Fig. 20.1
Types of study designs
Descriptive studies include case reports and series. These types of studies do not have a comparison group and therefore are primarily hypothesis-generating studies. They do not provide evidence of an association. Comparative or analytical studies compare two or more groups of patients and include cohort studies, case-control studies, and randomized clinical trials. Because there are comparison groups, the presence or absence and strength of an association can be described. A randomized controlled trial is also an experimental study and offers the highest degree of evidence of a therapeutic effect. Descriptions of each study type appear below.
Case Reports and Case Series
A case report or series describes the clinical findings or course of a single or multiple patients, respectively. These studies are very helpful for describing a unique case, novel syndrome, or therapeutic approach and to generate hypotheses to be evaluated with an analytical study. However, without a comparison group, the existence of or strength of an association between the risk factor (medication, clinical symptom or sign, surgical procedure, etc.) and the outcome (disease, surgical outcome, complication, etc.) cannot be determined. With a single case report, it is not possible to know if the findings are generalizable to other patients, as the patient may be unique. A case series is useful for reporting the clinical characteristics of a disease or group of patients who undergo a certain procedure, but without a comparison group, it is not possible to know if the characteristics are unique to that disease or if that procedure is truly associated with a higher success rate in comparison to other procedures. Key point: Much of the surgical literature consists of case series, so keep in mind these fundamental issues, which limit t he conclusions that can be drawn from the results of case series.
Cohort Study
A cohort study is a study in which patients with and without a risk factor (exposed and unexposed) or who receive two alternative treatments are compared to see if the groups differ with respect to one or more outcomes. So in Fig. 20.1, the direction of investigation is from the left side of the diagram toward the right (from exposure to disease). The groups are defined by the presence of the risk factor. This type of study is helpful for looking at any number of outcomes that result from a single risk factor; for example, eyelid height symmetry and surgical complications (the outcomes) can be compared between patients undergoing levator resection versus Fasanella-Servat procedure (the exposure or risk factor groups). The presence and strength of an association can be described, and it is possible to control for confounding variables (see confounding below) but only those factors of which you are aware and for which you collected data. When conducted prospectively, these studies take time and can be expensive to complete. Note that the comparison group in a cohort study may be referred to as a “control group,” but this terminology is not the same as “controls” in a case-control study.
Case-Control Study
A case-control study is the reverse of a cohort study. The study groups are defined by the outcome (diseased or not diseased), not by the exposure. The groups are then compared for the presence of one or more risk factors. For example, patients with thyroid eye disease can be grouped into those who did (the cases) or did not (the controls) develop an optic neuropathy, and then the two groups can be compared to see if one group had more smokers in it than the other group. In Fig. 20.1, the direction of investigation is from the top row toward the bottom (from disease to exposure). As with a cohort study, the presence and strength of associations can be described, but they are vulnerable to specific types of bias and must be designed carefully, a discussion of which is beyond the scope of this chapter. But a case-control study is very helpful for evaluating multiple risk factors, particularly for rare diseases or outcomes, which would be inefficient to study with a cohort study. Note that the “controls” in a case-control study are the subjects who do not have the outcome. A common mistake by authors is describing their study as a case-control study because of the presence of a “control group” of patients who did not receive the medication being studied, even though they are comparing outcomes between two groups that have been defined by which treatment was received (the “medication” group and the “control” group). Such studies are in fact cohort studies, and the “controls” are study subjects who do not have the exposure. In a case-control study, “controls” refer to the study subjects who do not have the disease.
Randomized Controlled (Clinical) Trial (RCT)
An RCT or an experimental study is similar to a cohort study, as the groups are defined according to whether the subjects have a specific risk factor or received a specific treatment, but the assignment to each group is determined by the investigator, who, in the case of an RCT, randomly assigns each subject to a treatment group. The power of the study design lays in the randomization process, which is the only way to potentially control for not only known confounders but also for unknown confounding factors (see below). Key point: An RCT is logistically complicated to execute but provides the strongest evidence of an association and causality.
