Purpose
To characterize the role of Big Data in evaluating quality of care in ophthalmology, to highlight opportunities for studying quality improvement using data available in the American Academy of Ophthalmology Intelligent Research in Sight (IRIS) Registry, and to show how Big Data informs us about rare events such as endophthalmitis after cataract surgery.
Design
Review of published studies, analysis of public-use Medicare claims files from 2010 to 2013, and analysis of IRIS Registry from 2013 to 2014.
Methods
Statistical analysis of observational data.
Results
The overall rate of endophthalmitis after cataract surgery was 0.14% in 216 703 individuals in the Medicare database. In the IRIS Registry the endophthalmitis rate after cataract surgery was 0.08% among 511 182 individuals. Endophthalmitis rates tended to be higher in eyes with combined cataract surgery and anterior vitrectomy ( P = .051), although only 0.08% of eyes had this combined procedure. Visual acuity (VA) in the IRIS Registry in eyes with and without postoperative endophthalmitis measured 1–7 days postoperatively were logMAR 0.58 (standard deviation [SD]: 0.84) (approximately Snellen acuity of 20/80) and logMAR 0.31 (SD: 0.34) (approximately Snellen acuity of 20/40), respectively. In 33 547 eyes with postoperative VA after cataract surgery, 18.3% had 1-month-postoperative VA worse than 20/40.
Conclusions
Big Data drawing on Medicare claims and IRIS Registry records can help identify additional areas for quality improvement, such as in the 18.3% of eyes in the IRIS Registry having 1-month-postoperative VA worse than 20/40. The ability to track patient outcomes in Big Data sets provides opportunities for further research on rare complications such as postoperative endophthalmitis and outcomes from uncommon procedures such as cataract surgery combined with anterior vitrectomy. But privacy and data-security concerns associated with Big Data should not be taken lightly.
It is with great pride that I greet you today for this 72nd Jackson Memorial Lecture as I regard this honor as being shared with colleagues, mentors, students, and all of the academic institutions with which I have been affiliated, including the University of California at Los Angeles, the Wilmer Eye Institute at Johns Hopkins, the University of Illinois, the Medical College of Virginia at Virginia Commonwealth University, Duke University, and the Collegiate School in Richmond, Virginia. For without the network of colleagues, friends, educators, students, mentors, and family that has surrounded me throughout my life and career, I would not be standing before you today. So first I would like to thank all of you who have interacted with me over the years for your support, guidance, critiques, friendship, intellect, integrity, and humor.
I approach this lecture with humility. None of us in this room had the pleasure of knowing Dr Edward Jackson, but based on my readings about him and his work, I am sure that we would all be honored to be in his presence, as his gifts to medicine and ophthalmology still impact all of us today. He not only gave us tangible discoveries that are named for him, such as the Jackson cross cylinder, but also his memory is forever documented and his legacy continues to grow through the Jackson Memorial Lectures, where we have been provided reviews and updates on ocular diseases, have been challenged about future directions of ophthalmology and medicine, and have been introduced to new hypotheses and theories about ocular diseases. Reviewing previous Jackson Memorial Lectures not only helped me decide how to organize my presentation today but also appealed to me as time capsules that offer perspectives on macro-level developments in our discipline.
Historical Perspective on the Standard of Care for Cataracts
As it happens, the last time cataract surgery was a topic for a Jackson Memorial Lecture was the 24th Jackson Memorial Lecture in 1967, when Dr Paul Chandler presented his paper, Surgery on Congenital Cataract. Considering that Neil Armstrong had not yet set foot on the moon at that point, I felt that it was time to revisit our most frequently performed surgical procedure. In 1932, Dr Jackson himself presented a paper to the Section of Medical History in Denver about the history of cataract operations. In his paper he presented findings from the 1917 Hunterian lectures of Colonel Elliot, who compared the results of couching vs cataract extraction among 780 patients at the Government Ophthalmic Hospital at Madras. A successful outcome was defined as vision of 20/200 or better, with partial success defined as being able to count fingers or better. The evidence favoring cataract extraction was so overwhelming as to obviate any formal statistical testing. Only 21.6% of couching patients had vision of 20/200 or better, compared to 96% with cataract extraction; the partial success rate of 16.7% in eyes that underwent couching and 2% in those that underwent cataract extraction raised the overall “success” rates to 38% and 98%, respectively, but as Dr Jackson pointed out, “the decrease of failures from 62 percent to 2 percent indicates the advance of modern surgery, the superiority of cataract extraction over couching.” Since then, advances in treatment and reassessments of what we regard as the standard of care have led to revisions in quality standards. No longer is the threshold for success set at vision of 20/200 or better. Rather, using Measure 191 of the Physician Quality Reporting System (PQRS) for the Center for Medicare and Medicaid Services as an example, vision of 20/40 or better is used as a quality measure, which seems more reasonable based on modern sensibilities.
