Highlights
- •
No-shows were associated with certain patient and appointment characteristics.
- •
Younger age was associated with a higher likelihood of no-show.
- •
Lower socioeconomic status was associated with a higher likelihood of no-show.
- •
Black and other races were associated with a higher likelihood of no-show.
- •
Longer lead times were associated with a higher likelihood of no-show.
Purpose
This study aimed to identify patient and appointment characteristics associated with no-shows to new patient appointments at a US academic ophthalmology department.
Design
Cross-sectional study.
Methods
This was a study of all adult patients with new patient appointments scheduled with an attending ophthalmologist at Penn State Eye Center between January 1 st and December 31 st of 2019. A multiple logistic regression model was used to assess the association between characteristics and no-show status.
Results
Of 4,628 patients, 759 (16.4%) were no-shows. From the multiple logistic regression model, characteristics associated with no-shows were age (Odds Ratio (OR) for 18-40 years vs. >60 years: 3.41, 95% Confidence Interval (CI) 2.57, 4.51, p <0.001 and OR for 41-60 years vs. >60 years: 2.14, 95% CI 1.67, 2.74, p<0.001), median household income (OR for <$35,667 vs. >$59,445: 1.59, 95% CI 1.08, 2.34, p<0.001), insurance (OR for None vs. Medicare: 6.92, 95% CI 4.41, 10.86, p<0.001 and OR for Medicaid vs. Medicare: 1.54, 95% CI 1.18, 2.01, p=0.002), race (OR for Black vs. White: 2.62, 95% CI 2.00, 3.43, p<0.001 and OR for Other vs. White: 2.02, 95% CI 1.58, 2.59, p<0.001), and commute distance (OR for 5-10 mi vs. ≤5 mi: 1.73, 95% CI 1.17, 2.55, p=0.006). Appointments with longer lead times and scheduled with glaucoma or retina specialists were also significantly associated with greater no-shows.
Conclusion
Certain patient and appointment characteristics were associated with no-show status. These findings may assist in the development of targeted interventions at the patient, practice, and health system levels to improve appointment attendance.
N onattendance to appointments, or no-shows, are a problem commonly faced by clinical practices in the United States, with no-show rates ranging from 5% to 55%. No-shows negatively impact health care delivery and result in negative individual and societal consequences, including poor health outcomes for the no-show patient, lost opportunity and longer wait times for other patients, and wasted resources, decreased revenue, and decreased provider productivity for the clinical practice. No-shows negatively impact the field of ophthalmology, a field characterized by high-volume clinical visits and surgical procedures. No-shows are a significant concern particularly for academic medical centers, which not only provide a disproportionate share of care to vulnerable patients but also handle patients with more complex medical conditions that require more resources and coordination of care. ,
Prior studies in various fields of medicine have found that younger age, lower socioeconomic status, longer commute distance, Medicaid insurance, Black or other nonwhite race, and longer appointment lead times were associated with a higher likelihood of nonattendance. Studies exploring nonattendance within ophthalmology are few, , , , and of those, only 1 study was conducted at an academic center in the United States, which explored the association of no-shows with appointment lead time and found that the greater the time in advance an appointment is scheduled, the higher the no-show rate. The present study aimed to identify patient and appointment characteristics associated with new patient appointment no-shows at an academic ophthalmology department in the United States.
METHODS
This was a retrospective cross-sectional study with approval obtained from the Institutional Review Board at the Penn State Hershey Medical Center. All methods described herein adhered strictly to the tenets of Declaration of Helsinki and Health Insurance Portability and Accountability Act regulations. A retrospective enterprise information management query was conducted on all new patient appointments of patients age 18 years and older who had a scheduled appointment with an attending ophthalmologist at Penn State Eye Center located in Hershey, Pennsylvania, from January 1, 2019, to December 31, 2019. Appointment status was categorized as “show” or “no-show.” A “show” appointment was defined as any scheduled appointment that was attended by the patient. A “no-show” appointment was defined as any scheduled appointment that was not attended by the patient and was not cancelled by the clinic or the patient before the time of the appointment.
As new patient appointments could include appointments of patients who were new to a particular provider but seen previously by other providers within the department, only the patient data and appointment status of the first scheduled new patient appointment within the study period were included in the analysis to ensure that patient data were counted and analyzed only once for each patient included in this study.
