Fig. 3.1
The LIEFTS model captures absolute thresholds. (a) Schematic envelopes of some of the stimuli for which such thresholds were measured. They are grouped into three categories, multiple-burst (MB), onset–offset (OO), and single-burst (SB) stimuli. (b) Average monaural thresholds, in dB SPL, of 206 ears for all stimuli tested and plotted as a function of stimulus duration excluding the gaps in the MB series. (c) Thresholds after application of the model, with P LIEFTS expressed in dB SPL, plotted as a function of stimulus duration including the gaps in the MB series. Model parameters are specified. The thin gray line has a slope of −1/3
We developed a “continuous look” model, according to which the stimulus generates stochastic detection events (Heil and Neubauer 2003). Threshold is reached when a criterion number of events have occurred (probability summation). The higher the probability of detection events, the shorter the average time required to accumulate the criterion number of events, and vice versa. No long-term integration is required. Our early work (Heil and Neubauer 2003; Neubauer and Heil 2004) allowed us to conclude that the probability of occurrence of the detection events was proportional to the (effective) stimulus amplitude raised to an exponent that was most likely 3, but possibly 4 or 5. We later refined the model (Neubauer and Heil 2008) and applied it to the dependence of the latencies of first spikes (neural detection events) of ANFs on the amplitude and temporal envelope of tonal stimuli (Neubauer and Heil 2008; Heil et al. 2008). The refinement involved the addition of a leaky integrator acting on the stimulus envelope before exponentiation; the time constant was 1–2 ms, similar to the time constant of the IHC membrane. It was also assumed that the detection events are generated by an inhomogeneous Poisson process. We also confirmed the exponent to be 3.
When applying this LIEFTS model (leaky integration, event formation, temporal summation) to our psychophysical threshold data, we can ignore both spontaneous activity and a term capturing the transmission delay. The model can therefore be simplified to


(3.1)
Here T is threshold (in Pa3 · s), R(t) is the rate of detection events, P(t) is the stimulus amplitude (in Pascals), P li(t) is the low-pass filtered stimulus amplitude (in Pascals), τ is the time constant of this filter (∼1–2 ms), α is 3, and k is a scaling factor. The stimulus amplitude at threshold therefore varies with the duration, t s, of the stimulus and with its temporal envelope. Figure 3.1c shows the application of this model to the thresholds shown in Fig. 3.1b. For better comparison, the ordinate in Fig. 3.1c represents the 3rd root of the integral mean of P li 3(t), P LIEFTS, a quantity which can be expressed in dB SPL. If the model fully captured the data, all data points, independent of temporal envelope and duration, would fall on a single line with a slope of −1/3 in this log–log plot (or −6.67 dB per decade of duration). We find this to be the case, except for stimuli of long duration (≥0.3 s) for which thresholds exceed the model predictions slightly (by ∼2 dB for the longest duration).
In the following sections we will suggest one factor that might contribute to the slight discrepancy between the data and model for long stimulus durations, provide evidence for the stochastic nature of the detection events, and confirm the value of the exponent in the model is 3.
2.2 Deviations of Thresholds for Long-Duration Tones from the LIEFTS Model May Be Due to Lower Attention
Here, we provide evidence for lower levels of attention during the presentation of long versus short tones, which might cause detection thresholds for long tones to be slightly higher than predicted by the LIEFTS model (Fig. 3.1c). The evidence comes from eyeblinks (EBs) measured by means of EEG and EOG in 22 subjects while they performed 3-I-3-AFC experiments as described above. EBs can be evoked by visual and (intense) auditory stimuli, but they also occur spontaneously and appear to be related to attention breaks (e.g., Nakano et al. 2009; Schleicher et al. 2008). Figure 3.2a shows the average instantaneous EB frequency during a trial. EBs are evoked by the onsets and offsets of the visual markers of the three observation intervals. EB frequency increases after the last interval and peaks sharply shortly after the button press. Figure 3.2b shows the instantaneous EB frequency over a 2-s epoch around the interval which contained the auditory stimuli. The five traces show the EB frequencies separately for the five different auditory stimuli used in these experiments (viz., 3.125-kHz tones with durations of 8.32, 30.72, 130.56, 264.32, and 1,064.32 ms), and vertical lines mark their timing within the observation intervals. The total number of EBs over the 2-s epoch is similar for the five different stimuli (∼0.3 EB/epoch), but their temporal distribution is different. For the four shorter stimuli, EB frequencies drop to very low values (0.04–0.07 EB/s) around the time of stimulus presentation. For the longer stimulus, the average EB frequency during its presentation is considerably higher (∼0.15 EB/s). If EBs are associated with attention breaks, so that EB frequency is inversely related to attention, then our data suggest that the average level of attention is lower during the presentation of the longest stimulus compared to the shorter stimuli. The EB patterns for those observation intervals which did not contain an auditory stimulus were very similar to that shown in Fig. 3.2b (not illustrated). This suggests that the auditory stimulus itself does not affect EB timing. Rather subjects appear to be aware when, relative to the visual markers, the auditory stimuli will occur, if they occur. This seems plausible since each track started with clearly audible stimulus levels and for each stimulus four tracks were completed by each subject.