The Evolving Nature of Quality of Care
Evolving quality standards provide yardsticks both for tracking success and identifying room for improvement. In 2013 Mats Lundström and colleagues from 15 European countries reported that the 2-month-postoperative visual acuity (VA) was 20/40 or better in 94.3% of 368 256 eyes that underwent cataract extractions and were included in the European Registry of Quality Outcomes for Cataract and Refractive Surgery (EUREQUO). We have made great strides in improving the VA outcomes of our procedures and continue to push the envelope to improve our success rates for postoperative VA to not just 20/40 but 20/20. In the EUREQUO registry 61.3% of cataract extractions had 2-month-postoperative VA of 20/20 or better. This was best-corrected distance vision; however, the current standards have been to provide better visual outcomes in patients without the need for glasses.
Device companies have been developing premium lenses, which are lenses that allow patients with cataract extractions to achieve excellent vision across a range of viewing distances. In 1997, the first premium intraocular lens in the United States was approved by the Food and Drug Administration (FDA). As of June 22, 2015, the FDA has approved intraocular lenses associated with 3 multifocal, 1 accommodating, 4 toric, and 2 phakic design platforms (Michelle Tarver, MD, PhD, Division of Ophthalmic and Ear, Nose, and Throat Devices [DOED], Office of Device Evaluation, Center for Devices and Radiological Health, Food and Drug Administration, written communication, June 25, 2015). The frequency of the implantation of a presbyopia correction lens by more than 1500 members of the American Society of Cataract and Refractive Surgery (ASCRS) was 7.2% in the 2014 ASCRS Clinical Survey.
Recently the American Academy of Ophthalmology (AAO) with the FDA sponsored a workshop on developing novel research endpoints for premium intraocular lenses. Since these lenses are reviewed by the FDA on a case-by-case basis, this workshop was designed to help with the development of standards for evaluation protocols and adverse-event occurrences that are applicable to premium intraocular lenses and generalizable to new variations. One of the workshop recommendations was to revise the current Safety and Performance Endpoints (SPEs) that have been used in clinical trials as historical benchmarks so that device companies do not have to have active control groups and can use smaller samples sizes for their investigative studies. As an illustration, the risk tolerance for postoperative endophthalmitis is operationalized through SPE rates of 0.1% for posterior chamber intraocular lenses and 0.2% for anterior chamber intraocular lenses. Incorporating an allowance for finite-sample variability, for a new intraocular lens to have an acceptable safety profile, the frequency of cumulative endophthalmitis cannot be statistically significantly greater than 0.1% if it is a posterior chamber intraocular lens.
Big Data as a Resource for Evaluating Quality of Care
Since cataract surgery has become so successful, it can be hard to detect improvements because the rates of postoperative complications are so small. We can use historical controls with SPEs as the FDA has done, or we can use large sample sizes or datasets. With large enough datasets, it is possible to investigate rare events, such as cataract surgery complications, with the goal of improving the success rates of cataract surgery by decreasing the complication rate even more. The title of my talk incorporates a term that has recently caught on, entering the lexicon as a sound bite that encompasses what I mean by “large enough datasets,” and that term is “Big Data.”
What is Big Data? One definition of Big Data, a little tongue-in-cheek, is that it involves a dataset that does not fit in an Excel spreadsheet. Another commonly used definition of Big Data makes reference to what are known as the 3 Vs, namely that there is a large volume and variety of data that is growing at a rapid velocity. Some observers have added a fourth “v,” veracity, to call attention to imperatives having to do with data quality; others have added a fifth “v,” variability, and the current Wikipedia page for “Big Data” alludes to a sixth characteristic, “complexity,” apparently in a nod to the notion that we should feel free to use the whole alphabet and not artificially constrain ourselves to 1 letter, veritably valuable and verifiably valid though that letter may be. A UCLA faculty colleague in the Department of Biostatistics, Professor Tom Belin, also known as my husband, pointed me to an American Statistical Association blog that identified 79 abstracts for presentations at this summer’s Joint Statistical Meetings that referred specifically to “Big Data.” Clearly, Big Data has become a big deal.
