The design of nonrandomized studies for causal effect estimation is an important enterprise. Although propensity score methods are now standard statistical tools in many branches of medical research and epidemiology for attacking this goal, with rare exception, they have not been used in ophthalmic research. Because propensity score methods also have been misused in some applications in medicine, this editorial briefly outlines their correct use.
The propensity score is a patient’s probability of being treated versus control as a function of all relevant observed covariates—that is, observed pretreatment measurements possibly related to posttreatment outcomes. The reason for the popularity of propensity score methods arises because comparing outcomes of treated and control patients with the same true propensity score provides an unbiased estimate of the causal effect of treatment versus control for patients with that value of the propensity score, as proved by Rosenbaum and Rubin.
For example, in a completely randomized experiment with half the patients treated and the other half control, the propensity score for all patients is one half; in this case, we simply can compare the outcomes of all treated patients and all control patients to obtain an unbiased estimate of the causal effect of treatment versus control in the group of patients participating in the experiment. In a randomized block experiment (where randomization occurs within strata defined by observed characteristics, which create groups of patients, blocks of them), propensity scores can vary. For example, one could imagine treatment versus control being randomized separately for males and females, with 60% of males and 40% of females assigned the active versus the control treatment. Here, we should make within-male and within-female comparisons of outcomes for treated and control patients to avoid potential bias because of the greater proportion of males being treated.
Now suppose the probability of being treated versus being a control depends in a known way on medical measurements such that patients who are healthier at baseline are more likely to be treated; simply comparing the posttreatment outcomes of treated and control patients could well be biased because the treated patients were healthier at baseline than the controls. This bias can be corrected, however, even though the propensity scores for the treated tend to be higher than the propensity scores for the controls. Grouping treated patients and controls with the exact same value of the propensity score (e.g., 0.3) adjusts for all covariates that entered the propensity score calculation, that is, that are used to calculate the health of the patient, because this collection of patients can be viewed as a block of a randomized block experiment, each patient in the block having the same probability of being treated.
In nonrandomized studies, however, propensity scores are not known, although the researcher may be confident that all relevant covariates that are used to influence treatment assignment decisions are available and recorded accurately in the database. Such an assignment mechanism is called unconfounded . If a data set is not rich enough to make the assumption of an unconfounded assignment mechanism plausible, it is usually wise to look for better data. But if treatment assignment reasonably can be assumed to be unconfounded, even though the true propensity scores are unknown, we can estimate them using the observed covariates to predict treated versus control status, for example, from a logistic regression. Often, even relatively coarse adjustment for estimated propensity scores can create approximate “balance” on all observed relevant covariates, where balance means having nearly the same distribution of covariates within subgroups of treated patients and controls. For example, all patients can be ranked by their estimated propensity scores from low to high and then can be categorized into 5 to 10 approximately equal-sized subclasses (i.e., groups with the same number of patients), where treated and control patients within the same subclass can be considered as creating a minirandomized experiment. This technique is called subclassification or stratified matching . Often it will be impossible to achieve covariate balance using all the treated and control patients, in which case some controls, and possibly some treated patients, with extreme propensity scores should be discarded because there are no comparable patients in the other group. In such a case, conclusions will have to be restricted to the types of patients represented in both treatment groups.
Of critical importance to the objective design of nonrandomized studies for causal effects, no outcome data are used to estimate propensity scores; no outcome data should be available to the researcher at this time, just as in the design of a randomized experiment, so that there is no opportunity for the researcher to obtain, intentionally or unconsciously, an answer in either direction or of any particular magnitude. Rather, the sole goal of propensity score estimation is to obtain balance on the covariates, which is assessed using simple diagnostics. If balance is not achieved (e.g., within subclasses), the propensity scores should be reestimated, perhaps including transformations of or interactions among the original covariates. Only after the search for balance has ended may outcome data be examined.
