Visual representation of three sine waves of different frequency being added to create a complex waveform
There are also aspects of voice production that are nonlinear, including the subglottal pressure-airflow relationship, vocal fold collisions, and stress-strain characteristics of laryngeal tissue [12]. This means that under certain conditions, such as high subglottal pressure and asymmetric tension or mass, vocal fold vibration may become aperiodic, producing chaotic acoustic signals [13]. Chaos, in this context, is a phenomenon that describes seemingly random behavior that is both nonlinear and deterministic. This is in contrast to white noise that is stochastic [12] (Fig. 8.1).
Voice Signal Types

Four spectrograms representative of the four voice types. (a) Type 1 voice with minimal subharmonics. (b) Type 2 voice with predominately periodic waves with subharmonics. (c) Type 3 voice with aperiodic waves and subharmonics. (d) Type 4 voice with no discernable periodicity and random noise
Parameters
GRBAS and CAPE-V
GRBAS is an auditory-perceptual metric that is used to subjectively assess voice based on five characteristics: grade, roughness, breathiness, asthenia, and strain. Grade assesses hoarseness and abnormality in the voice. Perceived roughness represents the degree of irregular vocal fold vibration. Breathiness assesses glottic air leakage [15, 16]. Asthenia measures perceived weakness in the voice. Strain is an assessment of perceived vocal hyperfunction. The five components of the GRBAS system are rated individually on a four-point scale, where zero corresponds to normal phonation and three corresponds to severely disordered phonation [17].
The Consensus Auditory-Perceptual Evaluation of Voice (CAPE-V) is another psychoacoustic metric that allows clinicians to assess voice based on overall severity, roughness, breathiness, strain, pitch, and loudness. This system uses a 100-mm-long visual analog scale, where the far left represents normal vocalization and the far right represents severely disordered voice [8, 18]. Both GRBAS and CAPE-V require a trained rater, typically a speech-language pathologist, to make judgements on a subject’s voice. Thus, as mentioned previously, these metrics are subject to the rater’s level of experience.
Both GRBAS and CAPE-V have been used with children to successfully distinguish between healthy and disordered voices and evaluate the outcomes of surgical procedures and voice therapy [19, 20, 21, 22, 23, 24]. The reliability of these measures has also been investigated. In a study of 50 children aged 4–20, the CAPE-V metric was used to assess dysphonia after laryngotracheal reconstruction. Seventeen of the samples were then rerated at a later time. Inter-rater reliability was high for perceptions of breathiness, roughness, pitch, and overall severity with intraclass correlation coefficients (ICC) ranging from 67% to 71%. Perceptions of loudness were less reliable (ICC = 57%). Except for strain, intra-rater reliability was strong for all parameters, with the ICC ranging from 63% to 93% [25]. Other studies in adults and children have also found that strain, measured using CAPE-V or GRBAS, is less reliable [26, 27, 28].
Fundamental Frequency
Fundamental frequency (Fo) is the lowest frequency of a periodic or nearly periodic signal. Within a complex periodic waveform, there exists a period (duration of a single cycle of a periodic signal) that is the smallest overall. This is the fundamental period To. Fo is then defined as 1/To [1]. Fo reflects the frequency of vocal fold vibration; qualitatively, changes in fundamental frequency will result in a change of pitch. This is calculated through a Fourier transform, which is a decomposition of the time-domain signal, in this case an acoustic recording, into its frequency components. Figure 8.1 depicts the different waves that can make up a signal. The frequency of each wave can be plotted along with their relative amplitudes. The lowest of these frequencies is taken as F0.
In a study of 218 healthy children aged 4–17, fundamental frequency was measured from recordings of four CAPE-V sentences. For three of the four sentences, fundamental frequency was found to decrease significantly more rapidly during ages 11–14 for boys, compared to ages 4–11 and 14–17. For girls, fundamental frequency linearly decreased with age. There was no critical age at which frequency dropped more significantly [29].
Jitter and Shimmer
Jitter and shimmer are parameters that track perturbation in the voice. Specifically, jitter is a measure of the change in fundamental frequency from cycle to cycle, and shimmer measures cycle-to-cycle change in amplitude of the signal [1]. Jitter and shimmer have been used extensively in acoustic analysis; however, these methods are less reliable in the analysis of aperiodic voice signals [12, 30, 31, 32].