The Evolving Nature of Quality of Care
Evolving quality standards provide yardsticks both for tracking success and identifying room for improvement. In 2013 Mats Lundström and colleagues from 15 European countries reported that the 2-month-postoperative visual acuity (VA) was 20/40 or better in 94.3% of 368 256 eyes that underwent cataract extractions and were included in the European Registry of Quality Outcomes for Cataract and Refractive Surgery (EUREQUO). We have made great strides in improving the VA outcomes of our procedures and continue to push the envelope to improve our success rates for postoperative VA to not just 20/40 but 20/20. In the EUREQUO registry 61.3% of cataract extractions had 2-month-postoperative VA of 20/20 or better. This was best-corrected distance vision; however, the current standards have been to provide better visual outcomes in patients without the need for glasses.
Device companies have been developing premium lenses, which are lenses that allow patients with cataract extractions to achieve excellent vision across a range of viewing distances. In 1997, the first premium intraocular lens in the United States was approved by the Food and Drug Administration (FDA). As of June 22, 2015, the FDA has approved intraocular lenses associated with 3 multifocal, 1 accommodating, 4 toric, and 2 phakic design platforms (Michelle Tarver, MD, PhD, Division of Ophthalmic and Ear, Nose, and Throat Devices [DOED], Office of Device Evaluation, Center for Devices and Radiological Health, Food and Drug Administration, written communication, June 25, 2015). The frequency of the implantation of a presbyopia correction lens by more than 1500 members of the American Society of Cataract and Refractive Surgery (ASCRS) was 7.2% in the 2014 ASCRS Clinical Survey.
Recently the American Academy of Ophthalmology (AAO) with the FDA sponsored a workshop on developing novel research endpoints for premium intraocular lenses. Since these lenses are reviewed by the FDA on a case-by-case basis, this workshop was designed to help with the development of standards for evaluation protocols and adverse-event occurrences that are applicable to premium intraocular lenses and generalizable to new variations. One of the workshop recommendations was to revise the current Safety and Performance Endpoints (SPEs) that have been used in clinical trials as historical benchmarks so that device companies do not have to have active control groups and can use smaller samples sizes for their investigative studies. As an illustration, the risk tolerance for postoperative endophthalmitis is operationalized through SPE rates of 0.1% for posterior chamber intraocular lenses and 0.2% for anterior chamber intraocular lenses. Incorporating an allowance for finite-sample variability, for a new intraocular lens to have an acceptable safety profile, the frequency of cumulative endophthalmitis cannot be statistically significantly greater than 0.1% if it is a posterior chamber intraocular lens.
Big Data as a Resource for Evaluating Quality of Care
Since cataract surgery has become so successful, it can be hard to detect improvements because the rates of postoperative complications are so small. We can use historical controls with SPEs as the FDA has done, or we can use large sample sizes or datasets. With large enough datasets, it is possible to investigate rare events, such as cataract surgery complications, with the goal of improving the success rates of cataract surgery by decreasing the complication rate even more. The title of my talk incorporates a term that has recently caught on, entering the lexicon as a sound bite that encompasses what I mean by “large enough datasets,” and that term is “Big Data.”
What is Big Data? One definition of Big Data, a little tongue-in-cheek, is that it involves a dataset that does not fit in an Excel spreadsheet. Another commonly used definition of Big Data makes reference to what are known as the 3 Vs, namely that there is a large volume and variety of data that is growing at a rapid velocity. Some observers have added a fourth “v,” veracity, to call attention to imperatives having to do with data quality; others have added a fifth “v,” variability, and the current Wikipedia page for “Big Data” alludes to a sixth characteristic, “complexity,” apparently in a nod to the notion that we should feel free to use the whole alphabet and not artificially constrain ourselves to 1 letter, veritably valuable and verifiably valid though that letter may be. A UCLA faculty colleague in the Department of Biostatistics, Professor Tom Belin, also known as my husband, pointed me to an American Statistical Association blog that identified 79 abstracts for presentations at this summer’s Joint Statistical Meetings that referred specifically to “Big Data.” Clearly, Big Data has become a big deal.
