Fig. 59.1
Temporal coherence model. The mixture (sum of one male and one female sentences) is transformed into an auditory spectrogram. Various features are extracted from the spectrogram including a multiscale analysis that results in a repeated representation of the spectrogram at various resolutions; pitch values and salience are represented as a pitch-gram; location signals are extracted from the interaural differences. All responses are then analyzed by temporal modulation band-pass filters tuned in the range from 2 to 16 Hz. A pair-wise correlation matrix of all channels is then computed. When attention is applied to a particular feature (e.g., female pitch channels), all features correlated with this pitch track become bound with other correlated feature channels (indicated by the dashed straight lines running through the various representations) to segregate a foreground stream (female in this example) from the remaining background streams
3 The Temporal Coherence Model
The proposed computational scheme emphasizes two distinct stages in stream formation (Fig. 59.1): (1) extracting auditory features and representing them in a multidimensional space mimicking early cortical processing and (2) organizing the features into streams according to their temporal coherence. Many feature axes are potentially relevant including the tonotopic frequency axis, pitch, spectral scales (or bandwidths), location, and loudness. All these features are usually computed very rapidly (<50 ms). Tokens that evoke sufficiently distinct (nonoverlapping) features in a model of cortical responses are deemed perceptually distinguishable and hence potentially form distinct streams if they are temporally anti-correlated or uncorrelated over relatively long time periods (>100 ms), consistent with known dynamics of the cortex and stream buildup.
Figure 59.1 illustrates these processing stages. Inputs are first transformed into auditory spectrograms (Lyon and Shamma 1997) followed by a multiresolution analysis analogous to that thought to occur in the primary auditory cortex (Chi et al. 2006). For the purposes of this model, this transformation is implemented in two steps: (1) a multiscale (spectral) analysis that maps incoming spectrograms into multiscale (bandwidth) representations, followed by (2) temporal rate analysis in which the temporal modulations of the (fine to coarse) multiscale spectrograms are analyzed by a filter bank tuned to rates from 2 to 16 Hz. In addition, other features such as pitch and location are estimated from the input spectrograms and the resulting tracks are later analyzed through the same rate analysis as for other channels, as illustrated in Fig. 59.1.
Subsequent to the feature and rate analysis, a pair-wise correlation matrix is computed among all scale-frequency-pitch-location channels, which is then used to group the channels into two sets representing the foreground and background streams. The responses are maximally correlated within each stream and least correlated across the two streams. One such factorization procedure is illustrated for the simple two-tone alternating (ALT) and synchronized (SYNC) sequences shown in Fig. 59.2. The correlation matrix cross-channel entries induced by these two sequences are quite different, being strongly positive (negative) for the SYNC (ALT) tones. A principal component analysis would then yield an eigenvector that can function as a “mask” to segregate the anti-correlated channels of the ALT stimulus, while grouping them together for the SYNC sequence, in agreement with their usual percept.
4 Attention and Binding
It remains uncertain if the representation of streams in the brain requires attention or is simply modulated by it (Carlyon et al. 2001; Sussman et al. 2007). But it is intuitively clear that attending selectively to a specific feature such as the pitch of a voice (symbolized by the yellow-shaded pitch region in Fig. 59.1) results in binding the pitch with all other voice attributes in the foreground stream while relegating the rest of the concurrent sounds to the background. To explain how this process may occur, we consider the simpler two-tone stimulus in Fig. 59.2. When attention is directed to a particular channel (e.g., yellow arrow to tone B), the entries in the correlation matrix along the row of the selected channel can readily point to all the other channels that are highly correlated and hence may bind with it. Basically, this row is an approximation of the eigenvector of the correlation matrix and can be used as “mask” to assign the channels to the different streams (rightmost panel). Note that in such a model, the attentional focus is essential to bring out the stream, and without it the correlation matrix remains unused. This idea is implemented to segregate the two-talker mixture in Fig. 59.1. Specifically, the female speech could be readily extracted by simply focusing on the rows of the correlation matrix corresponding to the female pitch (shaded yellow in Fig. 59.1) and then using the correlation values as a mask to weight all correlated channels from the mixture.