Signal-to-Noise and Harmonic-to-Noise Ratios
Signal-to-noise ratio (SNR) quantifies how dominant the voice signal is over random noise. Harmonic-to-noise ratio (HNR) is similar to SNR in that it quantifies the dominance of periodic (harmonic) signal elements over noise [33]; however, noise in this case refers to random noise, aperiodic signal elements, and perturbations such as jitter and shimmer [34]. This parameter is helpful for assessing vocal characteristics such as breathiness, which results from turbulent airflow [33]. Both SNR and HNR have been used to successfully analyze healthy and disordered voices in children [35, 33, 36, 37].
Perceptual and signal processing techniques can also be used in combination to provide a comprehensive acoustic analysis of voice. In a study of 39 children aged 7–14 with a diagnosis of bilateral vocal nodules, acoustic analyses were performed to assess progression during voice therapy. Therapy lasted for 8 weeks and consisted of lessons on vocal hygiene and voice abuse reduction, breathing and phonation coordination, and laryngeal massage. Jitter and shimmer improved most significantly post-therapy. HNR was also lower after therapy compared to baseline, but Fo did not change. Perceptual ratings according to the GRBAS system showed that grade, roughness, breathiness, and strain all improved [36].
Correlation Dimension, Lyapunov Exponents, and Kolmogorov Entropy
Nonlinear dynamic methods are useful for analyzing normal and disordered voices, and they provide the advantage of not having to track cycle boundaries or fundamental frequency, which can be difficult for aperiodic voices [38, 39, 40, 41]. Correlation dimension (D2), Lyapunov exponents, and Kolmogorov entropy are nonlinear dynamic methods that have been used extensively in research [12, 13, 30, 42, 43, 44]. D2 measures the number of degrees of freedom that are necessary to describe a dynamic system, with more complex systems having a higher D2 [43]. In the context of voice analysis, D2 objectively describes the degree of periodicity or aperiodicity and chaos in the voice. When D2 does not converge to a finite value, this indicates the presence of a high level of random noise [43]. Lyapunov exponents assess a dynamic system by focusing on two trajectories that are initially nearby and measuring their rate of divergence or convergence over time [44]. For voice samples that are nearly periodic, the signal remains stable, and the value of a Lyapunov exponent remains close to zero; however, chaotic signals have positive Lyapunov exponents [30]. Second-order Kolmogorov entropy (K2) quantifies the rate that information about the system dynamics is lost, with a positive K2 value indicating chaos in the signal [43]. The calculation of Lyapunov exponents, D2, and K2 involves reconstructing the phase space of the corresponding signal, which is used to describe all possible dynamic states of the voice signal over time [30, 45].
Meredith et al. performed nonlinear dynamic acoustic analysis of sustained vowels in 23 dysphonic and 15 healthy children. D2 was higher among dysphonic children, indicating that the voices of dysphonic children require a higher number of degrees of freedom to be fully quantified and are therefore more aperiodic. Additionally, though jitter was higher among dysphonic children, variability was high for both groups [46].
Linear and nonlinear acoustic analyses were also utilized in a study of 111 healthy female and 101 healthy male children aged 6–12. While jitter and shimmer did not vary significantly with age or sex, fundamental frequency and largest Lyapunov exponent were lower in boys and decreased with age. These findings show that the boys’ voices had lower frequency and were more stable than girls’ voices [47]. Higher Lyapunov exponents have also been seen in cleft palate patients with hypernasality compared to cleft palate patients without hypernasality [48].
Formants
Resonance created by vocal tract filtering can be described by formants, which are characterized by a center frequency and bandwidth and are influenced by the length and shape of the vocal tract [49]. Similar to fundamental frequency, changes to formant frequency and spacing may provide important information about the voice [49, 50, 51].
In a study of ten 5-year-old children with cerebral palsy, formant measures were used to investigate dysarthria [51]. Analysis of single word recordings showed that children with dysarthria had smaller second formant ranges when uttering words that are known to require larger changes in vocal tract shape [51]. Formants were also used to collect normative acoustic data in Brazilian children aged 4–8. Seven different vowels were uttered by each of the 207 children. Frequencies of the first three formants were generally higher in girls than boys, and formant frequency decreased with age [52].