Scientific, Pragmatic, and Societal Considerations
There are a number of issues raised by the availability of larger and larger datasets, including scientific considerations relevant to interpretation of results, pragmatic matters relevant to data processing and analysis, and societal concerns related to the power of information to affect interpersonal and social dynamics. In my own work I have made considerable use of Big Data sets, including early in my career for my epidemiology PhD thesis, using Medicare data to study complications associated with glaucoma surgery. More recently, I have used Medicare data to study associations between cataract surgery and subsequent fractures that might be anticipated among elderly patients who fall, revealing that cataract surgery helped to prevent falls, particularly for the most elderly patients. That finding might seem counterintuitive at first because older patients might be expected to experience more setbacks from surgery, but on the other hand, they are at greater risk of falling and thus can be understood as having more to gain.
Illustrative Example: Evaluating Endophthalmitis Rates Using Medicare Claims Data
To shed light on what Big Data has to offer medical providers in the 21st century, I’ll start with an illustrative example, making use of data from a 5% sample of Medicare claims from 2010, 2011, 2012, and 2013. One might ask, “Is there evidence of a change in endophthalmitis rates following cataract surgery across the time span from 2010 to 2013?” In Table 1 the annual endophthalmitis rate fluctuates between 0.13% and 0.16%. A χ 2 statistical test yields a P value of .35, indicating that the year-to-year variation in endophthalmitis cases is consistent with what would be expected by chance across 53 000–55 000 surgeries per year with an overall rate of endophthalmitis of 0.14%. Thus, even though we might expect the large sample sizes to provide substantial sensitivity to detect differences in endophthalmitis rates as being statistically significant, the data presented here do not provide convincing evidence of any trend or other systematic pattern in endophthalmitis rates across the time span.
Year | Number of Cases With Cataract Surgeries | Number of Endophthalmitis Cases After Cataract Surgery During the Year | Rate (%) of Endophthalmitis Cases After Cataract Surgery During the Year |
---|---|---|---|
5% Medicare claims files (by patients) | |||
2010 | 54 819 | 73 | 0.13% |
2011 | 53 947 | 88 | 0.16% |
2012 | 53 771 | 68 | 0.13% |
2013 | 54 166 | 71 | 0.13% |
Overall (2010–2013 combined) | 216 703 | 300 | 0.14% |
Intelligent Research in Sight (IRIS) Registry data files (by patients) | |||
2013 and 2014 combined | 511 182 | 400 | 0.08% |
Intelligent Research in Sight (IRIS) Registry data files (by eyes) | |||
2013 and 2014 combined | 736 197 | 441 | 0.06% |
Some related questions might then arise: how large would the magnitude of year-to-year differences need to be in order to emerge as statistically significant with comparable sample sizes? How large would samples have to be to provide a good chance, say 80% power, to detect as significant a 25% increase in the endophthalmitis rate (ie, from 0.14% to 0.175%)? How large would the endophthalmitis rate have to be to provide a solid foundation for judging a new procedure studied on 100 cases to have an unacceptably high endophthalmitis rate? These questions veer into the realm of statistical power calculations. It turns out that with 58 786 patients in each of 2 groups, one has 80% power to detect as significant a difference between an endophthalmitis rate of 0.14% in 1 group and 0.21% in the other, an elevation of 50% in the rate when considered in percentage terms that would translate into an excess of 41 cases in absolute terms. It would take 207 182 in each of the 2 groups to have 80% power to detect a 25% elevation in a baseline endophthalmitis rate of 0.14% as statistically significant. Against a background rate of 0.14%, a new procedure studied on 100 cases will only yield 80% power to detect a statistically significant elevation in the endophthalmitis rate if the true rate for a new procedure exceeds 2%. (This illustrates the fundamental principal of statistical power: to find a statistically significant difference using a small sample, that difference must be large. You need a large sample [often a very large sample] to find a small, statistically significant difference.) If we care about less extreme elevations in surgical complication rates—which of course we do, as reflected in the discussion of SPEs at the recent AAO/FDA workshop on intraocular lenses—we need to consider tracking evidence by integrating Big Data into a post-FDA-approval surveillance environment.
