Purpose
To determine whether glaucoma subspecialty training, formal risk estimation, or patient age has an impact on physician treatment recommendations in cases of ocular hypertension.
Design
Experimental study.
Methods
Members of the American Academy of Ophthalmology (118) and American Glaucoma Society (58) were recruited. Each physician was first asked how many young and old patients with ocular hypertension he or she would treat to prevent someone from progressing to glaucoma (number needed to treat). The physicians then reviewed 100 simulated cases of patients with ocular hypertension and reported their likelihood to treat each case. Half of these cases were presented with an estimated risk of conversion to glaucoma within 5 years and half were presented without an estimate. The treatment recommendations were analyzed to determine whether subspecialty status or the presence of a risk calculation had any impact on treatment recommendations.
Results
Both glaucoma specialists and non-glaucoma specialists were more likely to recommend treatment in cases for which a risk calculation was provided ( P = .001). Furthermore, non-glaucoma specialists were more likely to recommend treatment for ocular hypertensive patients than were glaucoma specialists ( P < .001). Finally, both groups indicated they were more likely to treat young patients than old.
Conclusions
Both provision of a risk estimate and lack of glaucoma subspecialty training were associated with physicians being more likely to treat ocular hypertension. These findings have implications with regard to ways in which the treatment of ocular hypertensive patients could be modified and possibly made more consistent with available evidence.
Estimation of a particular patient’s risk of disease is an essential aspect of rational clinical decision making. Only by comparing this risk to some threshold can a clinician reliably decide whether treatment is warranted for a particular patient. Although these estimates have historically been made by clinicians using some combination of experience and data, there is evidence that estimates made in this way do not coincide with formally calculated risk. This inability of clinicians to estimate risk has been demonstrated specifically in the case of conversion from ocular hypertension to glaucoma. While clinical decisions can arguably not be reduced to formulaic solutions, it is logical that there should be some correspondence between validated risk measurement and therapy choice.
One approach to estimating patient risk relies on longitudinal clinical studies to identify the relative contribution of specific risk factors to a particular diagnosis or outcome. The Framingham Heart Study was the first to use data in this way and a number of risk calculators have subsequently been developed to estimate the risk of cardiovascular disease. Similar calculators have been developed for estimating the 5-year risk of developing glaucoma in patients who are similar to the pre-glaucoma suspects who were participants in the Ocular Hypertension Treatment Study (OHTS). While the Framingham and OHTS calculators are derived from large patient groups and excellent study designs, it appears that the use of these data by clinicians has met resistance. For example, the impact of the Framingham risk calculator on clinical practice has apparently been modest. This may be more a matter of how easily information is presented to the clinician who is making the decision. When presented with actual risk estimates in constructed patient scenarios, we showed that glaucoma subspecialists altered their therapeutic decisions for glaucoma suspects.
The ability of physicians to estimate clinical risk and to incorporate it into their decisions is a complex task that is based on a number of factors, some of which are intrinsic to the physician and some of which may depend upon characteristics of the individual patient. To characterize the impact of plausible factors on the behavior of ophthalmologists, we used simulated clinical scenarios to analyze the effect of patient age and risk estimates on physician behavior and to compare the behavior of ophthalmologists without glaucoma fellowship training to glaucoma subspecialists.
Methods
Subject Recruitment
The methods used to recruit members of the American Glaucoma Society (AGS) to participate in the study have been described. In brief, we recruited physicians via an e-mail to AGS members asking them to participate in a study of the management of ocular hypertension. We subsequently recruited members of the American Academy of Ophthalmology (AAO) by sending letters to 1000 randomly selected mailing addresses taken from its membership list, after obtaining approval from the AAO. The letter also asked the recipient to participate in a study of how ophthalmologists manage ocular hypertension and offered them US$150 for their time. Enrollment was limited to those who were practicing ophthalmologists and who managed patients with ocular hypertension or glaucoma. Physicians from both the AGS and AAO who met the criteria and were interested were directed to a website.
Case Simulation
The details of generating the simulated case scenarios used in this project have also been described. Briefly, we used the results of the OHTS to generate for each simulated glaucoma suspect values for the 6 OHTS-identified risk factors for developing glaucoma: age, intraocular pressure (IOP), cup-to-disc ratio (CDR), pattern standard deviation (PSD) from automated field testing, central corneal thickness (CCT), and self-reported diabetes mellitus. These values were generated for each patient in 2 groups of simulated case scenarios. In the first group of cases, physicians were presented only with the demographic and clinical findings, while in the second group they also were given the calculated risk of developing glaucoma within 5 years. This risk was estimated using the formula described and validated by Medeiros and associates . We have previously shown that the distributions of estimated glaucoma risk in the 2 groups of simulated cases were identical and were similar to the group of ocular hypertensive subjects in the Diagnostic Innovations in Glaucoma Study. Thus, the only difference between the 2 groups of cases was knowledge of the estimated risk by the ophthalmologists.