Variables
Individual characteristics
Individual characteristic variables included age, sex, and race. Age was categorized as 18–40, 41–60, and >60 years old. Race was determined through self-identification as White, Black/African American, Asian, American Indian/Alaskan Native, Other, 2 or more races, Unknown, or Declined to answer, and categorized as White, Black, Asian, Other, or Unknown.
Socioeconomic characteristics
Patients’ ZIP (Zone Improvement Plan) codes were used as an estimate of the patients’ median household income, which was determined by linking publicly available data from the US Census 2013–2017 American Community Survey, a method previously described in other studies. , Median household income was categorized using government established cut points: <60%, 60%–100%, or >100% of the state-wide median household income, which was $59,445 for the state of Pennsylvania. Primary and secondary insurance information were categorized as Medicare, Medicaid, Commercial, Military, None, Other, or Unknown.
Contextual/health system characteristics
Commute distance was estimated by a patient’s ZIP code and the location of Penn State Eye Center in Google Maps and categorized as ≤5 miles, >5–≤10 miles, >10–≤ 30 miles, or >30 miles. Lead time was defined as the time between the date the appointment was scheduled and the date of the appointment. The scheduled appointment time was used to determine the day of the week, time of day, and season of the year, which was categorized as spring (March–May), summer (June–August), fall (September–November), or winter (December–February). Appointments were categorized by the subspecialty of the provider the patient was scheduled to see. During the study period, the department had 3 cornea specialists, 3 glaucoma specialists, 1 neuro-ophthalmologist, 2 oculoplastics specialists, 2 strabismus specialists, and 5 retina specialists.
Statistical Analysis
Descriptive statistics for categorical variables are presented as frequencies, n (%). χ 2 tests were used to assess the bivariate association of each of the previously described individual patient, socioeconomic, and contextual/health system characteristic with appointment no-show status. Additionally, logistic regression was used to assess the bivariate association of each individual patient, socioeconomic and contextual/health system characteristic with appointment no-show status in order to obtain unadjusted odds ratios (ORs) and 95% confidence intervals (CIs). A multivariate model was constructed using multiple logistic regression to assess the associations of an individual patient, socioeconomic, and contextual/health system characteristic with appointment no-show status while adjusting for the other factors in the model to provide adjusted ORs. For the multiple logistic regression model, the independent variables (eg, age, sex, race, and so forth) were first assessed for collinearity prior to inclusion in the final model. Multiple logistic regression model fit was determined by examining the Hosmer-Lemeshow goodness-of-fit test and the C-statistic (ie, the area under the receiver operating characteristic curve). Secondary insurance was not included in the multivariate analyses but was analyzed separately. Among patients with Medicare listed as their primary insurance, a χ 2 test and bivariate logistic regression analysis were conducted to examine the association between secondary insurance and no-show status. Listing Medicaid as either primary or secondary insurances also was analyzed for its association with no-show status. All hypothesis tests were 1-sided, and statistical analysis was performed using SAS version 9.4 software (SAS Institute, Inc., Cary, North Carolina, USA).
RESULTS
During the 1-year time period, a total of 4,628 unique patients and their associated new patient appointments met the inclusion criteria. The overall no-show rate in this study was 16.4% but varied across the individual characteristic variables ( Table 1 ).