Emerging Parameters
While existing acoustic parameters have been beneficial to voice analysis in both research and clinical settings, they demonstrate limitations. Specifically, nonlinear methods including correlation dimension (D2) and Lyapunov exponents are unable to distinguish between low-dimensional, deterministic chaos and high-dimensional, stochastic noise [53]. The implication for voice analysis is that it may be impossible to objectively quantify type 4 voice signals using these methods. This presents a significant limitation, considering that patients with significant breathiness, as in unilateral vocal fold paralysis, may have prominent stochastic noise and thus type 4 characteristics. However, nonlinear dynamic methods have been proposed recently that are better able to handle noise in the voice signal, resulting in fewer computational errors. These methods are spectrum convergence ratio (SCR), nonlinear energy difference ratio (NEDR), and rate of divergence (ROD) [54, 55, 56]. Computation of SCR relies on short-time Fourier transform analysis (STFT), which tracks how signal frequency components change with time. Most importantly, this analysis can be used to detect changes in periodicity. When a signal is more aperiodic or affected by turbulent noise, its spectrum consists of segments that are dissimilar to each other. Because SCR quantifies the convergence of segments, type 1 signals tend to have the highest SCR. A study in adults has shown that SCR can be successfully computed for all four voice types and that average values are significantly different across groups [54].
NEDR is similar to SCR in that it also involves a Fourier transform to decompose the signal into its frequency components. However, NEDR uses an iterative algorithm for calculating spectral energy variation among these frequency components. Briefly, the algorithm uses a nonlinear weighted function to weigh local data points based on their relative position to the data point of interest, before using the weighted function to perform a Fourier transformation. This process is repeated several times to improve the accuracy of the subsequent spectral energy distribution calculation. The output of NEDR characterizes the stability of the voice signal. Periodic signals will exhibit stable energy distributions, while the spectral energy of aperiodic signals will vary over time. NEDR has been found to be lowest in adults with type 1 voices. NEDR has also demonstrated the ability to distinguish among all four signal types [55].
ROD uses a modified algorithm for calculating Lyapunov exponents, which, as discussed previously, are the average exponential rates of divergence or convergence of nearby orbits in phase space. Higher maximum Lyapunov exponents indicate more chaos or instability in the voice signal. A limitation of this parameter is that calculation of Lyapunov exponents requires a known embedding dimension, which cannot be determined for type 4 voices. Rather than calculating Lyapunov exponents directly, ROD calculates the rate of divergence of two nearby points followed in three dimensions only. A pair of points is followed for three sample intervals, before a new pair is chosen. In total, eight fragments are analyzed for each voice sample, and the average value is taken to represent the ROD, which tends to be highest for type 4 voices. ROD has been used to successfully distinguish between all four voice types in a study of adults [56]. The ability to objectively distinguish between voice types 3 and 4 is particularly useful, because it enables the detection of subtler differences that may not be recognized perceptually.
Recently, SCR and ROD were used for the first time to study healthy pediatric voices. Acoustic recordings of 20 adult and 36 pediatric subjects aged 4–17 were taken. Subjects were then grouped according to their voice type using spectrogram analysis and CAPE-V. Mean SCR and ROD were found to be significantly different between the pediatric and adult groups, while jitter and shimmer were not. Using adult reference values for the SCR and ROD boundaries between type 2 and 3 voices, pediatric voices were grouped as primarily periodic or aperiodic. Using the original voice type designation as the true categorization, the adult SCR and ROD reference values were only capable of correctly sorting 36.1% of pediatric subjects. For analysis based on gender, boys in the age groups of 4–7, 8–12, and 13–17 all had similar SCR and ROD values, while girls aged 8–12 and 13–17 had significantly different values of SCR. These findings suggest that future research is needed, particularly for establishing appropriate pediatric reference values for these nonlinear parameters [57]. Potentially, these emerging methods could become clinically useful and be used in conjunction with other parameters to provide a comprehensive acoustic voice assessment.

Full access? Get Clinical Tree
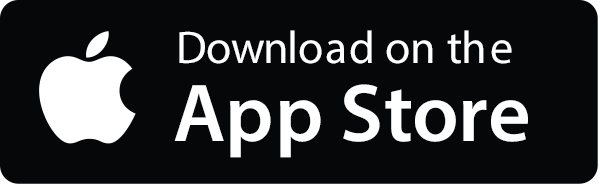