Types of Big Data
I have ventured into the realm of Big Data down the path of government databases, but there are other forms of big data that must be included in this discussion for completeness. Broadly, some observers have classified health-related big data into 3 categories : (1) Traditional medical data, which are primarily originated from the health system (eg, electronic health records, personal and family history, medication history, lab reports, pathology results). The objective of analysis of these data is to obtain a better understanding of disease outcomes and their risk factors, reduce health system costs, and improve its efficiency. (2) “Omics” data, referring to large-scale datasets in the biological and molecular fields (eg, genomics, microbiomics, proteomics, and metabolomics). The aim of analysis of this type of information is to understand the mechanisms of diseases and accelerate the individualization of medical treatments. For example, in the case of developing accurate predictive genetic risk models, genomic testing and mapping could be used in the future to identify high-risk individuals for developing neovascular age-related macular degeneration, which would allow providing them with preventive care. Such information has the potential to reduce the need for large-scale, potentially hazardous future interventions for other low-risk individuals. (3) Data from social media, which include signs and behaviors on how individuals use the Internet, social media, mobile applications, sensor devices, wearable computing devices, or other technological and nontechnological tools to better inform and enhance their health. These tools can give information about individuals’ food consumption, diet, lactation, et cetera. In recent years, there is an evolving definition of patient-generated data that refers to patient-sourced information of all types. Patient-reported outcomes represent a portion of this type of data, which are collected using questionnaires. The Patient-reported Outcome Task Force of the Patient-Centered Outcomes Research Institute (PCORI) defines patient-generated data as “health-related data—including health history, symptoms, biometric data, treatment history, lifestyle choices, and other information—created, recorded, gathered, or inferred by or from patients or their designees (ie, care partners or those who assist them) to help address a health concern.” For example, this type of data includes vital signs measured and recorded by the patient or proxy, self-reported lifestyle data, self-reported quality-of-life data, and information from biometric sensors or home glucose monitoring.
The Centers for Medicare and Medicaid Services (CMS) is making Medicare claims data available to researchers and what the Affordable Care Act refers to as “qualified entities” for analysis. Analyzing these data together with claims data from private insurers can offer meaningful insights. However, these efforts would not be characterized as fully leveraging Big Data’s information potential, as might happen if CMS data were combined with differently formatted data (for example, information from patients’ electronic health records or wearable devices or social media); such an approach would underscore how pooling a variety of data sources could expand knowledge by assembling the necessary ingredients to study associations connecting patient characteristics, clinical treatments, and patient health outcomes. For example, information regarding the number of steps that an individual patient walks per day from a wearable device could be linked to their Medicare claims data or the Intelligent Research in Sight (IRIS) Registry to determine whether there is increased physical activity after cataract surgery and whether this activity is affected by postoperative VA.
Previous Uses of Big Data in Ophthalmology
Returning to the use of the Medicare claims data to inform ophthalmic care, I view the 1991 manuscript by Jonathan Javitt, Al Sommer, and co-authors as an important contribution to the field and one that influenced me greatly. They reported that in 1984, when 58% of 338 141 cataract surgeries were extracapsular cataract extractions, 30% were intracapsular cataract extractions, and 8% were phacoemulsification, the risk of rehospitalization for endophthalmitis in the year following cataract surgery was 0.17% in patients who had intracapsular cataract extractions vs 0.12% in those with extracapsular cataract extractions or phacoemulsification, a finding that was statistically significant, with a P value of <.002. In addition, patients who had complicated cataract surgeries with an anterior vitrectomy had a 0.58% rate of endophthalmitis at 1 year compared to 0.13% for those with cataract surgery alone ( P < .0001). Since then, several investigators have reported on the rate of endophthalmitis using the Medicare database or other large data sources. Emily West and Oliver Schein and co-authors found that the rate of endophthalmitis was 0.179% within 6 months of cataract surgery in 1994 and 0.247% in 2001. They hypothesized that this increasing rate of endophthalmitis was secondary to the use of clear corneal incisions, which became more popular in the late 1990s. In addition, they found that among patients 80–89 years old the rate was 0.23% and 90 years or older, 0.319%. In the analysis of the complete Medicare database of cataract surgeries, the annual endophthalmitis rate had decreased to 0.133% in 2003 and 0.111% in 2004. Part of the decrease in the rate could be secondary to the inclusion of only endophthalmitis cases within 42 days of the cataract surgery date instead of within the year following cataract surgery or within 6 months, as was used in the prior studies. Another possible explanation given by the authors was changes in wound construction in the early 2000s. Interestingly, the rate of endophthalmitis in Canada during the same time period was similar to that observed in the United States (0.14%), while the annual endophthalmitis rate reported in Sweden by Lundström and co-authors from the Swedish National Cataract Registry was 0.048%.