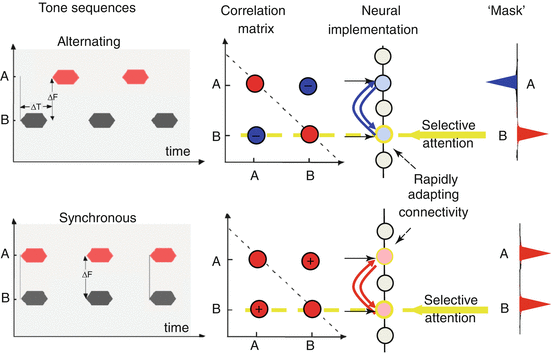
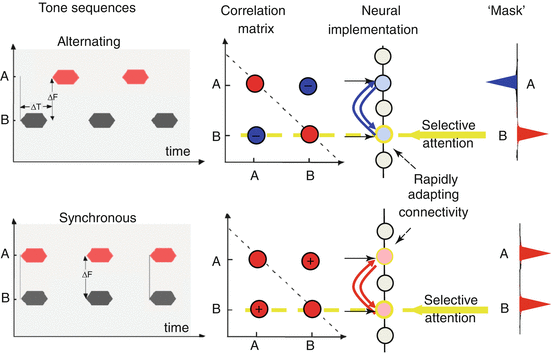
Fig. 59.2
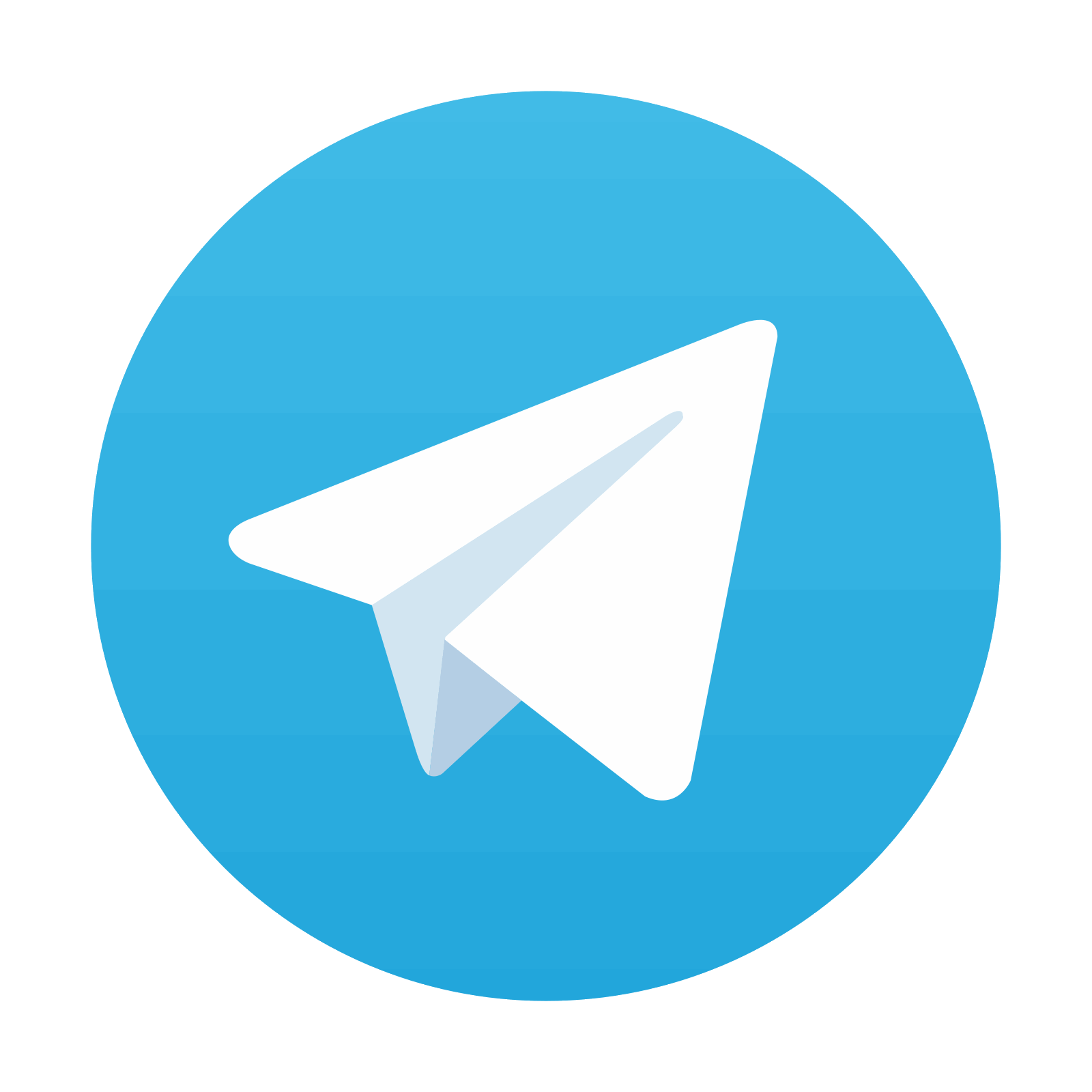
Streaming of two-tone sequences. Alternating tone sequences are perceived as two streams when tones are far apart (large ΔF) and rates are relatively fast (small ΔT). Synchronous sequences are perceived as a single stream regardless of their frequency separation. The correlation matrices induced by these two sequences are different: pair-wise correlations between the two tones (A, B) are negative for the alternating sequence and positive for the synchronous tones. Neural implementation of this correlation computation can be accomplished by a layer of neurons that adapts rapidly to become mutually inhibited when responses are anti-correlated (alternating tones) and