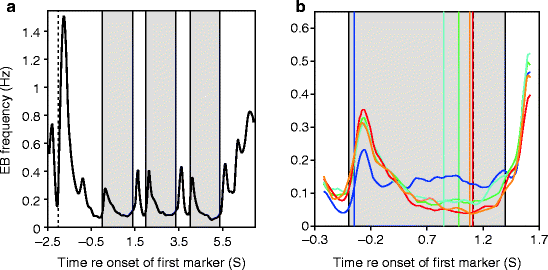
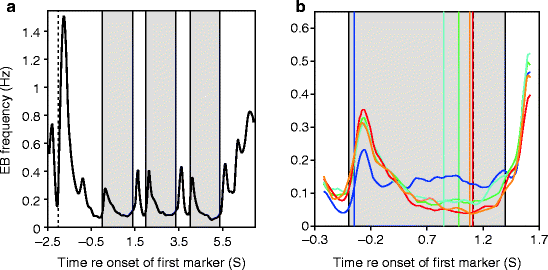
Fig. 3.2
Frequency of eyeblinks (EBs) during threshold measurements differs between long and short tones during their presentation. (a) EB frequency in the course of a trial of the 3-I-3-AFC threshold measurement (based on ∼69.000 EBs, accumulated over all near-threshold trials, stimuli, and subjects). The visually marked observation intervals are identified by gray bars, the button press in response to the previous trial by the vertical dashed line at −2 s. (b) EB frequency during a 2-s epoch around the interval during which the auditory stimulus was presented. EB traces are separated according to stimulus duration. Colored vertical lines mark the onsets of the five different stimuli, the dashed vertical line their common offset
In summary, the differential EB frequencies, and – by inference – levels of attention, during the presentation of short versus long tones may be one factor underlying the elevation of predicted thresholds for long-duration stimuli (Fig. 3.1c).
2.3 The Stochasticity of the Detection Events Can Be Shown by Means of Simple Reaction Times
The 3-I-3-AFC procedure does not allow examination of the time at which a particular stimulus is detected, but the stochastic nature of the detection events in the LIEFTS model can be tested using simple reaction times (SRTs). We have previously shown (Heil et al. 2006) that an individual SRT can be conceived of as the sum of the time required to execute the motor response and the time that a stimulus must be on before the subject detects the stimulus. We refer to this latter component as the initial effective stimulus portion. The LIEFTS model predicts that (1) the initial effective stimulus portion should most often be shorter than the stimulus duration. Hence, SRTs can be, but due to the motor component need not be, shorter than the stimulus duration. (2) For any given stimulus, which is detected on at least some of its presentations, there should be trial-to-trial variation in the duration of its initial effective portion. This causes, or contributes to, the trial-to-trial variation in SRTs. (3) When the amplitude of a test stimulus is increased, with other parameters unchanged, detection probability should increase and the average duration of the effective initial portion of the stimulus, and its variability, should decrease. Thus, mean SRT and the associated variability should decrease, so that the cumulative distributions of SRTs to test stimuli of different amplitudes diverge early and increase in steepness with increasing test stimulus amplitude. (4) When the duration of a test stimulus is increased, with other parameters unchanged, detection probability should also increase. In this case, however, the cumulative distributions of SRTs to test stimuli of different durations should have a common initial course. For any two stimuli of different durations, the common course of the SRT distributions should last as long as the duration of the shorter stimulus. (5) Since the exponent α in the LIEFTS model is associated with the (low-pass filtered) stimulus amplitude and is greater than 1, the increase of detection probability due to increasing stimulus duration by some factor is less than that due to increasing stimulus amplitude by the same factor.
