Purpose
To investigate flow characteristics of the choriocapillaris using optical coherence tomography angiography.
Design
Retrospective observational case series.
Methods
Visualization of flow in individual choriocapillary vessels is below the current resolution limit of optical coherence tomography angiography instruments, but areas of absent flow signal, called flow voids, are resolvable. The central macula was imaged with the Optovue RTVue XR Avanti using a 10-μm slab thickness in 104 eyes of 80 patients who ranged in age from 24 to 99 years of age. Automatic local thresholding of the resultant raw data with the Phansalkar method was analyzed with generalized estimating equations.
Results
The distribution of flow voids vs size of the voids was highly skewed. The data showed a linear log-log plot and goodness-of-fit methods showed the data followed a power law distribution over the relevant range. A slope intercept relationship was also evaluated for the log transform and significant predictors for variables included age, hypertension, pseudodrusen, and the presence of late age-related macular degeneration (AMD) in the fellow eye.
Conclusions
The pattern of flow voids forms a scale invariant pattern in the choriocapillaris starting at a size much smaller than a choroidal lobule. Age and hypertension affect the choriocapillaris, a flat layer of capillaries that may serve as an observable surrogate for the neural or systemic microvasculature. Significant alterations detectable in the flow pattern in eyes with pseudodrusen and in eyes with late AMD in the fellow eye offer diagnostic possibilities and impact theories of disease pathogenesis.
Imaging the choriocapillaris in vivo is difficult because of light scattering within overlying tissue, particularly the retinal pigment epithelium (RPE). Although the choriocapillaris is composed of relatively large-diameter capillaries, they are interconnected in a densely packed arrangement with very small intercapillary pillars. The size of the separation is often less than the lateral resolution afforded by dye-based angiography. Fluorescein readily leaks from the choriocapillaris, and would obscure visualization of the choriocapillaris. Although indocyanine green shows less leakage, it also leaks from the choriocapillaris and stains the Bruch membrane and the choroidal stroma. Optical coherence tomography (OCT) has high axial resolution, but the illumination spot size is typically so large that the lateral resolution is insufficient to visualize the choriocapillaris clearly in the posterior pole. OCT angiography detects a flow signal by evaluating change in reflectivity from sequentially acquired images. While OCT angiography suffers the lateral resolution problems of structural OCT, it also retains the depth-resolved nature of the underlying structural OCT and blood flow selectively can be visualized in specified layers.
When the innermost thickness of the choroid is sampled with OCT angiography, a granular image is obtained that is suggestive of the choriocapillaris. The resultant image shows a pattern of bright areas, thought to represent flow. The granularity of the choriocapillaris image is created not only by the bright regions, but also by many small dark regions in the image. The anatomic structure of the choriocapillaris does not appear to explain the dark areas, but there has to be a relative decrease in local flow signal for the dark areas to be imaged. As such, these small areas are called flow voids. Using image processing software it is possible to measure the size and number of the flow voids. Evaluation of the distribution of the number of flow voids at any given size revealed a potential mathematical relationship that could be used to grade choriocapillaris flow. This paper discusses the development of a method to evaluate choriocapillaris flow, particularly the pattern of regional perfusion, the analysis of the flow voids imaged, and the relationship of a mathematical description to systemic and ocular characteristics. This novel noninvasive approach may have utility in evaluating ocular disease, and possibly may be a method to evaluate systemic microvascular health.
Methods
This retrospective study was approved by the Western Institutional Review Board and complied with the Health Insurance Portability and Accountability Act of 1996. Visual acuity was measured with habitual refraction and converted to logMAR prior to analysis. Late age-related macular degeneration (AMD) was considered to be either choroidal neovascularization or center-involving geographic atrophy. Patients were considered to have a diagnosis of hypertension if they reported having a physician diagnosis of high blood pressure. This was cross-validated with the patients in reviewing their medication list.