mutually excitatory when they are coherent (synchronous tones). When selective attention (yellow arrow) is directed to one tone (B in this example), the “row” of pair-wise correlations at B (along the yellow dashed line) can be used as a mask that indicates the channels that are correlated with the B stream. For the alternating sequence, tone A is negatively correlated with B, and hence, the mask is negative at A and eliminates this tone from the attended stream. In the synchronous case, the two tones are correlated, and hence, the mask groups both tones into the attended stream
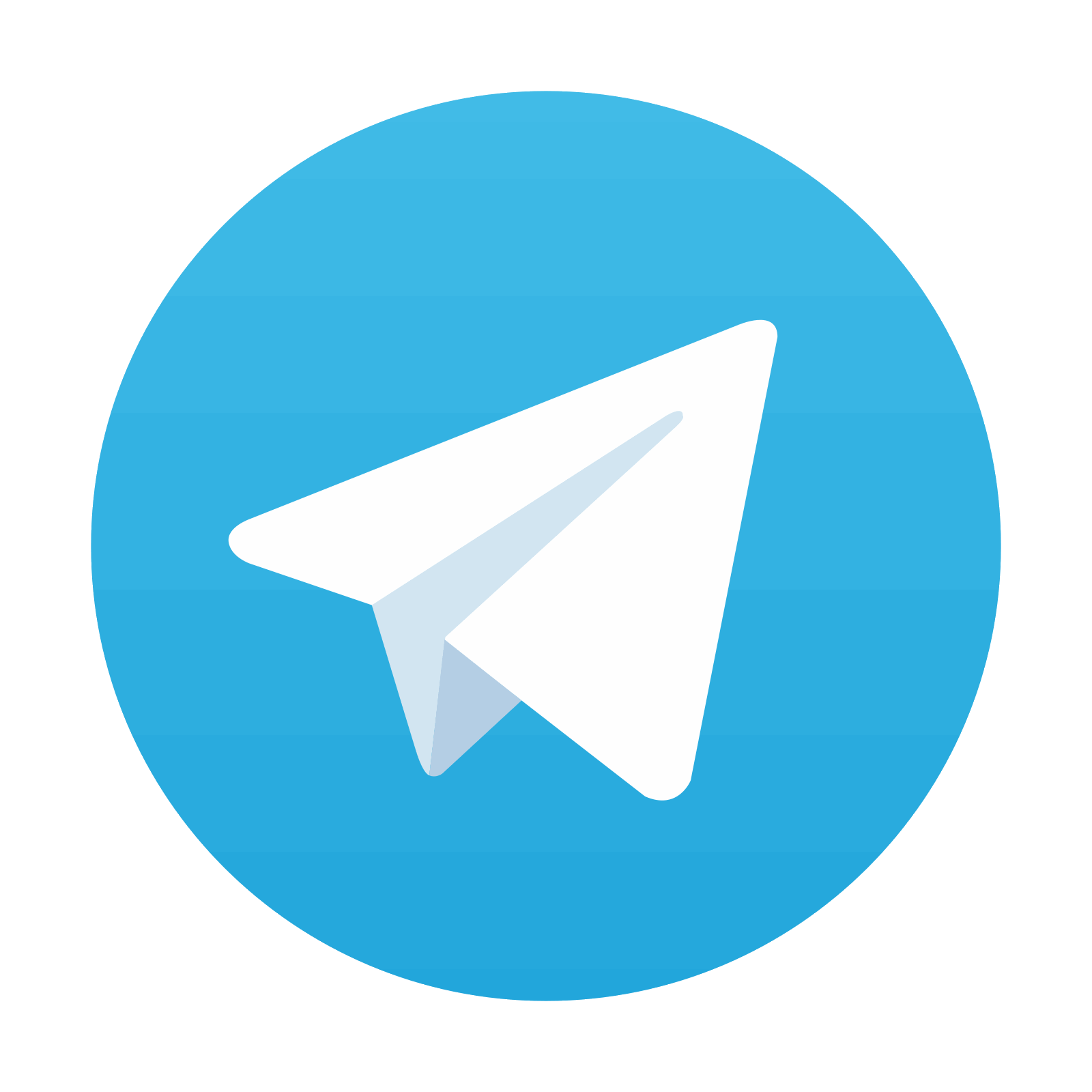
Stay updated, free articles. Join our Telegram channel

Full access? Get Clinical Tree
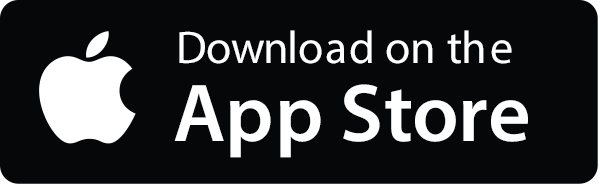

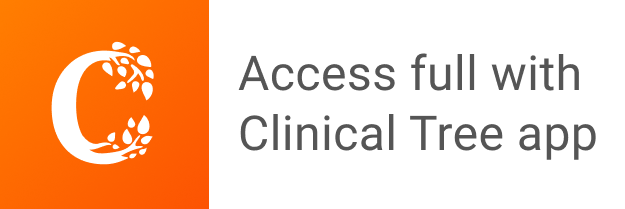