Data Collection
The risk factors from the 2 groups of simulated cases were placed in a web-based survey tool to facilitate review by the participating ophthalmologists. Participants were instructed to review each patient scenario including values for all 6 OHTS risk factors as well as patient sex, which was included to better approximate real clinical decision making. For each simulated patient, the physicians were asked how likely they would be to recommend treatment to prevent glaucoma. Participants were explicitly instructed that this recommendation represented their initial position in the discussion with the patient. Responses were recorded along a 7-point scale that included “Definitely No” (no treatment), “Probably No,” “Possibly No,” “Unsure,” “Possibly Yes,” “Probably Yes,” and “Definitely Yes.”
We first asked physicians whether they routinely use IOP as the sole criterion for choosing treatment in ocular hypertension. Those who responded positively to this question would not provide useful information for this study and were excluded. Physicians who denied using only IOP when making treatment decisions were then asked to review 50 case scenarios without a calculated risk value and 50 cases with the risk value given. The order of the cases in 2 groups of patients was randomized within each group to minimize any effect of presentation order.
Participants were also asked to provide their sex, racial background, number of years in practice, subspecialty training, monthly clinic volume, fraction of practice devoted to glaucoma, and whether they now use a formal risk calculator in their practice. We wished to compare the actual treatment choices from the case scenarios with what physicians reported as their approach to treatment of ocular hypertension. To estimate the latter, we asked each participant for the number of ocular hypertensive patients they would be willing to treat to prevent 1 of them from developing glaucoma within 5 years. This value represents each physician’s personal number needed to treat (NNT). The NNT question was asked twice, once specifying patients between 40 and 50 years old and once for patients 70 to 80 years old.
Statistical Analysis
The demographics of glaucoma-trained and non-glaucoma-trained participants were compared using the Fisher exact test for categorical variables (sex, race, use of risk calculator) and the t test for numeric variables.
The first step in analyzing the treatment recommendations was to reduce the 7-point treatment recommendation scale to 3 levels. “Definitely No” and “Probably No” were combined as “No.” “Possibly No,” “Unsure,” and “Possibly Yes” were combined as “Unsure,” while “Probably Yes” and “Definitely Yes” were labeled “Yes.” The particular mapping was planned prior to data collection to move responses from the 7-point scale to the nearest value in the 3-point scale while still explicitly maintaining the concept of “Unsure.” The choice of a 3-point scale was made to facilitate interpretation of the subsequent regression analysis and to ensure an adequate number of responses in each category. Furthermore, there is evidence that 3-point scales are adequate, this approach is suggested for Likert-scale data in order to make subsequent analysis more understandable, and a similar approach was used in a recent study of treatment recommendations in ocular hypertension.
We calculated 2 statistics to compare the recommendations made by the 2 groups of physicians (with and without glaucoma subspecialty training). First, we estimated what we have termed the confidence of the physician in his or her recommendation and whether it was altered by the knowledge of the formal risk estimate. Each physician’s confidence was calculated as the proportion of all of his or her recommendations that were either Yes or No (ie, those that were not Unsure). Second, we created a measure called inconsistency, which is highest for a physician who is equally likely to assign a patient with a given risk to any of the 3 treatment categories (Yes, Unsure, No). Similarly, inconsistency is lowest (best) for a physician who makes recommendations that are well correlated with the risk of glaucoma. We operationalized this measure for each physician as the sum of the variances of the calculated risk in each of their 3 treatment recommendation groups (No, Unsure, Yes). Like confidence, inconsistency is a measure of each participating physician. To test whether confidence and inconsistency were related to the availability of a risk calculator or to subspecialty training (glaucoma or other), we used analysis of variance (ANOVA).