Variables | Overall n (%) | Show n (%) | No-Show n (%) | P Value a |
---|---|---|---|---|
All patients | 4,628 (100.0) | 3,869 (83.6) | 759 (16.4) | |
Individual characteristics | ||||
Age | <.001 | |||
18–40 y | 839 (18.1) | 582 (69.4) | 257 (30.6) | |
41–60 y | 1,455 (31.4) | 1,156 (79.5) | 299 (20.5) | |
>60 y | 2,334 (50.4) | 2,131 (91.3) | 203 (8.7) | |
Sex | .909 | |||
Females | 2,601 (56.2) | 2,173 (83.5) | 428 (16.5) | |
Males | 2,027 (43.8) | 1,696 (83.7) | 331 (16.3) | |
Race | <.001 | |||
White | 3,302 (71.4) | 2,951 (89.4) | 351 (10.6) | |
Black | 419 (9.1) | 268 (64.0) | 151 (36.0) | |
Asian | 147 (3.2) | 121 (82.3) | 26 (17.7) | |
Other b | 539 (11.6) | 387 (71.8) | 152 (28.2) | |
Unknown c | 221 (4.8) | 142 (64.3) | 79 (35.7) | |
Socioeconomic characteristics | ||||
Median household income d | <.001 | |||
<$35,667 | 194 (4.2) | 127 (65.5) | 67 (34.5) | |
$35,667–$59,445 | 1,566 (34.1) | 1,223 (78.1) | 343 (21.9) | |
>$59,445 | 2,838 (61.7) | 2,493 (87.8) | 345 (12.2) | |
Primary Insurance | <.001 | |||
Medicare | 1,935 (41.8) | 1,736 (89.7) | 199 (10.3) | |
Medicaid | 869 (18.8) | 592 (68.1) | 277 (31.9) | |
Commercial | 1,527 (33.0) | 1,335 (87.4) | 192 (12.6) | |
Military | 125 (2.7) | 118 (94.4) | 7 (5.6) | |
None | 125 (2.7) | 44 (35.2) | 81 (64.8) | |
Other | 43 (0.9) | 41 (95.3) | 2 (4.7) | |
Unknown | 4 (0.1) | 3 (75.0) | 1 (25.0) | |
Contextual/Health systems characteristics | ||||
Commute distance | <.001 | |||
≤5 miles | 516 (11.2) | 467 (90.5) | 49 (9.5) | |
>5–≤10 miles | 718 (15.5) | 585 (81.5) | 133 (18.5) | |
