75 Medical Statistics • Categorical data—nominal (sex), grade of tumour (ordinal) • Quantitative data—measured or counted, e.g., age, blood pressure • Measure of variation—interquartile range and median • Histograms—display grouped frequency (distribution of a continuous variable)—should generally have 5 to 15 groups • Bar charts—distribution of a discrete variable or a categorical one (spaces between bars) • Mean will be affected by outlying data, median will not • Standard deviation (SD) gives an indication of the spread about the mean—relies on the data being symmetrically distributed • If normal distribution occurs: • SD in ungrouped data uses degrees of freedom = division by total number of observations minus 1 • Skewed data are often best presented via a log transformation • Measurement error—SD for repeated measurements • Coefficient of variation = intrasubject SD/mean expressed as a percentage • Absolute risk reduction (ARR) = difference between 2 risks for 2 treatments (%age) • If new therapy beneficial = number needed to treat—ARR will be +ve (1/(P1 − P2)) • Risk ratio or relative risk (RR)—if <1 = lower risk in control group • RR reduction = (control risk—experimental risk)/control risk • Odds (event) = probability of event happening (P)/(1 − P) • Odds ratio (OR) = odds of event 1/odds of event 2 • Use of median or mean does not depend necessarily on distribution of data; if there is a small group at one extreme of the distribution then the median will be more useful, otherwise the mean is generally preferred • Data not normally distributed may well derive useful information from both median and mean • SD is only interpretable for variables that have approximately symmetrical distribution • SD should not be used for data that are not plausibly normal e.g., age—interquartile range (IQR) better • Case-control studies—quote OR • Cross-sectional studies—either OR or RR • Standard error (SE) used to study significance of difference between 2 means = SD/n; measure of precision of a population