Purpose
To evaluate whether a combination of topographic optic disc measurements and visual field data may improve the estimation of rates of structural and functional progression in glaucoma and the prediction of future outcomes in the disease.
Design
Observational cohort study.
Methods
The study included 242 eyes of 179 glaucoma patients followed for an average of 6.4 ± 1.1 years. Subjects were longitudinally monitored with standard automated perimetry (SAP) and confocal scanning laser ophthalmoscopy (CSLO). Slopes of functional and structural change over time were evaluated by the parameters mean deviation (MD) and rim area, respectively. For each eye, the follow-up time was divided into 2 equal periods: the first half was used to obtain the slopes of change and the second period was used to test the predictions. Slopes of change were calculated using 2 methods, the conventional approach of ordinary least squares linear regression and a Bayesian joint regression model integrating structural and functional information. The mean square error (MSE) of the predictions was used to compare the predictive performance of the different methods.
Results
Bayesian slopes were more accurate than those obtained by the ordinary least squares method in predicting future MD (MSE: 5.13 vs 11.2, respectively; P < .001) and rim area values (MSE: 0.016 vs 0.027, respectively; P < .01).
Conclusion
A Bayesian joint regression model combining structure and function resulted in more accurate and precise estimates of slopes of change compared to the conventional method of ordinary least squares linear regression.
Detection of progression is paramount to the diagnosis and management of glaucoma; however, although most glaucoma patients will show some evidence of change if followed long enough, the rate of deterioration can be highly variable among them. While most patients progress relatively slowly, some have aggressive disease with rapid deterioration that necessitates more aggressive therapeutic intervention. According to the Consensus on Medical Treatment from the World Glaucoma Association, glaucoma treatment is generally indicated for patients whose rates of progression will most likely result in loss of vision-related quality of life over the projected remaining years of life. Therefore, accurate and precise estimation of rates of change are essential in order to determine the need and/or aggressiveness of treatment.
Standard automated perimetry (SAP) remains the most common method for estimating rates of functional progression. However, there is evidence that many patients can present structural changes in the optic nerve or retinal nerve fiber layer (RNFL) before detectable changes in SAP. There is also evidence that progressive changes to the optic nerve are highly predictive of future functional deterioration and, therefore, monitoring rates of structural damage becomes essential to improve individualized management of the disease. On the other hand, several patients show evidence of functional deterioration without measurable changes in currently available structural tests, highlighting the need for assessing rates of both structural and functional change throughout the course of the disease.
The disagreement between structural and functional methods for detecting progression could be related to the different algorithms employed to assess change, to the variability of measurements over time, or to the different scales used to assess structure and function. While some disagreement is to be expected, we have recently shown that a combination of structural and functional tests can significantly improve detection of glaucoma progression. Using Bayesian statistics, we developed a joint model that takes into account the correlation between the different tests in order to integrate the longitudinal information obtained from structural and functional evaluations, allowing the information derived from one test to influence the inferences obtained from the other test. For example, a visual field change that would otherwise be declared nonstatistically significant by analysis of visual field data alone may be declared significant after taking into consideration the structural changes occurring in the same eye, and vice versa. We applied our method to combine SAP and scanning laser polarimetry RNFL measurements and we demonstrated that the method significantly improved detection of glaucoma progression compared to either test used alone or to the use of both tests, but without taking into account the correlation between them.
The main purpose of calculating rates of change is to predict future outcomes for an individual patient. That is, it is assumed that current rates of disease progression will remain relatively unchanged unless further interventions take place and, therefore, are good predictors of future observations. In the current study, we expand our Bayesian methodology to a combination of SAP and topographic optic disc measurements to demonstrate that it can also improve the estimation of rates of structural and functional damage in the disease and prediction of future outcomes in a cohort of glaucoma patients followed over time.
Methods
This was an observational cohort study. Participants from this study were included in 2 prospective longitudinal studies designed to evaluate optic nerve structure and visual function in glaucoma (the African Descent and Glaucoma Evaluation Study [ADAGES] and the Diagnostic Innovations in Glaucoma Study [DIGS]). The 3-site ADAGES collaboration includes the Hamilton Glaucoma Center at the Department of Ophthalmology, University of California, San Diego (UCSD) (data coordinating center); the New York Eye and Ear Infirmary; and the Department of Ophthalmology, University of Alabama at Birmingham (UAB). Although the DIGS includes only patients recruited at UCSD, the protocols of the 2 studies are identical. Methodological details have been described previously.