In my work with the AAO’s H. Dunbar Hoskins, Jr, MD, Center for Quality Eye Care, which I am proud to have served as founding director, I have worked with Dr Flora Lum and the AAO Public Health Committee to investigate trends in cataract surgery outcomes in the United States using the 5% public use Medicare database files. Although several investigators in the United States have used the Medicare database and other large databases such as those of the Veterans Administration Hospital and Kaiser Permanente to investigate trends in cataract surgery outcomes and complications, our goals included investigating not only the current status and trends in the 5% Medicare sample but also the personal-level data from patients who have had cataract surgery and are available in the IRIS Registry.
The AAO IRIS Registry as an Additional Big Data Source
The AAO initiated the IRIS Registry in March 2014 as a longitudinal, clinical data registry to advance knowledge in the field and to enhance tracking and improvement of patient outcomes over time. The IRIS Registry as of December 31, 2014, already included 6.5 million unique patients with 17.2 million patient encounters, allowing ophthalmologists to meet quality reporting requirements of federal programs, as well as to track their own performance and compare their patients’ outcomes with national benchmarks (Flora Lum, MD, written communication, June 11, 2015). The IRIS Registry uses a software tool capable of extracting data automatically from a practice’s electronic health record (EHR) system, thus avoiding manual entry by staff members and interruptions to the everyday workflow. This approach can be adapted to any EHR system, and the IRIS Registry is also able to receive standardized files from cloud-based EHR systems. Integration can also be achieved by interfacing with practice management systems to extract procedure codes used for billing purposes, if not available in the EHR system.
By evaluating the systemic and ocular factors associated with success and complications, the AAO hopes to provide better guidance and benchmarks for the improvement of the quality of cataract surgery and eye care in general for the US population. To emphasize the potential for Big Data to inform our clinical activities, I thought it would be useful to present some new findings, first from further recent analyses of Medicare data from 2010 to 2013 and then from recent analysis of the IRIS Registry database from 2013 to 2014.
Methods
Methods for Medicare Claims Databases
Patient selection
We first obtained the 5% random sample of Medicare physician suppliers Part B files from the Centers for Medicare & Medicaid Services’ claims for the service of cataract surgery in each year from 2010 to 2013. Cataract surgery cases were identified based on the Current Procedural Terminology (CPT) codes for cataract surgery, including 66840, 66850, 66852, 66920, 66930, 66940, 66982, 66983, and 66984 ( Appendix 1 ; Supplemental Material available at AJO.com ). If multiple claims of cataract surgery occurred for the same patient on the same date, we considered them as a single surgery. The first occurrence of cataract surgery within each year was considered as the index surgery for each Medicare beneficiary. Therefore, the unit of analysis is the patient, and index surgeries were defined by patient. The date of index cataract surgery was recorded, along with the number of cataract surgeries for each Medicare beneficiary during each year.
All beneficiaries who underwent cataract surgery were then merged with the Denominator file in each year to obtain their demographic and background information. Beneficiaries who met the following conditions were excluded from further analysis: a) age younger than 65 years; b) residence outside the 50 US states or District of Columbia; c) lack of Medicare Part B coverage; and d) health maintenance organization coverage not processed by the CMS.
Outcomes
The primary outcome of interest was the presence of endophthalmitis after the index cataract surgery within each year. The presence of postoperative endophthalmitis was determined by the Medicare physician suppliers Part B claims for endophthalmitis-related service using the International Classification of Diseases, 9th Revision, and Clinical Modification (ICD-9-CM) diagnosis codes, including 360.0x ( Appendix 2 ; Supplemental Material available at AJO.com ). The date of the first occurrence of postoperative endophthalmitis after the index cataract surgery was also recorded, and the time interval between the index cataract surgery and the first occurrence of postoperative endophthalmitis was calculated.
There are well-known limitations associated with the use of claims databases such as the Medicare database, which does not include laterality of the eye. Thus, there could be misattribution of the source of postoperative endophthalmitis, because the eye that received cataract surgery could be different from the eye that had the postoperative endophthalmitis.