>10–≤ 30 miles | 2,144 (46.3) | 1,768 (82.5) | 376 (17.5) | |
>30 miles | 1,250 (27.0) | 1,049 (83.9) | 201 (16.1) | |
Lead time | <.001 | |||
0–14 d | 862 (18.6) | 806 (93.5) | 56 (6.5) | |
15–30 d | 780 (16.9) | 692 (88.7) | 88 (11.3) | |
31–90 d | 1,888 (40.8) | 1,522 (80.6) | 366 (19.4) | |
>90 d | 1,098 (23.7) | 849 (77.3) | 249 (22.7) | |
Appointment day | .012 | |||
Monday | 1,227 (26.5) | 1,018 (83.0) | 209 (17.0) | |
Tuesday | 1,000 (21.6) | 810 (81.0) | 190 (19.0) | |
Wednesday | 836 (18.1) | 696 (83.3) | 140 (16.8) | |
Thursday | 1,022 (22.1) | 870 (85.1) | 152 (14.9) | |
Friday | 543 (11.7) | 475 (87.5) | 68 (12.5) | |
Appointment time | <.001 | |||
7:00 AM–11:59 AM | 3,081 (66.6) | 2,536 (82.3) | 545 (17.7) | |
12:00 PM–5:00 PM | 1,547 (33.4) | 1,333 (86.2) | 214 (13.8) | |
Appointment season | .127 | |||
Spring | 1,191 (25.7) | 1,020 (85.6) | 171 (14.4) | |
Summer | 1,103 (23.8) | 916 (83.0) | 187 (17.0) | |
Fall | 1,205 (26.0) | 1,006 (83.5) | 199 (16.5) | |
Winter | 1,129 (24.4) | 927 (82.1) | 202 (17.9) | |
Subspecialty | <.001 | |||
Cornea | 863 (18.7) | 794 (92.0) | 69 (8.0) | |
Glaucoma | 708 (15.3) | 588 (83.1) | 120 (16.9) | |
Neuro-ophthalmology | 638 (13.8) | 556 (87.1) | 82 (12.9) | |
Oculoplastics | 874 (18.9) | 745 (85.2) | 129 (14.8) | |
Strabismus | 75 (1.6) | 58 (77.3) | 17 (22.7) | |
Retina | 1,470 (31.8) | 1,128 (76.7) | 342 (23.3) |
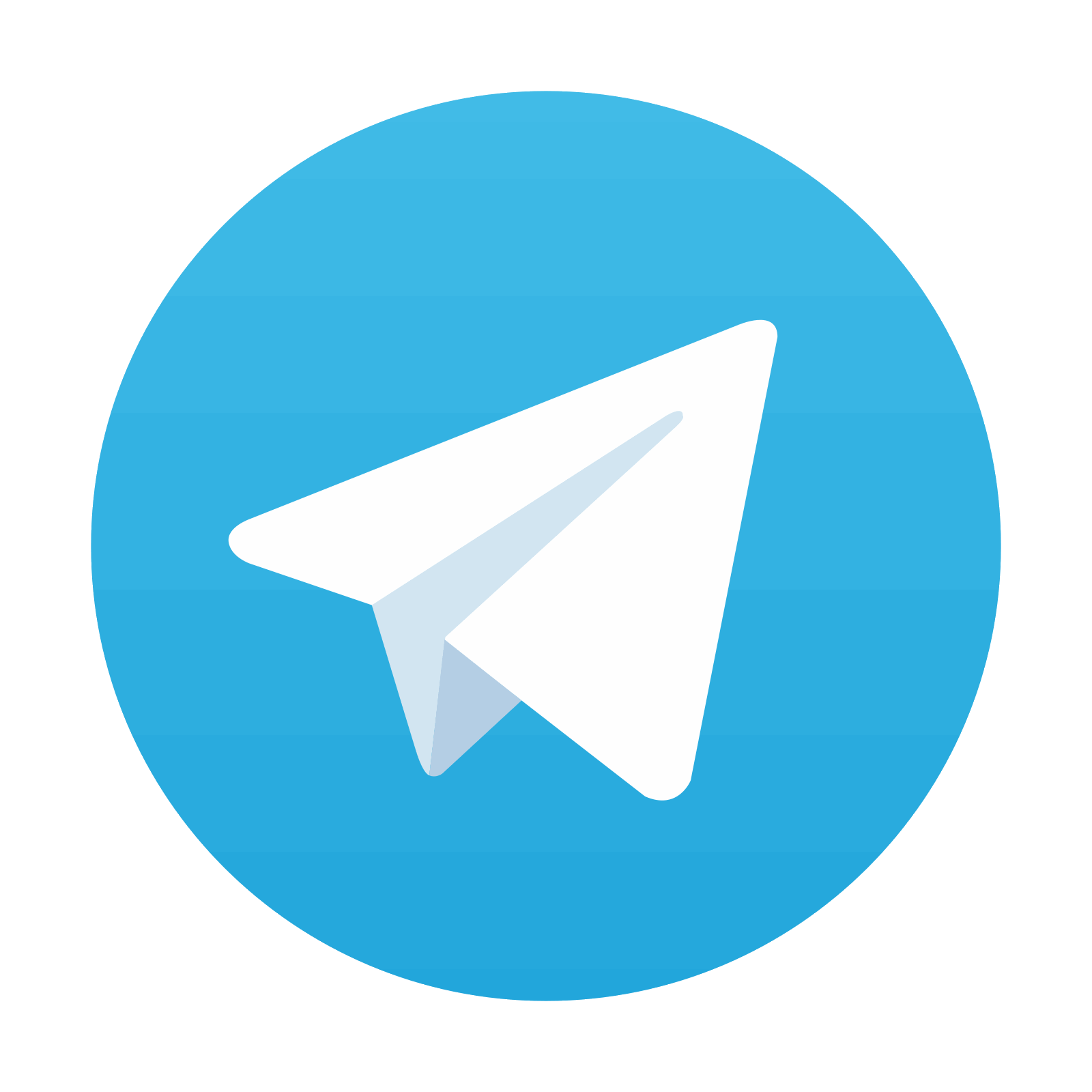
Stay updated, free articles. Join our Telegram channel

Full access? Get Clinical Tree
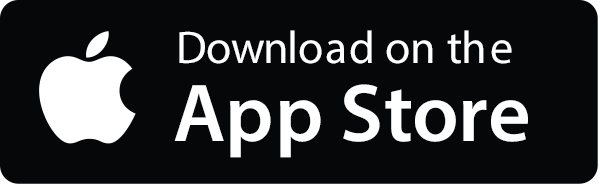

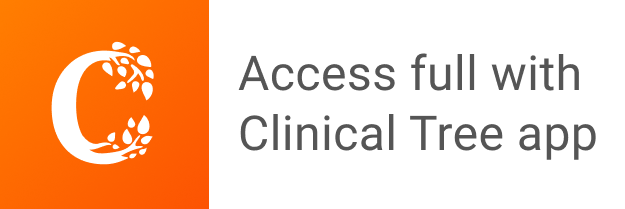