At each visit during follow-up, subjects underwent a comprehensive ophthalmologic examination including review of medical history, best-corrected visual acuity, slit-lamp biomicroscopy, intraocular pressure (IOP) measurement, gonioscopy, dilated funduscopic examination, stereoscopic optic disc photography, and automated perimetry using Swedish interactive threshold algorithm (SITA Standard 24-2). Only subjects with open angles on gonioscopy were included. Subjects were excluded if they presented with a best-corrected visual acuity less than 20/40, spherical refraction outside ±5.0 diopters and/or cylinder correction outside 3.0 diopters, or any other ocular or systemic disease that could affect the optic nerve or the visual field.
The study included 242 eyes of 179 patients diagnosed with glaucoma, as determined on the baseline visit. Seventy-five patients were recruited from the University of California, San Diego, 45 from the University of Alabama, and 59 from the New York Eye and Ear Infirmary. Eyes were classified as glaucomatous if they had repeatable (2 consecutive) abnormal visual field test results on the baseline visits and a glaucomatous-appearing optic disc based on masked stereophotograph assessment. An abnormal visual field was defined as a pattern standard deviation (PSD) outside of the 95% normal confidence limits, or a glaucoma hemifield test result outside normal limits.
Standard Automated Perimetry
All visual fields were evaluated by the UCSD Visual Field Assessment Center (VisFACT). Visual fields with more than 33% fixation losses or false-negative errors, or more than 15% false-positive errors, were excluded. The only exception was the inclusion of visual fields with false-negative errors of more than 33% when the field showed advanced disease. Visual fields exhibiting a learning effect were also excluded. Visual fields were further reviewed for the following artifacts: lid and rim artifacts, fatigue effects, inappropriate fixation, evidence that the visual field results were attributable to a disease other than glaucoma (such as homonymous hemianopia), and inattention. The VisFACT requested repeats of unreliable visual field test results, and these were obtained whenever possible.
Evaluation of rates of visual field change during follow-up was performed using the mean deviation (MD) global index provided by the Humphrey perimeter (Carl-Zeiss Meditec Inc, Dublin, California, USA).
Confocal Scanning Laser Ophthalmoscopy
Topographic optic disc measurements were obtained using confocal scanning laser ophthalmoscopy (CSLO) with the HRT II (software version 3.0, Heidelberg Engineering, Dossenheim, Germany). It uses confocal scanning laser principles to obtain a 3-dimensional topographic image of the optic nerve. Its principles of working have been described in detail elsewhere. For each patient, 3 topographic images were obtained and were combined and automatically aligned to make a single mean topography used for analysis. An experienced examiner outlined the optic disc margin on the mean topographic image while viewing stereoscopic photographs of the optic disc. Good-quality images required a focused reflectance image with a standard deviation not greater than 50 μm. Corneal curvature measurements were used to correct images for magnification error. The CSLO Reading Center at the University of California, San Diego conducted all quality assessment and image processing and certified all operators according to standard protocol. The parameter rim area was used to evaluate rates of structural change over time.
Data Analysis
A minimum of 4 HRT examinations and 4 SAP tests during follow-up were required. Average follow-up time was 6.4 ± 1.1 years (range: 5.0 to 13.1 years). For each eye, the follow-up time was divided into 2 equal periods: the first half was used to obtain the slopes of change and the second period was used to test the predictions. That is, rates of change were obtained using only the tests available during the first half of follow-up. Subsequently, these rates of change were then used to predict observations for individual eyes during the second half of the follow-up period. Slopes of change were calculated using 2 methods, the conventional approach of ordinary least squares linear regression and a Bayesian joint regression model. Using ordinary least squares regression, 2 slopes were obtained for each eye, 1 representing rim area loss over time and another representing MD change over time. Ordinary least squares regression is the standard approach used to calculate slopes of change in commercially available software packages from perimeters and imaging devices and does not take into account the correlation between the results of the 2 tests. The Bayesian model also provides 2 slopes for each eye, 1 for rim area and 1 for MD; however, the calculation of the slope for MD loss over time takes into account the slope of rim area change and vice versa, as described below.
For each measurement (rim area and MD) and method (ordinary least squares vs Bayesian), the residual difference, or error, between the actual value and the predicted value was calculated for each observation available during the second half of the follow-up period. The mean square error (MSE) of the predictions was used to evaluate and compare the predictive performance of the different methods.
Bayesian Joint Regression Model
A joint regression model was implemented using a Bayesian hierarchical modeling framework to integrate the 2 longitudinal measures obtained over time, that is, the HRT rim area and SAP MD. Details of the modeling approach have been described in a previous publication. In brief, random-intercept random-slope hierarchical models were used to evaluate the evolution of each response over time. In these models, the average evolution of a specific response is described using some function of time, and subject-specific deviations from this average evolution are introduced by random intercepts and random slopes, allowing for different baseline values and different rates of change for each eye. Hierarchical models can also easily handle the correlation structure arising from data coming from both eyes of the same patient. In a joint-modeling approach using hierarchical models, random effects are assumed for each response process and the different processes are associated by imposing a joint multivariate distribution on the random effects. This approach has great flexibility and allows joining models for responses of different types and also with different numbers of observations, as in our application.