Optical Coherence Tomography Angiography
The instrument used for OCT angiography images was the Optovue RTVue XR Avanti (Optovue, Inc, Freemont, California, USA) to obtain amplitude decorrelation angiography images. This instrument has an A-scan rate of 70 000 scans per second, using a light source centered on 840 nm and a bandwidth of 45 nm. Each OCTA 3 × 3-mm volume contains 304 × 304 A-scans with 2 consecutive B-scans captured at each fixed position before proceeding to the next sampling location. Split-spectrum amplitude-decorrelation angiography was used to extract the OCT angiography (OCTA) information. Each OCTA volume is acquired in about 3 seconds. Two orthogonal OCTA volumes were acquired in order to perform motion correction while minimizing motion artifacts arising from microsaccades and changes in fixation. All subjects had pupillary dilation. The choriocapillaris later was sampled as a slab 10 μm thick starting 31 μm posterior to the retinal pigment epithelium–Bruch membrane complex segmentation. As mentioned in a previous publication, this area seems to be somewhat posterior to the actual location of the choriocapillaris. This image was compared with an image obtained with the same slab thickness but starting at 34 μm posterior to the RPE–Bruch membrane complex. The scan with the least projection artifact was selected.
The resultant image was exported as what the Optovue software calls a raw image. Inspection of the histograms of the raw image output typically shows a Gaussian curve of grayscale values for imaged patients over a wide range of ages and pathologies, raising the possibility that the raw images actually were scaled to fit into the 255 grayscale values potentially displayable.
Analysis of the Choriocapillaris Images
Thresholding and void counting
The OCT angiography images of the choriocapillaris were analyzed for shadowing caused by overlying retinal vessels. These regions were masked so that the shadows would not affect subsequent analysis. Drusen are associated with histologic evidence of choriocapillaris ghost vessels, which are considered to be nonfunctioning capillaries. However, drusen can cause shadowing and a decreased visualization of the underlying choriocapillaris. Because there is no proven way to differentiate a flow void from shadowing, eyes with drusen causing shadowing (generally those with drusen greater than 40 μm in height) were excluded. Instead of analyzing the choriocapillaris for where flow signal could be detected, the converse was done. Areas where there was a lack of flow information—flow voids—were identified by thresholding and those areas were measured. By looking at the voids the pattern of the flow structure could also be evaluated.
The images were then imported into FIJI (an expanded version of ImageJ version 1.51a, available at fiji.sc , free of charge). Automatic local thresholding was done with the Phansalkar method using a radius of 15 pixels. Adaptive local thresholding was done to obviate small regional variations in image brightness and the Phansalkar method was used because it was designed to select darker regions in potentially low-contrast images. The thresholded images were then analyzed with the “Analyze Particles” command, which measured and counted all thresholded areas greater than or equal to 1 pixel where there was a lack of flow information.
These results are saved as a comma-delimited file, which can be imported into Excel (Microsoft Office 2010; Microsoft Inc, Redmond, Washington, USA). The data were logarithmically binned and plotted as log number vs log size. These data followed a linear association, which is a lower level of evidence of a power law relationship because under some circumstances the results can be biased and because there is no indication how well the data are fitted by a power law equation. The models plotted in the spreadsheet were fitted with a trendline that followed the form log (number) = m log (size) + b , a simple slope intercept equation, according to the logarithmically binned data. As such, the variables m and b can be calculated and compared between subjects.
Python and power law
Python is a high-level open source programming language that has the support of numerous modules. The Python distribution used was Anaconda Python 3.5 (available free of charge from Continuum Analytics, Inc, Austin, Texas, USA; https://www.continuum.io/downloads ). Powerlaw 1.4.1 (available free of charge, https://pypi.python.org/pypi/powerlaw ), an implementation of maximum-likelihood fitting methods with goodness-of-fit tests, was used to evaluate data and in particular to test the probability that the observed distribution represented a power law distribution as opposed to the frequently more common lognormal distribution. Given the finite range of data possible because of the 304 × 304 pixel size of the Optovue angiographic image, one would expect an upper bounding effect. As such, there was no expectation to be able to differentiate power law from an exponentially truncated power law distribution, a discrimination that has no useful benefit in the range of the data available. The Python program using Powerlaw 1.4.1 was also used to plot the probability distribution function of the data.
Inclusion and Exclusion
The inclusion criteria were scan signal 55 or greater, and the exclusionary criteria included gross eye movements; ocular disease that could affect scan quality, visual acuity, or ability to fixate; history of retinal or macular surgery; high myopia, defined as a refractive error more than 6 diopters of myopia; any macular condition including large drusen or pigmentation that would cause shadowing of the choriocapillaris; history of central serous chorioretinopathy; and previous ocular laser photocoagulation or photodynamic therapy, previous retinal vascular occlusion, choroidal neovascularization, and center-involving geographic atrophy.
