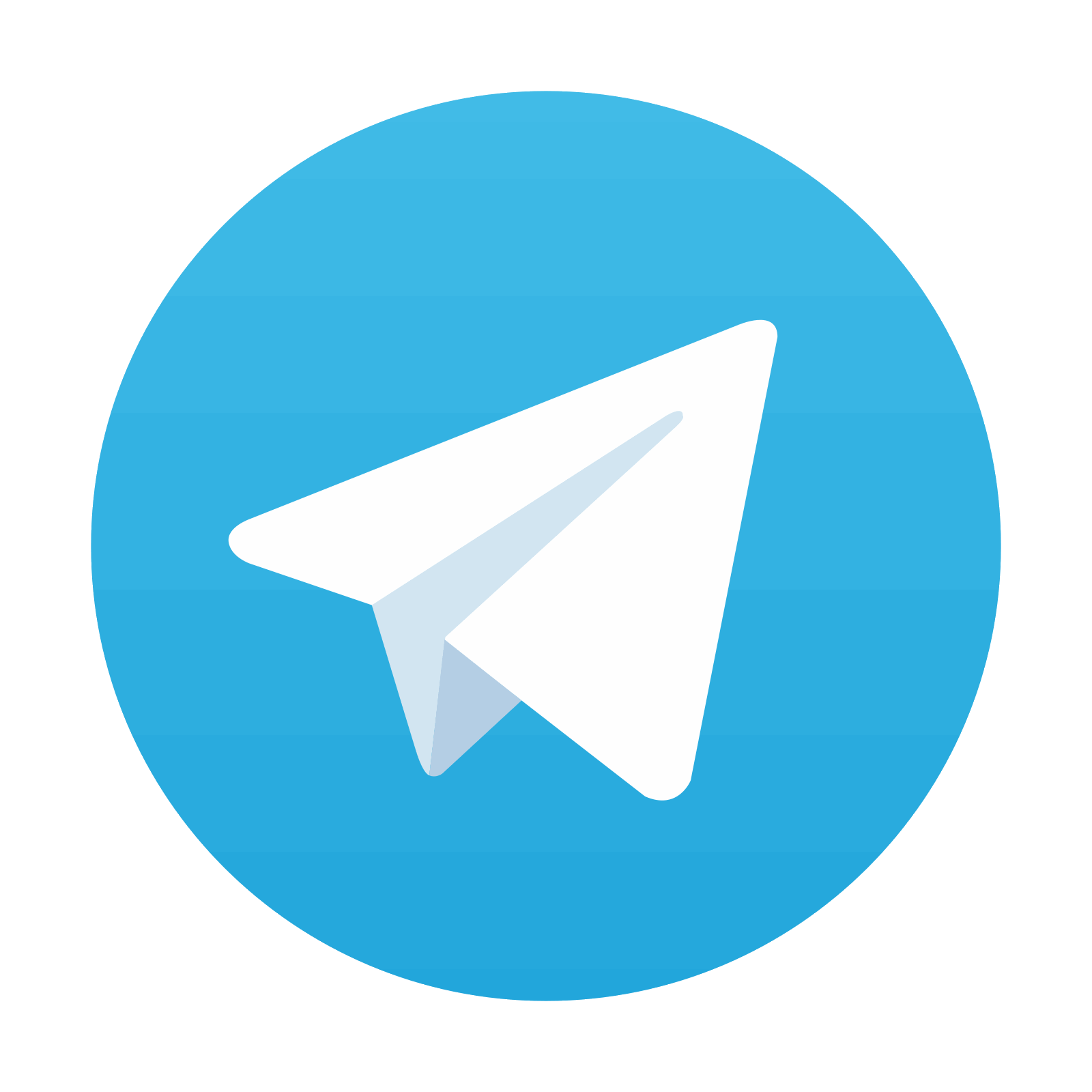
Stay updated, free articles. Join our Telegram channel

Full access? Get Clinical Tree
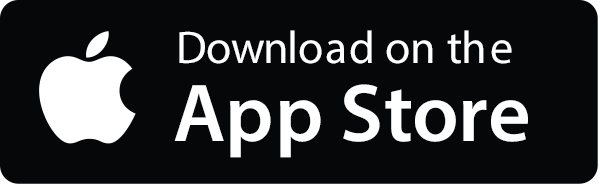

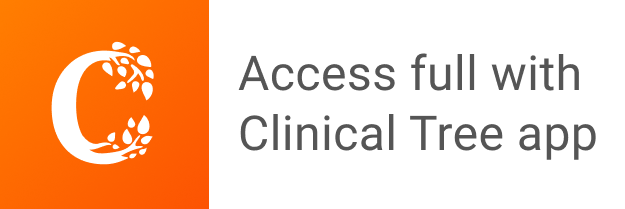