Prospective Versus Retrospective
The terms prospective and retrospective refer to the manner in which the data were collected, not to the type of study. For example, a cohort study can be conducted prospectively or retrospectively. Prospective data collection involves collecting data forward in time, as the events are occurring, which allows the investigators to take measures to maximize the reliability and completeness of the data. Retrospective data collection involves collecting data backward in time, after the events have already occurred, which has the advantage of efficiency. Key point: Retrospective data collection in and of itself is not a study limitation. This error is very commonly made by peer reviewers and authors. Data collected retrospectively are subject to various types of bias resulting from inconsistent and missing measurements, but even when such bias is present in a study, it may be irrelevant with regard to the strength of the study conclusions, depending upon the study question (see Bias below).
Blinding (Masking)
A blinded study is one in which the subjects and/or investigator are not aware of the group assignment of the subjects. In ophthalmology, we prefer to use the term “masked,” for obvious reasons. Masking group assignment helps to guard against bias resulting from changes in the behavior of the subjects that influence the study outcomes or bias in the manner in which outcome measurements are made by the study investigators. Randomization and masking are not the same thing. Randomization refers to the process by which subjects are assigned to a study group. Masking refers to whether the subjects or investigators know that assignment.
Levels of Evidence
When evaluating the strength of the evidence available to answer your question, the first step is to consider the study design. The following hierarchy represents the strength of evidence from lowest to highest.
Lowest
- 1.
Anecdotal experience
- 2.
Expert opinion/consensus
- 1.
Non-comparative studies
- 3.
Case report
- 4.
Case series
- 3.
Comparative studies
- 5.
Case-control studies
- 6.
Cohort studies
- 7.
Randomized controlled (clinical) trial
- 8.
Meta-analysis of randomized trials
- 5.
Highest
Subjects
Clearly define the inclusion and exclusion criteria for eligibility for enrollment in the study, by formulating a clear study question. Match the study subjects to the question you are posing. Specify characteristics such as age, study period, diagnosis, treatment received, and minimal follow-up length. Consider the characteristics necessary to assign a subject to an exposure or outcome group, the availability of data in the medical record, sample size requirements, and potential confounders. One method for dealing with confounders is to restrict enrollment to subjects that do, or do not, possess the confounding factor. For example, if studying the worsening of thyroid eye disease by alcohol use, smoking could be a confounding factor, because smoking is associated with both alcohol use and worsening of thyroid eye disease, so studying only patients who do not smoke or studying only patients who do smoke, and excluding the other group, would remove the potential for smoking to be a confounding factor. However, restricting the study sample in this manner could make the results less generalizable to other groups of patients. In this example, an alternative approach would be to not exclude based upon smoking status but rather control for confounding analysis (see below).
Sample Size
The sample size requirement for your study depends upon your study question. It is driven by statistical considerations. The sample size will increase with increasing power desired to detect a difference between groups, decreasing difference in the outcome being measured between groups (20% difference in surgical complications versus 10% difference), increasingly stringent definition of statistical significance (0.1 versus 0.05 versus 0.01), and increasing variability in the factor being measured among the study subjects. A biostatistician can help you to perform an appropriate sample size calculation.
Key point: Having a specific sample size requirement is very important for ensuring that you will be able to answer your study question with the study you are proposing to do. Without one, you may find out later that your non-statistically significant result is not interpretable, because you did not have enough statistical power to answer the study question. Sometimes, you need to use a “convenience sample” of all available patients with a given disease or undergoing a specific procedure, because the clinical volume is simply limited. In such cases, the study question may need to be modified into a question that is answerable with the data available.
Data Collection
Decisions about which data to collect are driven first and foremost by your study question and analysis plan. Ensure data are collected for exposure/ treatment group assignment, primary outcome measures, secondary outcome measures, and potential confounding variables (see Confounding below). When defining these variables, think critically about what data are recorded consistently in the medical record and measured in a reliable fashion. For example, if postoperative lagophthalmos is an outcome of interest, but it is not measured clinically in a consistent manner, using millimeters of lagophthalmos as an outcome variable may not be practical, whereas corneal exposure (yes or no) may be a more reliable and still clinically meaningful measure of the degree of lagophthalmos. Generally, good study planning will ensure that all required data variables are collected without collecting data that is never used. Resist the urge to collect as many variables as possible “just in case” they are needed, as this approach is inefficient and often results in wasted effort.