parameter • Random sampling allows a population to be studied more conveniently—may be stratified to allow for age/sex distribution • Unbiased measurement = average of a large set will be close to the true value • Precise measurement = repeatable • Non-random samples, e.g., hospital patients vs. community, volunteers vs. non; reduce biases by providing demographic data • Acceptable response rate from a survey = 65 to 70%; useful to present data on nonresponders; smaller responses valid if no biases • Sample SD = estimate of population parameter (variability of observations) • SE of an estimate will decrease with increasing sample size • SD is used to describe data, i.e., normal distribution • SE is used to describe the outcome of a study, e.g., estimate the prevalence of disease • 95% limits = reference range = mean ± 1.96 SD (~ 2 SD) • p-value = probability of getting the observed value (or more extreme) if the null hypothesis were correct (e.g., p < 0.05) • 95% CI = mean ± 1.96 SE (~ 2 SE)—this indicates that only 5% chance that this range excludes the mean • Reference range refers to individuals; confidence interval (CI) refers to estimates • Null hypothesis = no difference between populations compared • Type I error = rejection of null hypothesis when in fact it is true—using mean ± 1.96 SE = 1/20 chance of being wrong • A non-significant difference does not make the null hypothesis likely; this is just absence of evidence • If CI excludes 0, the chance of samples being from same population is less than 5%
75.1 Data Display and Summary
75.2 Summary Statistics for Quantitative and Binary Data
Mean ± 1 SD = 68% of data
Mean ± 2 SD = 95% of data
Mean ± 3 SD = 99.7% of data
75.3 Populations and Samples
75.4 Statements of Probability and Confidence Intervals
75.5 Differences between Means: Type I and Type II Errors and Power
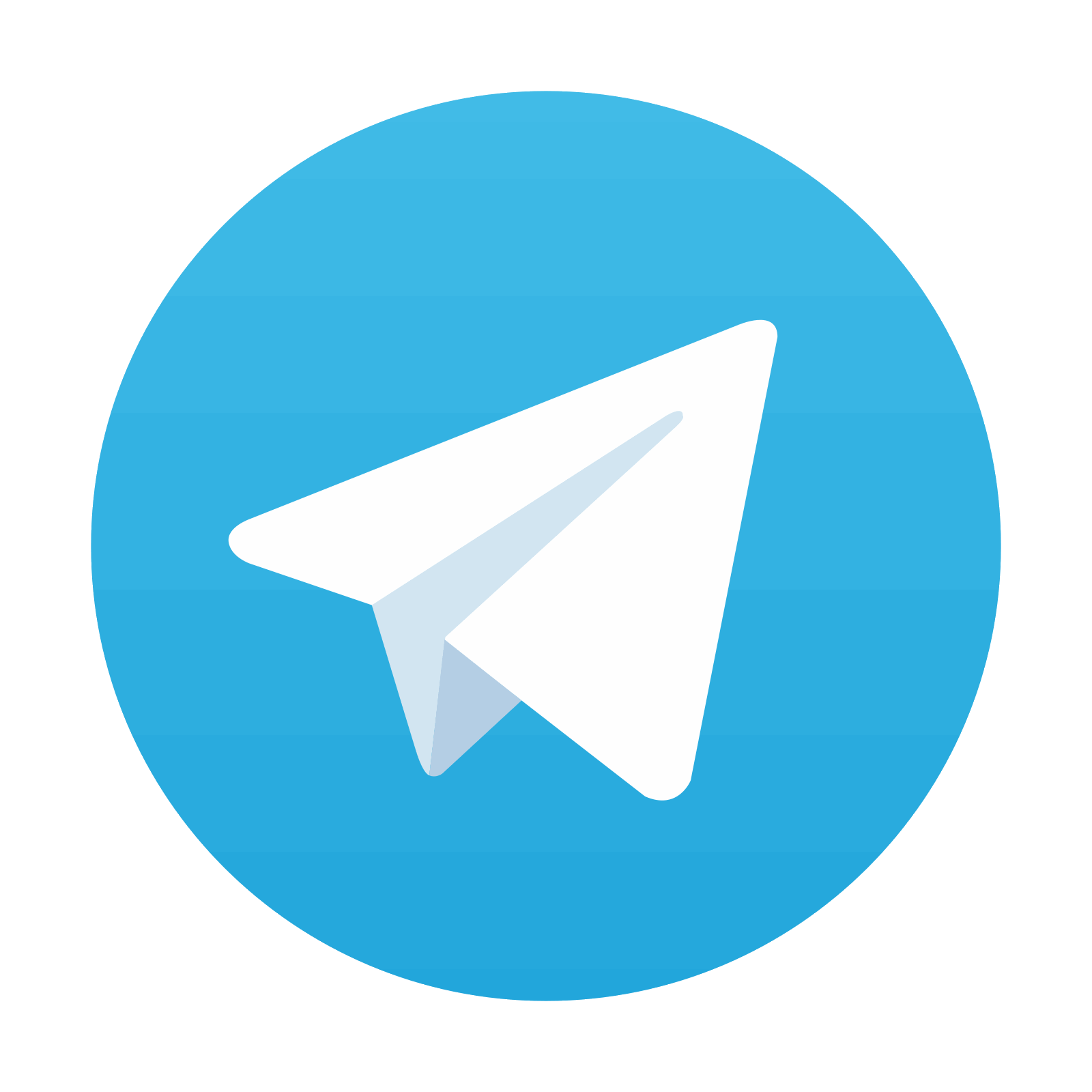
Stay updated, free articles. Join our Telegram channel

Full access? Get Clinical Tree
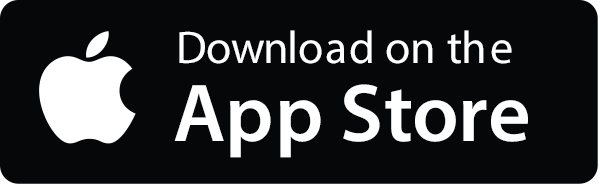