At the first level, each response process is described using a linear model:
Y 1i = α 1j[i] + β 2j[i] × time i + ε 1i , ε 1i ∼ N ( 0 , σ 1 y 2 ) for i = 1 , 2 , 3 , 4 , … , n
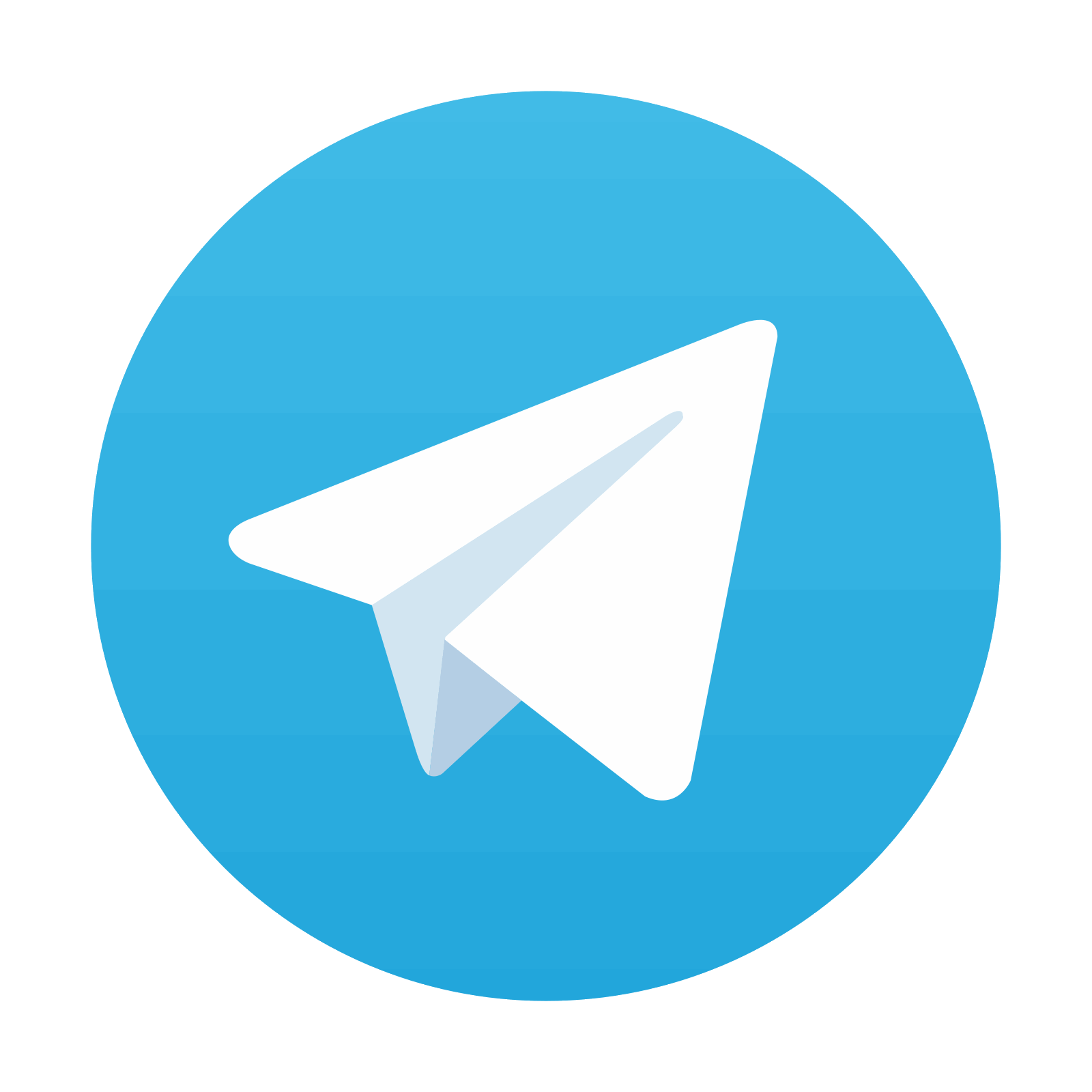
Stay updated, free articles. Join our Telegram channel

Full access? Get Clinical Tree
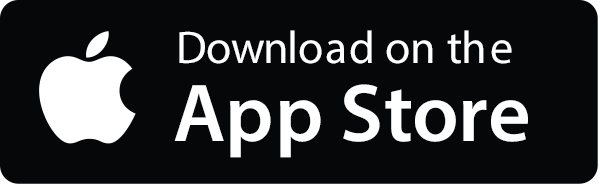