Key point: Categorize and code variables using numbers rather than letters, because numbers are analyzable. For example, 1 = female, 2 = male; 1 = levator resection, 2 = frontalis sling with autologous fascia lata, etc. As you create your data spreadsheet, always simultaneously keep an additional spreadsheet to serve as a data dictionary, in which the first column contains the variable name, and the second column contains the coding scheme for the variable. One approach to organizing a data file in Microsoft Excel or Google Sheets that facilitates analysis by a biostatistician, who will be using a common statistical software package for the analysis, is to have one sheet organized by “subject,” containing variables (one per column) that occur only once (demographics, baseline risk factors, etc.) for each subject, with one row per subject, and a second sheet organized by “visit,” containing variables that are unique to each clinical evaluation, with one row per office visit. If only baseline and latest follow-up measures are being collected, rather than numerous visits, then a separate “visit” sheet may not be necessary.
Electronic Medical Record (EMR) Data
The use of EMR systems presents a tremendous opportunity to answer research questions faster, with much larger amounts of data and greater numbers of subjects. Identification of subjects can be accelerated by using billing codes and setting inclusion and exclusion criteria that are defined based upon readily accessible variables from the EMR. However, to use this approach, you must plan ahead and consistently code each visit with all relevant codes, so that subjects are not missed when you eventually undertake a research study. EMR exports can be used to generate large data spreadsheets or comma-separated-values files, which are importable into spreadsheets. These data can then can be manipulated and analyzed.
Generally, EMR-generated datasets will require varying amounts of “augmentation” through manual data collection, as well as manual interpretation and coding of the data into analyzable, number-based datasets. The quantity of manual “augmentation” can range from a lot, in which case EMR-generated files still offer a very efficient data abstraction mechanism; to a little, in which case EMR greatly accelerates the project and multiplies the sample size; to none, which is an ideal. How much “augmentation” is necessary depends upon how data are recorded by the clinicians in the medical record, how data are stored and provided by the EMR, and of course the study question itself. For example, a research question involving only refraction data may require no manual data collection or coding, if the data are stored in the EMR separately for each eye; the refractions are broken down by sphere, cylinder, and axis; and these fields are used consistently by the clinicians performing the refractions. Under these circumstances, a dataset can be generated, and relatively simple statistical programming can be done to manipulate the data as necessary to answer the study question, without any manual manipulation or augmentation of the dataset. In contrast, studies that require analysis of radiology imaging reports will require the investigator to manually read and code those reports, though the EMR can rapidly generate a file of the reports. Similarly, strabismus examination documentation varies widely among pediatric ophthalmologists, with varying abbreviations and combinations of words (exotropia or XT) mixed with magnitudes (35 prism diopters) and control descriptors (poorly controlled) in single or multiple fields in the EMR, requiring an investigator to manually recode the EMR-generated dataset of strabismus measurements into discrete separate fields and codes or requiring a biostatistician to write complex coding algorithms to manipulate the data into an analyzable form. An intermediate example is a study of the prevalence of retinal hemorrhages among otherwise healthy children [1]. Such an uncommon finding can efficiently be studied using EMR data, because an EMR dataset can rapidly be searched by sorting a spreadsheet by fundus examination fields (disc, macula, vessels, periphery), permitting rapid identification of the very few children with this rare retinal finding.
Key point: The ability to eventually access large amounts of data rapidly for research purposes depends upon you adopting a consistent, standardized way of recording data in the EMR in your daily clinical practice. In EMR-based research, trade-offs can be made between increasing the efficiency of the study (i.e., less manual data collection and coding) and not taking into account some variables that were not consistently coded or recorded in the medical record. The importance of such limitations in the EMR naturally depends upon the study question, but such limitations can be minimized by standardizing your daily documentation. Think about how you or your colleagues code your visits for billing, record physical examination findings, and document assessments and plans. You can standardize your practices and facilitate EMR research by entering data as numbers or standard codes as much as possible; using predesigned or custom-designed data fields; working with your EMR provider to see how data can be recorded in discrete variables rather than in free text fields, which require excessive manipulation for research use; using diagnostic billing codes in a complete, consistent, and thorough way; and generally anticipating that you may eventually want to answer quality improvement or research questions in the future.
Data Analysis
As discussed above, a basic way to conceptualize the purpose of a clinical research study is the determination of the presence and strength of an association between an exposure and an outcome. For example, the exposure may be a specific surgical technique, compared to another technique, and the outcome may be a postoperative complication. Therefore, it is important to clearly define how groups are defined (exposed/unexposed, outcome present/outcome absent) for the analysis. These definitions and the analysis are determined by the study question, as are all aspects of the study design.
