Fig. 55.1
Proportion of total FFR contained in FFRENV and in FFRCAR at each harmonic of 100 Hz from (a) experimental measures and (b) model predictions
Modeling results also suggest that different acoustic frequencies contribute to FFRENV and FFRCAR. In the model, peripheral channels with the lowest characteristic frequencies (CFs) tend to contribute to FFRCAR and peripheral channels with the highest CFs contribute to FFRENV, with a crossover point of about 2 kHz (Fig. 55.2a). The model also predicts that the channels that contribute the most to the 100-Hz FFRENV for our /dah/ syllable have CFs in the mid-to-high frequency range, around 1 kHz (Fig. 55.2a).
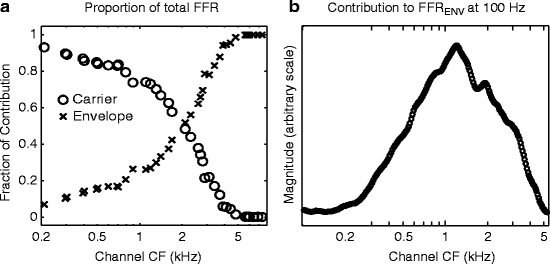
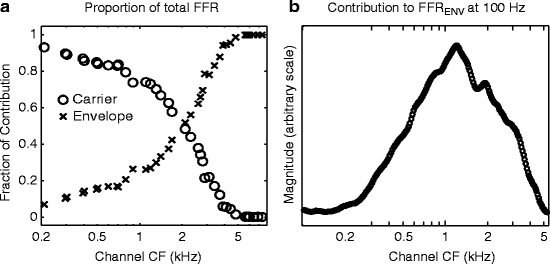
Fig. 55.2
(a) Relative strength of FFRENV and FFRCAR generated by each peripheral channel as a function of characteristic frequency (CF). (b) Relative contribution of each CF channel to strength of FFRENV at stimulus F0 of 100 Hz
3.2 Effects of Reverberation and Age on Selective Attention
Selective attention performance decreases as reverberant energy increases, reaching chance levels for all but five listeners in the highest reverberation level (Fig. 55.3; chance performance is one-third; modeling performance as a binomial distribution of 600 independent trials, we computed the 95 % confidence interval around this level).
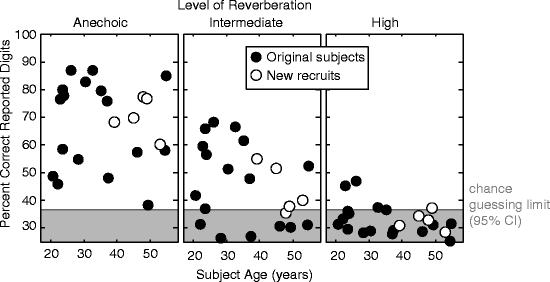
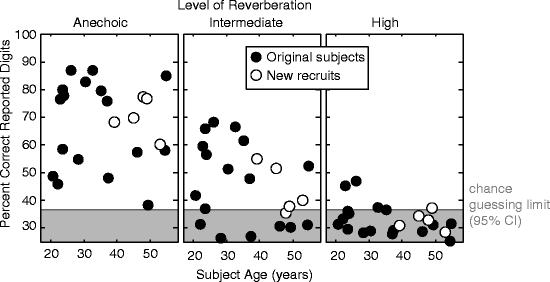
Fig. 55.3
Percentage of target digits correctly reported as a function of individual listener age for the three room conditions. Open symbols show subjects not in Ruggles et al. (2011)
We quantified the fidelity of envelope temporal structure encoding for each listener as the FFRENV at 100 Hz. To quantify coding of the temporal fine structure in the input stimulus, we took the average of FFRCAR for four harmonics (600–900 Hz, henceforth denoted FFRCAR-AV). Importantly, these two statistics are not significantly correlated (r = 0.03, p = 0.905, N = 22), supporting the modeling prediction that each component reflects different aspects of temporal coding precision driven by different tonotopic portions of the auditory pathway.
We performed a multi-way, repeated-measures ANOVA on the selective attention results with factors of reverberation, age, FFRENV-100, and FFRCAR-AV (treating reverberation as categorical and all other factors as continuous). Although there is no statistically significant effect of age on selective attention performance (Fig. 55.1a; F(1, 16) = 1.42, p = 0.251), there is a significant interaction between age and reverberation (F(1, 16) = 5.88, p = 0.025) and a significant main effect of reverberation (F(1, 16) = 155.17, p = 7.01 × 10−11). Although age does not predict how well an individual performs overall, the toll that reverberation takes increases with age.
3.3 Relationship Between FFR Components and Performance
Consistent with previous results showing that the total FFR strength at 100 Hz (a measure dominated by envelope phase locking; see Fig. 55.1) predicted selective attention ability (Ruggles et al. 2011), we find a significant main effect of FFRENV-100 on performance (F(1, 16) = 5.03, p = 0.040). Importantly, however, there is a significant interaction between age and FFRENV-100 (F(1, 16) = 4.64, p = 0.048). There is also a significant interaction between age and FFRCAR-AVE (F(1, 16) = 4.64, p = 0.047), with no main effect of FFRCAR-AVE (F(1, 16) = 0.216, p = 0.649). The regression coefficients of the ANOVA analysis reveal that the younger a listener is, the better FFRENV-100 is in predicting selective attention, whereas FFRCAR-AVE is a better predictor the older the listener. These results suggest that FFRENV-100 and FFRCAR-AVE reflect different perceptual cues that each aid in selective auditory attention but that are weighted differently as listeners age.
3.4 Individual Differences in FFR
Figure 55.4 plots FFRENV-100 and FFRCAR-AVE as a function of age. While both components tend to decrease as age increases, age is not significantly correlated with either FFRENV-100 or with FFRCAR-AVE. Notably, a good percentage of the younger adult listeners have strong FFRs (particularly for FFRENV-100), whereas nearly all the older listeners have weak FFRs. Thus, most of the variance in the FFRs is from the younger listeners and cannot be explained by age alone.
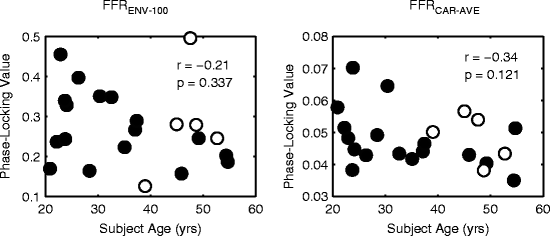
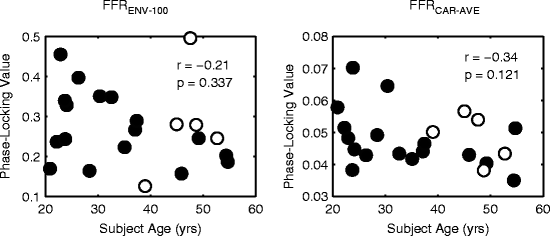
Fig. 55.4
(a) FFRENV-100 as a function of age. (b) FFRCAR-AVE as a function of age. Open symbols show subjects not in Ruggles et al. (2011)
3.5 Relationship Between FM Detection Threshold and Performance
We previously found that FM detection threshold, a measure thought to reflect coding fidelity of temporal fine structure (Moore and Sek 1996), was also related to attention performance (Ruggles et al. 2011). This relationship remains significant with our additional subjects, as shown in Fig. 55.5.