A clear understanding of linear regression analysis is of fundamental importance to quantitative research. In this editorial, I briefly discuss some of the key concepts; a comprehensive treatment is available in many textbooks, such as that by Kutner and associates. Linear regression is used to describe the relationship of a continuous outcome measure to 1 or more explanatory or predictor measures. Consider a hypothetical research study on patients with an ophthalmologic disease comparing visual acuity outcomes (measured by logarithm of the minimal angle of resolution [logMAR]) for a novel therapy versus standard care. The simplest analysis of the data from this study is to use a 2-sample t test: doing so shows that the 100 therapy subjects (0.119 ± 0.162, mean ± standard deviation) had better visual acuity outcomes (lower mean logMAR) than the 100 subjects in usual care (0.179 ± 0.167), and that the difference was statistically significant (difference in means ± standard error of −0.060 ± 0.023, P = .010 from 2-sample t test).
Equivalently, a linear regression can be used to do this analysis. The individual values of logMAR (Y) are regressed on an indicator for group membership (X = 1 for subjects in novel therapy and X = 0 for those in usual care) to get the best fit linear regression model
where b 0 is the constant or intercept for the regression line (ie, the mean of Y when X is equal to 0) and b 1 is the average increment in Y associated with a 1-unit increase in X. The results of the linear regression are shown in Table 1 .
Parameter | Estimate (SE) | P Value |
---|---|---|
b0 (intercept) | 0.179 (0.016) | <.001 |
b1 (treatment a ) | −0.060 (0.023) | .010 |
To interpret these results in the context of the visual acuity study, the mean of logMAR for usual care was 0.179 (b 0 ) and novel therapy subjects had logMAR values that averaged 0.060 less (b 1 = −0.060) than those for usual care subjects ( P = .010). Inference (ie, the standard error of the difference and the associated P value) for this parameter is exactly the same as that produced by the 2-sample t test.
The linear regression presentation of the simple analysis does not add information to the t test results, so why go to the extra trouble? The answer is that other factors (eg, age and duration of disease) are likely to be associated with visual acuity; if these factors are not evenly distributed between the treatment groups then differences in these factors may be driving the apparent difference between the treatments. Indeed, for the example data, on average the novel therapy subjects were younger and had shorter disease durations than the usual care subjects. Linear regression can be used to obtain an estimate for the treatment group difference after adjusting for age and disease duration. The logMAR values are now regressed on the group indicator (X = 1 for novel therapy and X = 0 for usual care), age (subject age at time of study, in years), and DxDur (disease duration in years). The model is
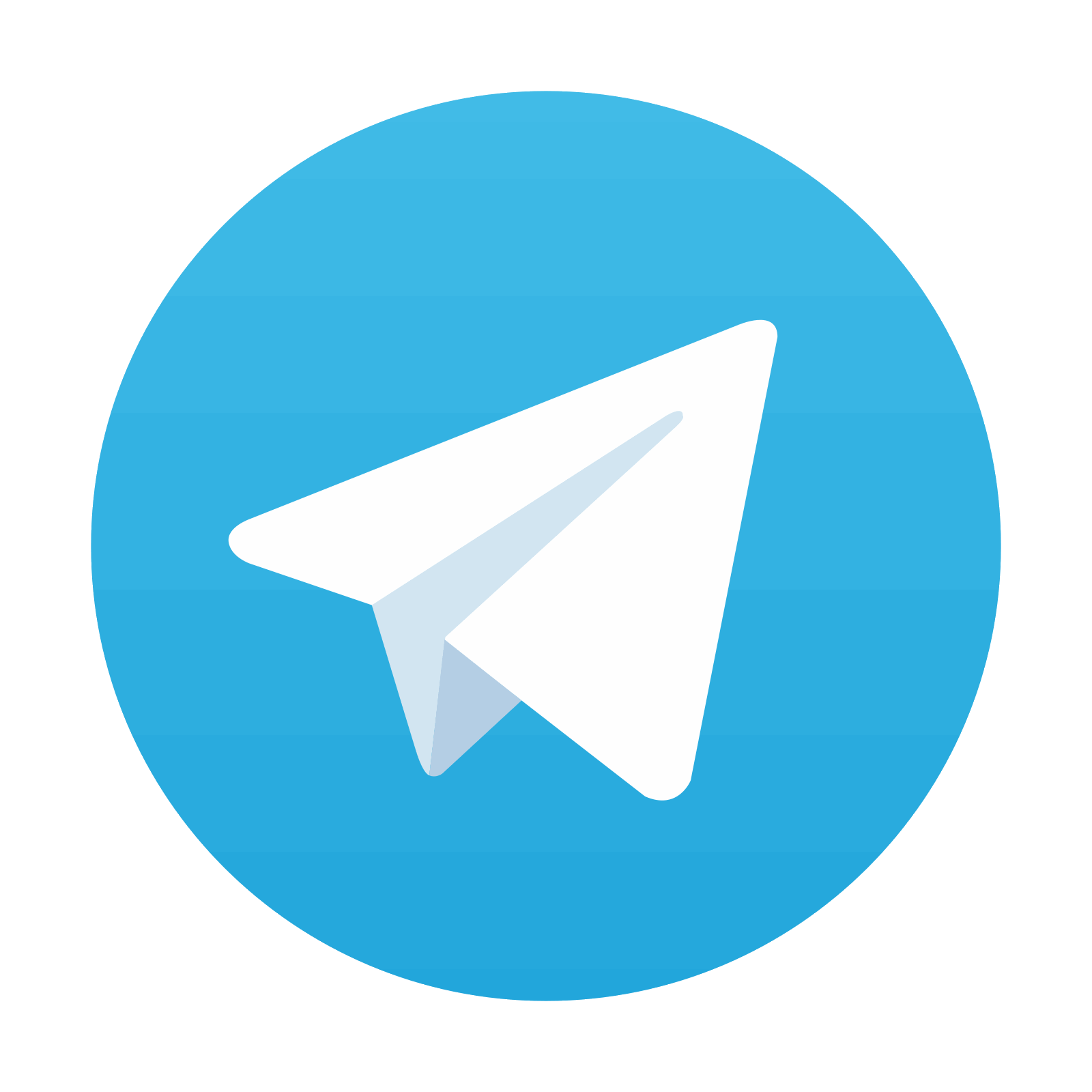
Stay updated, free articles. Join our Telegram channel

Full access? Get Clinical Tree
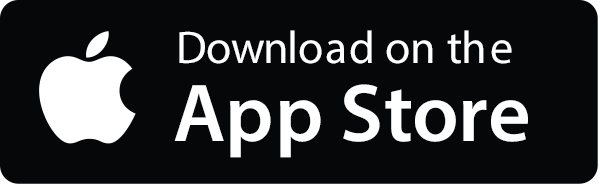