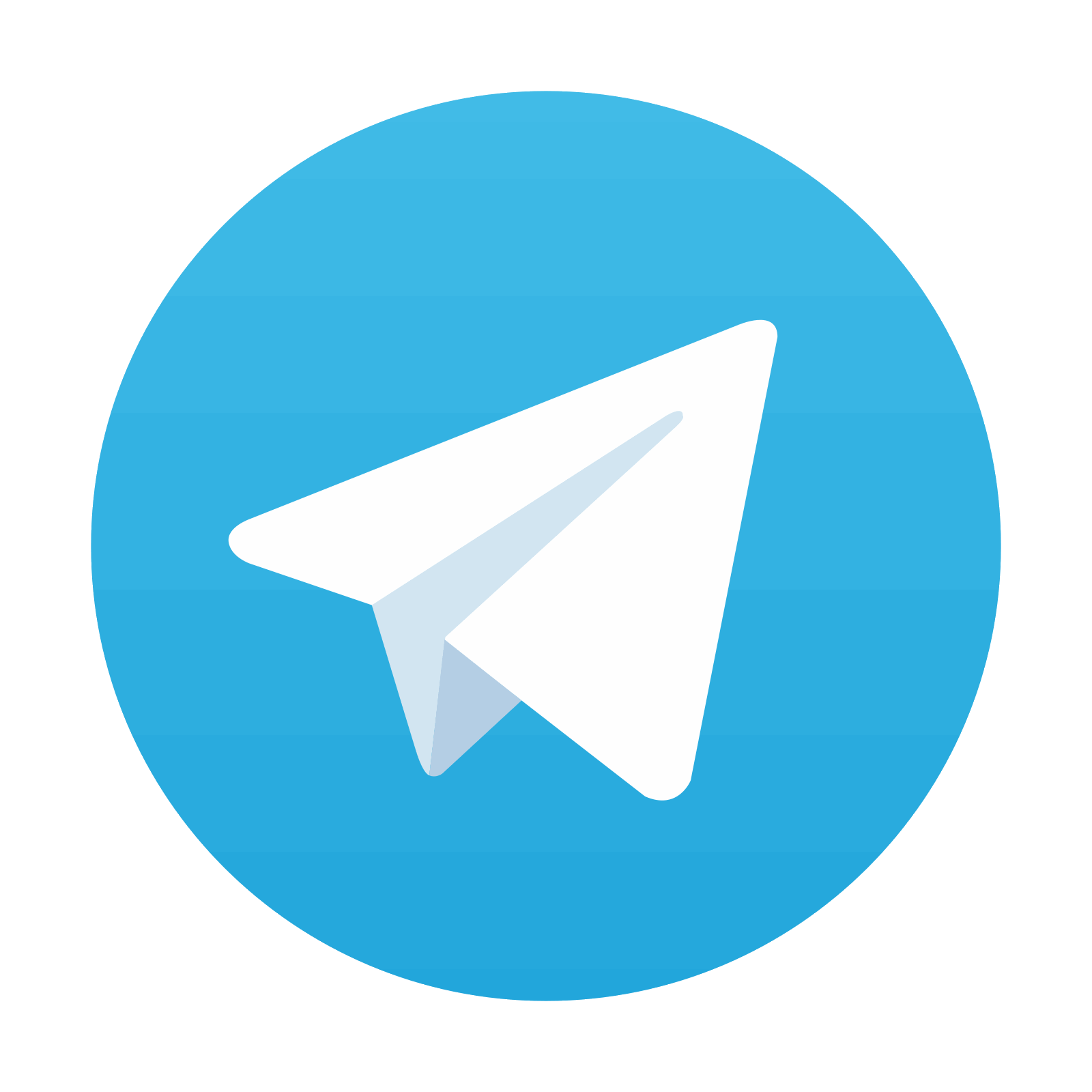
Stay updated, free articles. Join our Telegram channel

Full access? Get Clinical Tree
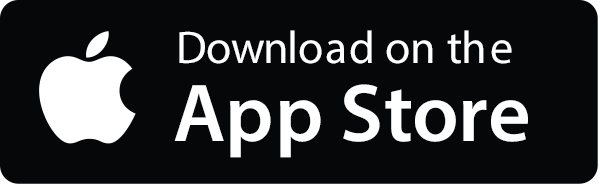