Exposures
The exposures of interest included whether the index cataract surgery was combined with other ocular procedures or not. The occurrence of combined cataract surgery was determined using CPT codes ( Appendix 1 ) by the Medicare physician suppliers Part B claims for any ocular-related procedures occurring on the same day as the index cataract surgery. Combined cataract surgery was further characterized into the following classifications: a) any combined cataract surgery, b) combined cataract surgery and anterior vitrectomy, and c) combined cataract and glaucoma surgery.
Methods for IRIS Registry databases
Patient selection
In parallel evaluations using cataract surgery data in the IRIS Registry databases provided by AAO, all cataract surgery performed between January 1, 2013 and December 31, 2014 were extracted (data provided by Craig Salman and Flora Lum, MD, Quality of Care and Knowledge-based Development, American Academy of Ophthalmology on May 21, 2015). As opposed to the Medicare database, the IRIS Registry database has information on laterality of surgery, so the index surgery was defined by eye. If multiple cataract surgeries occurred in the same eye on the same date, they were considered as a single surgery. If there was an indication for bilateral cataract surgery, the bilateral cataract surgery was considered as 2 cataract surgeries, 1 in each eye. The first occurrence of cataract surgery in each eye during the study period was considered as the index surgery for that eye. The date of index cataract surgery was recorded, along with the number of cataract surgeries each patient had during the study period.
Outcomes
The primary outcome of interest was the presence of endophthalmitis after the index cataract surgery during the study period. The presence of postoperative endophthalmitis was determined from the endophthalmitis database provided by AAO, using ICD-9 and International Classification of Diseases, 10th revision (ICD-10) codes ( Appendix 2 ). The date of the first occurrence of postoperative endophthalmitis after cataract surgery in each eye was also recorded, and the time interval between the index cataract surgery and the presence of postoperative endophthalmitis was calculated. For the purpose of comparing postoperative endophthalmitis rates between IRIS Registry and Medicare databases, the rate of endophthalmitis was analyzed and presented both by eyes and by patients. In the by-patient analysis, only 1 eye per patient was included in the analysis, and the eye with first cataract surgery during the study period was selected for patients with cataract surgery in both eyes during the study period.
A secondary outcome of interest was VA before and after the index cataract surgery. Because VA data are not available in the Medicare database, there was no foundation for the comparison of VA results between the IRIS Registry and Medicare databases. We used eyes as the units of analysis for studying VA in the IRIS Registry. For each index cataract surgery, data were obtained on preoperative VA within 30 days of the index cataract surgery as well as postoperative VA within 1 week, 1 month (30 ± 15 days), and 3 months (90 ± 30 days) after the index cataract surgery. If there were multiple VA measurements performed on the same day, the value used for analysis was taken in the following order, depending on availability: (1) distance VA, near VA, or unspecified; (2) best-corrected VA, uncorrected VA, or unspecified. The VA value was recorded as Snellen acuity, and then converted to the logarithm of the minimal angle of resolution (logMAR) for analysis. In order to compare the results with other studies, the VA value was also evaluated according to 2 dichotomous thresholds: 20/20 or better and worse than 20/40.
Exposures
The exposures of interest included whether the index cataract surgery was combined with other ocular procedures or not. The occurrence of combined cataract surgery was also determined based on the same CPT codes used in the Medicare databases analysis ( Appendix 1 ), including: a) any combined cataract surgery, b) combined cataract and glaucoma surgery, and c) combined cataract surgery and anterior vitrectomy.
Statistical Analysis
All statistical analyses were performed using SAS software version 9.4 (SAS Institute, Inc, Cary, North Carolina, USA). Descriptive statistics were calculated for all variables of interest (outcomes and exposure variables). Mean, standard deviation (SD), and median were calculated for continuous variables, while frequency and percentage were calculated for categorical variables. The rate of postoperative endophthalmitis was calculated as the percentage of patients (or eyes) with the event among all patients (or eyes) with cataract surgery and among patients (or eyes) with each of the exposure variables. The rates of VA above or below particular thresholds were calculated as percentages among all eyes for which VA was available. Statistically significant differences in rates of outcomes between patients (or eyes) with and without the exposure variables were assessed using Fisher exact tests.