Statistical Analysis
The data were analyzed with frequency and descriptive statistics. Generalized estimating equation (GEE) modeling was used to analyze the parameters of the slope intercept equation. The proportion of the pixels showing lack of flow information accounted for by flow voids greater than or equal to 10 000 μm 2 (abbreviated FV10000) was calculated, as were the predictors of these proportions by GEE. For all tests, P < .05 was considered significant. The statistical analyses were performed with IBM-SPSS software version 21.0 (IBM-SPSS Inc, Chicago, Illinois, USA).
Results
There were 104 eyes of 80 patients who ranged in age from 24 to 99 years (median 71, interquartile range [IQR] 56.5–77 years). There were 45 female patients (56.3%). Of the 80 patients, 28 (35%) had a diagnosis of hypertension and 11 eyes of 104 (10.6%) had pseudodrusen. The median visual acuity was 20/25 (logMAR 0.0969, IQR 20/20 to 20/30).
A visual comparison of the texture of the choriocapillaris flow signal across various ages is shown in Figure 1 . A comparison of the flow signal from a 75-year-old subject with a history of choroidal neovascularization is shown in Figure 2 . The results of the automatic local thresholding are shown by comparison with the flow voids shown in white. Each of these spots were counted and measured. Figure 3 shows various methods that may be used to visualize the data from the thresholded image from Figure 2 . The top image in Figure 3 shows the number of flow voids within each bin size, as measured in μm 2 . Note the highly skewed distribution, and only the first 30 bins are shown. Given the skewed (non-normal) nature of the distribution, calculation of mean and standard deviation is not possible. Figure 3 (Middle and Bottom) show 2 methods of examining the data using a log-log transformation. Figure 3 , Middle shows the probability for any given flow void size as calculated in the power law package. Figure 3 , Bottom shows the number of flow voids as binned in logarithmically sized bins. The distribution of the size in flow voids ranged over nearly 3 log units in Figure 3 , Bottom. A trend line can be fitted to the data and this trend line has a simple slope intercept formula.