In randomized studies, expected covariate balance is achieved as a consequence of randomization. At the stage of interpreting outcome results, however, there are legitimate concerns about conducting multiple analyses and the consequential invalidity of P values, but reestimation of propensity scores before looking at study outcomes raises no multiple comparison concerns; rather, this step simply aims to mirror, as closely as possible, the balancing properties of randomization.
To illustrate, the United States General Accounting Office reviewed earlier results from 6 randomized controlled trials (RCTs) comparing mastectomy and breast conservation therapy for the treatment of breast cancer for a class of patients (e.g., node-negative, relatively small tumors). The results provided no evidence of any benefit to the much more radical operation, at least for the type of women who participated in these RCTs and for the care at the participating centers. The question remained, however, how broadly these results could be generalized to other node-negative women with small tumors and less specialized treatment centers.
The General Accounting Office used the National Cancer Institute’s Surveillance, Epidemiology and End Results observational database to address this question because designing a new randomized experiment not only was ethically dubious, but its results, even if possible, would be years away. Entry restrictions were applied to the Surveillance, Epidemiology and End Results database to correspond to those for the RCTs, which reduced the database to 1106 women receiving breast conservation and 4220 receiving mastectomy. Approximately 20 covariates and interactions were identified that, according to experts, influenced doctors’ and women’s decisions to undergo breast conservation (e.g., age, tumor size, marital status). Logistic regression was used to predict breast conservation versus mastectomy from these covariates based on the data from the 5326 women, yielding an estimated propensity score—the estimated probability, based on covariate values, of receiving breast conservation rather than mastectomy—for each woman. The 5326 women then were into divided 5 approximately equally sized (1065 ± 1) subclasses based on their ranked estimated propensity scores. Before examining any outcome data, the subclasses were checked for balance to accord with propensity score theory that, within each subclass, the distribution of all covariates should be approximately the same in the treated and control patients. After some reestimation, this balance was found to be satisfactory. If assessing balance had included the examination of estimated causal effects on 5-year survival, then the selection of a particular propensity score model could have been used to bias the estimate of the causal effect in a so-called preferred direction. The objective design of a nonrandomized trial requires us to check for balance without being influenced by estimates of causal effects. Estimates of 5-year survival rates based on the resulting propensity score subclassification are given in the General Accounting Office report and are discussed by Rubin with emphasis on the imperative of finalizing propensity score estimation before evaluating outcomes. The average of the 5 subclass-specific differences between breast conservation and mastectomy was very small, consistent with the conclusions from the earlier RCTs.
Despite their broad usefulness, it is important to remember that propensity score methods can adjust only for observed covariates and not for unobserved ones, which is always a limitation of nonrandomized studies relative to RCTs. Because of this limitation, confidence in their causal conclusions must be built by seeing how consistent answers are with other evidence and with medical judgment, and how sensitive the conclusions are to reasonable deviations from the unconfoundedness assumption (where the determination of what is reasonable itself requires medical judgment).
Also, propensity score methods work better in large samples for the same reason that RCTs work better in large samples. As with randomized experiments, the distributional balance of observed covariates created by the propensity score is an expected balance. In small RCTs, random imbalances of some covariates can be substantial, and analogously, in small studies, substantial imbalances of some covariates may be unavoidable despite using a sensibly estimated propensity score. Nevertheless, any causal questions put to a nonrandomized database should be approached first using propensity score methods to see if the question can be addressed legitimately, and if so, for which subset of patients.