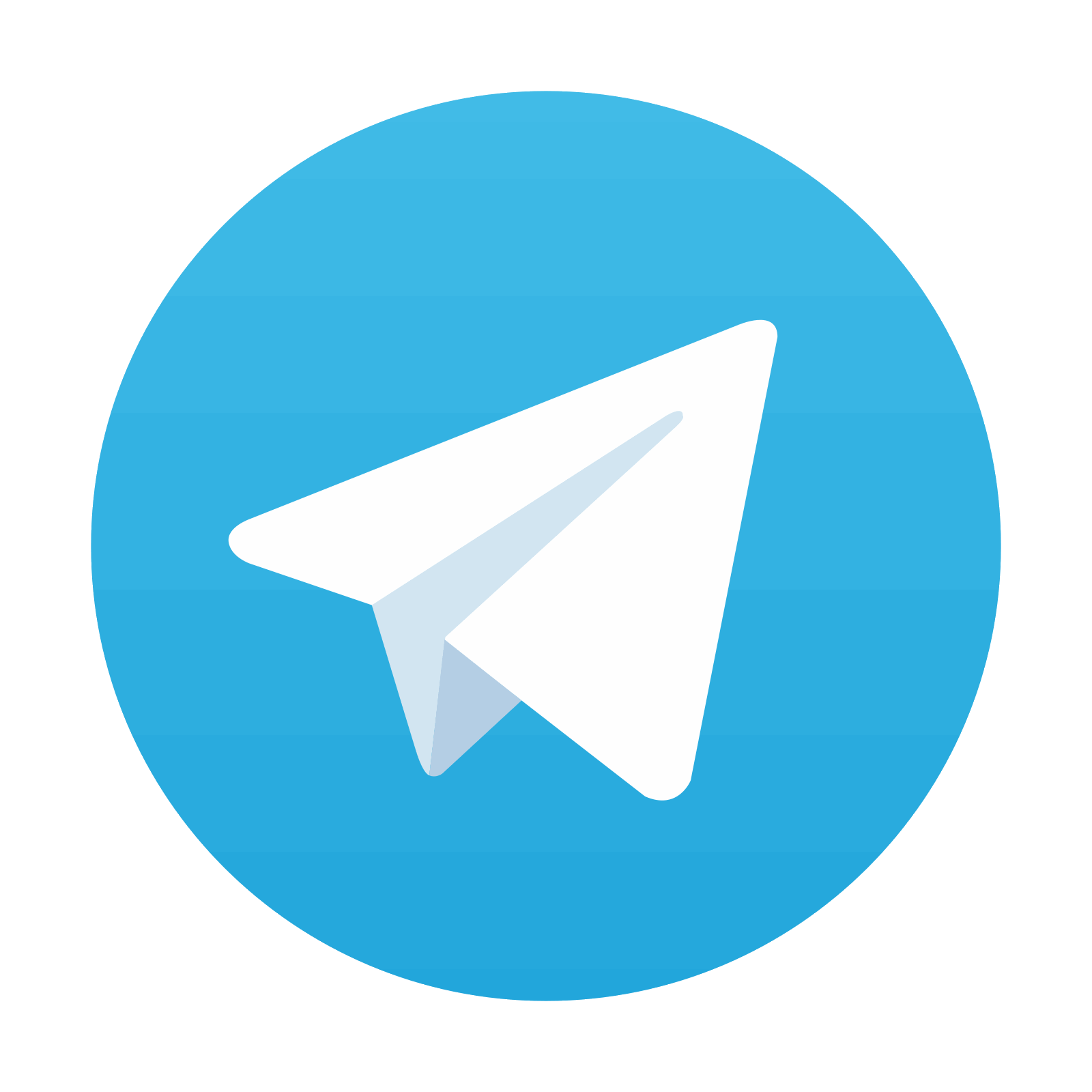
Stay updated, free articles. Join our Telegram channel

Full access? Get Clinical Tree
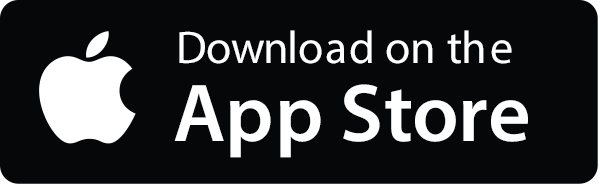