For the group, using the slope intercept equation log (number) = m log (size) + b showed a mean m of −0.63 and the mean of b was 3.91. The proportion of pixels with poor flow accounted by flow voids greater than or equal to 10 000 μm 2 , FV10000, ranged from 0.25 to 0.845 with a mean of 0.436. Using GEE, the predictors of m were age and hypertension ( Table 1 ). The predictors of b were age, hypertension, and a diagnosis of late AMD in the fellow eye ( Table 2 ). The predictors for FV10000 were age, late AMD in the fellow eye, and the presence of pseudodrusen ( Table 3 ). A consolidated summary of the findings in terms of a slope intercept schematic is shown in Figure 4 .
Parameter | Parameter Estimates | |||||
---|---|---|---|---|---|---|
B | Standard Error | 95% Wald Confidence Interval | Hypothesis Test | |||
Lower | Upper | Wald χ 2 | Significance | |||
(Intercept) | −.776 | .0298 | −.835 | −.718 | 676.798 | <.001 |
Age | .002 | .0005 | .001 | .003 | 15.156 | <.001 |
Hypertension | .070 | .0325 | .007 | .134 | 4.694 | .030 |
Parameter | Parameter Estimates | |||||
---|---|---|---|---|---|---|
B | Standard Error | 95% Wald Confidence Interval | Hypothesis Test | |||
Lower | Upper | Wald χ 2 | Significance | |||
(Intercept) | 4.335 | .0764 | 4.185 | 4.484 | 3216.953 | <.001 |
Age | −.005 | .0014 | −.008 | −.002 | 13.782 | <.001 |
Hypertension | −.177 | .0671 | −.309 | −.046 | 7.001 | .008 |
Late AMD Fellow eye | −.167 | .0694 | −.303 | −.031 | 5.791 | .016 |
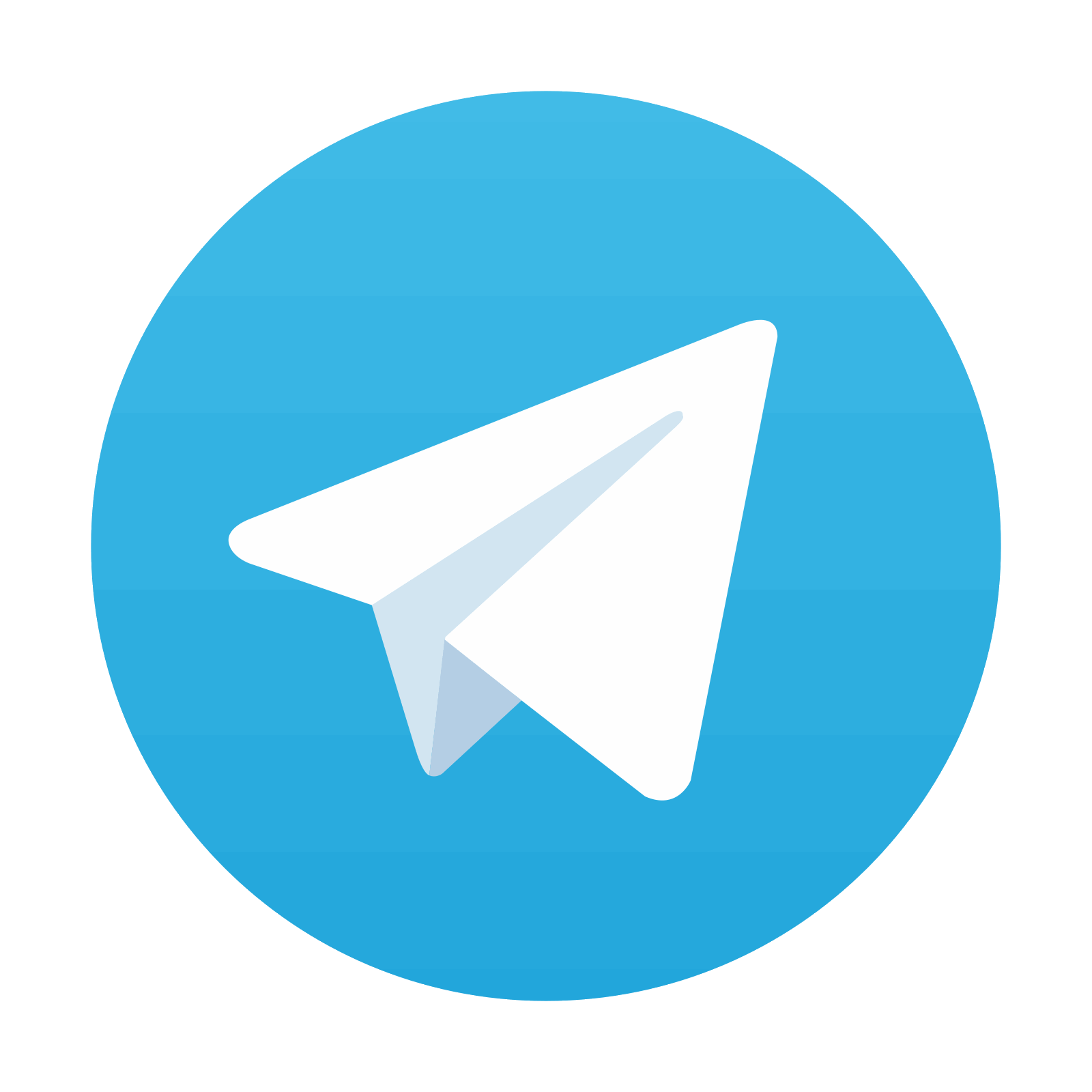
Stay updated, free articles. Join our Telegram channel

Full access? Get Clinical Tree
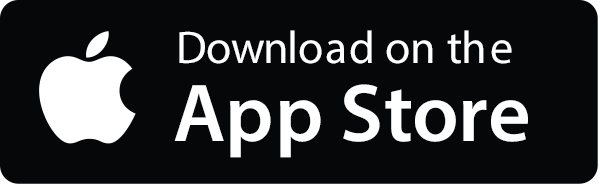

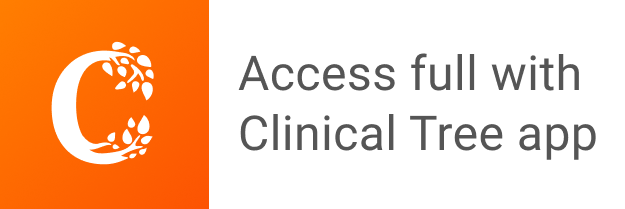