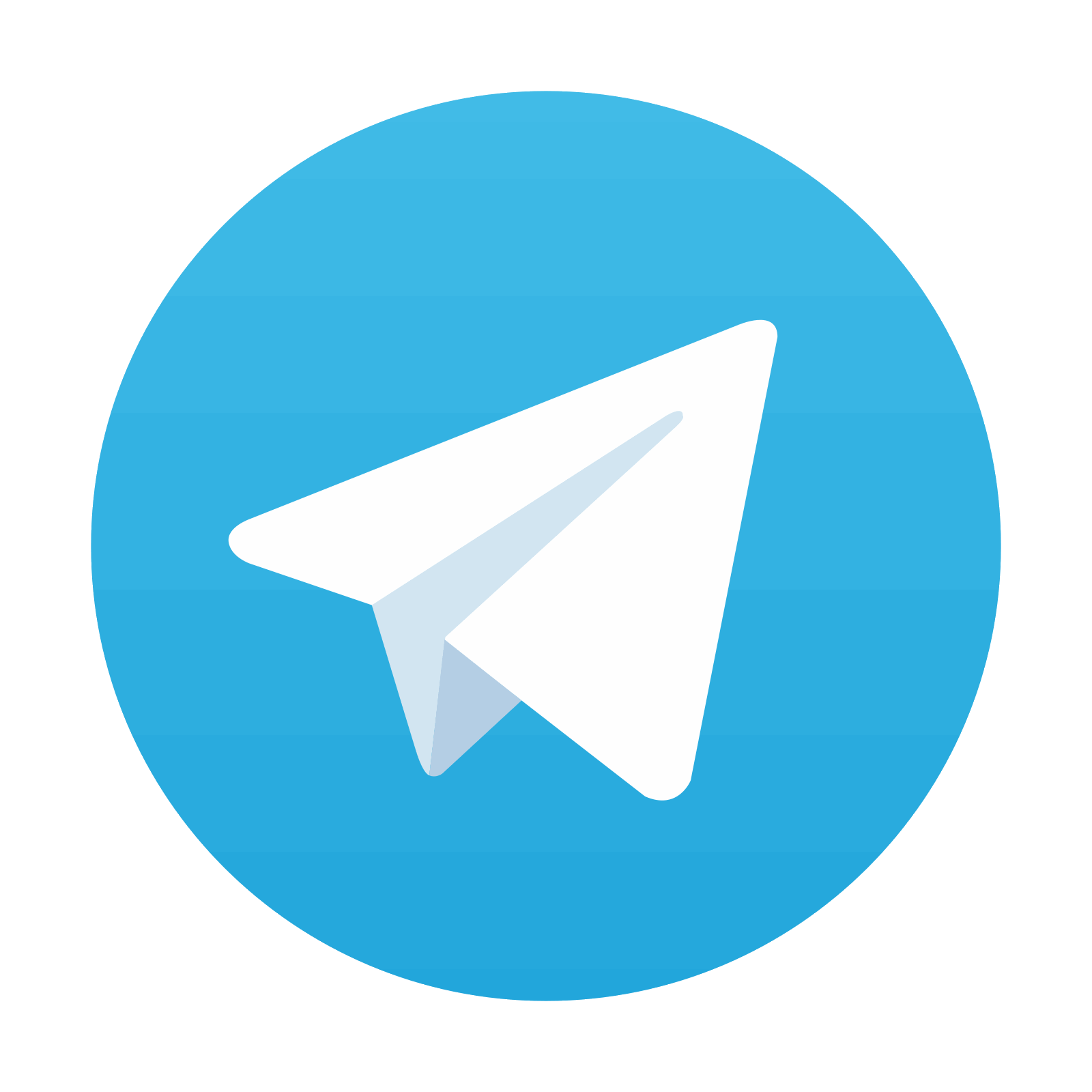
Stay updated, free articles. Join our Telegram channel

Full access? Get Clinical Tree
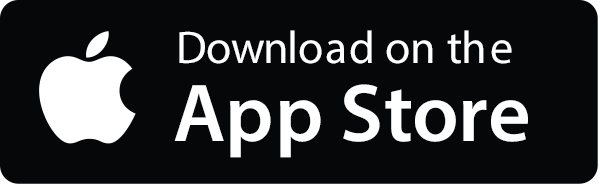