The values for NNT reported by each physician for patients aged 40 to 50 and 70 to 80 years were used to calculate the average risk of glaucoma each physician would be willing to treat. The value for average risk can be calculated by starting with the formula for NNT:
where CER is the control (untreated) event rate and EER is the experimental (treated) event rate in the OHTS population. This denominator is the absolute reduction in risk related to treatment to lower IOP. From the OHTS, we know that CER = 9.5% and EER = 4.4% in ocular hypertensive patients (treatment reduced the rate of 5-year progression by roughly half). Using the fact that EER = 0.46 × CER in this setting, we can calculate the average risk of glaucoma each physician would be willing to treat given his or her stated NNT:
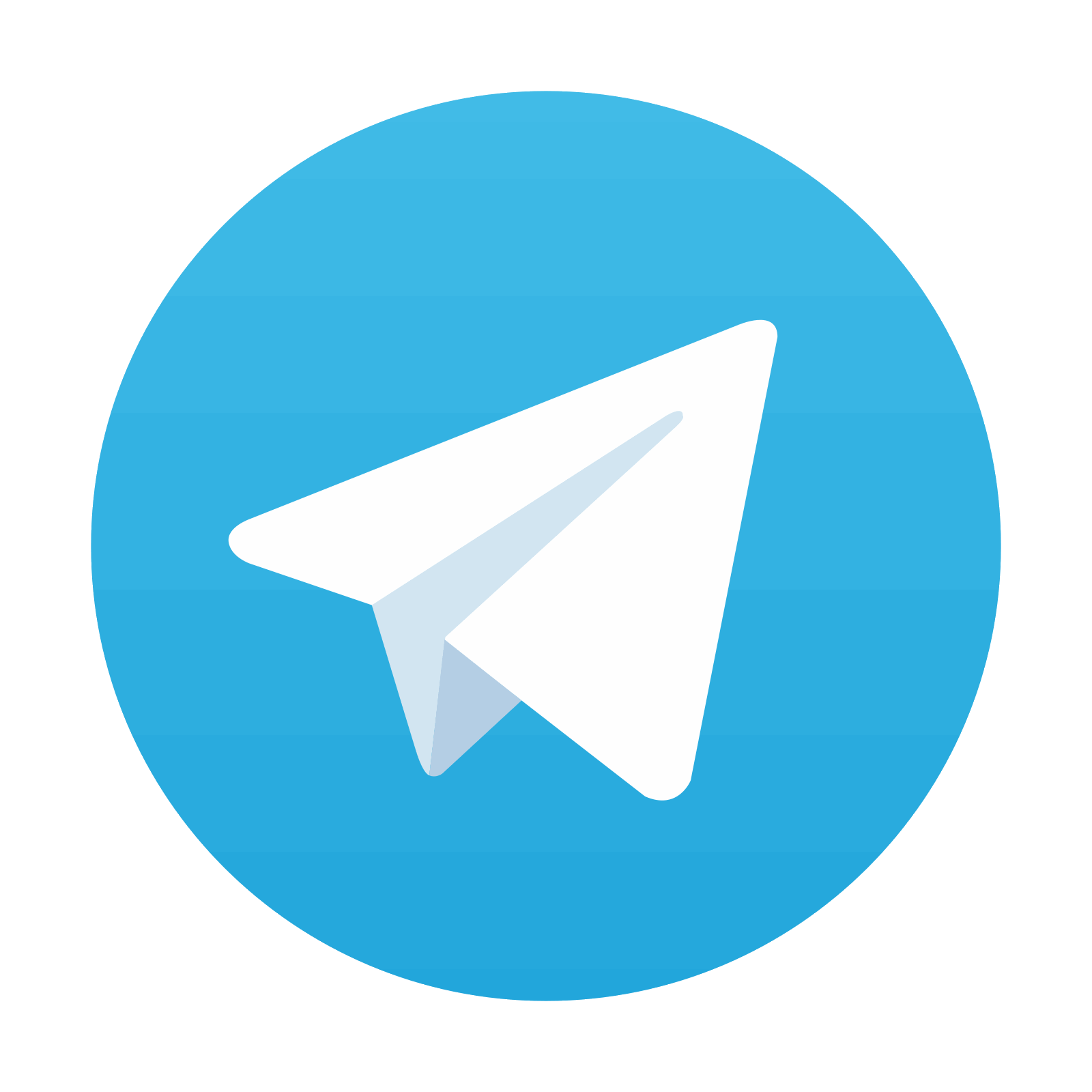
Stay updated, free articles. Join our Telegram channel

Full access? Get Clinical Tree
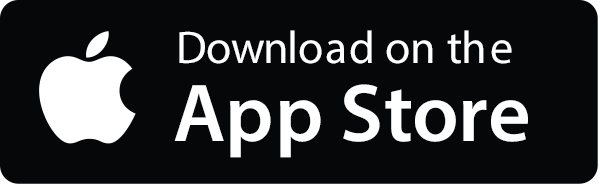