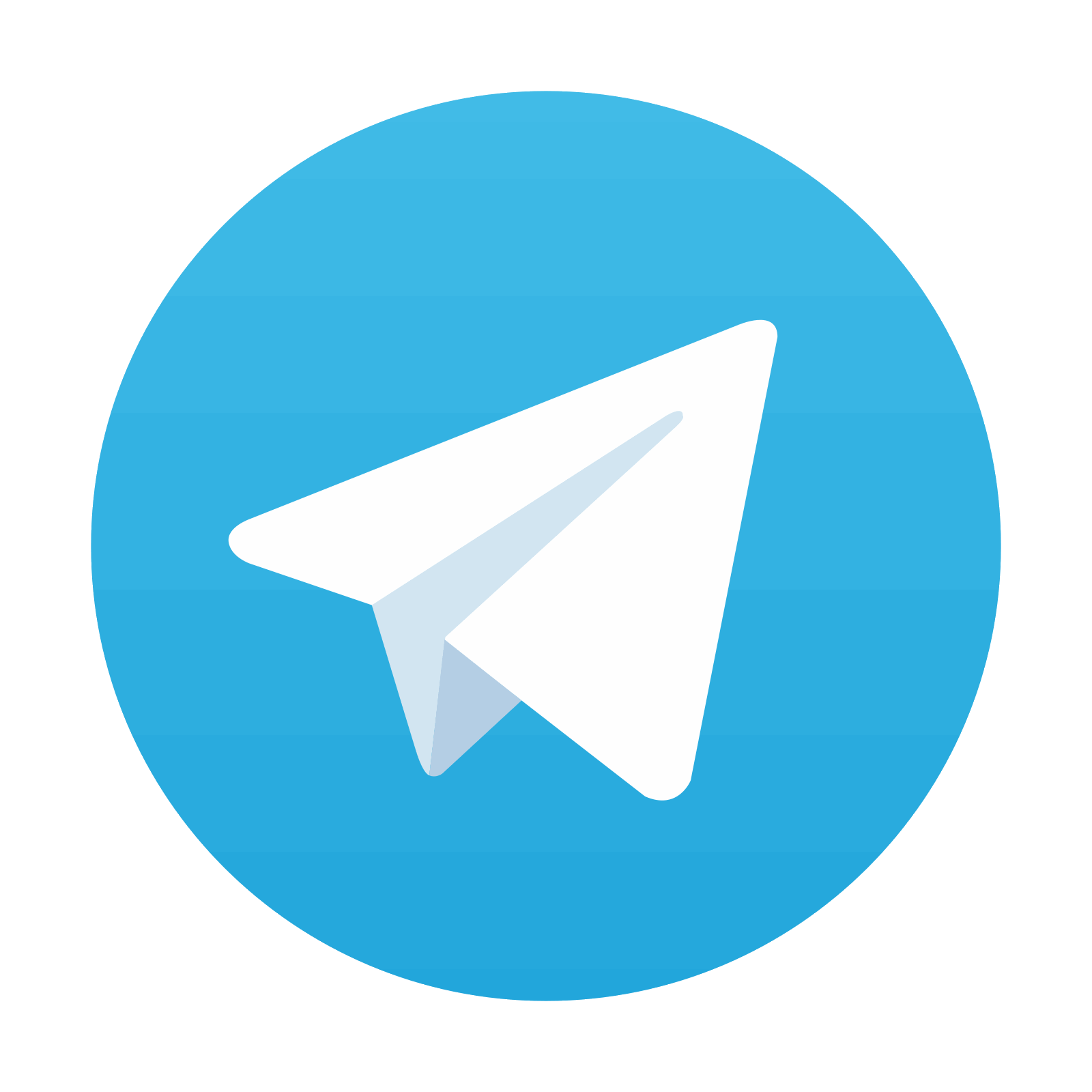
Stay updated, free articles. Join our Telegram channel

Full access? Get Clinical Tree
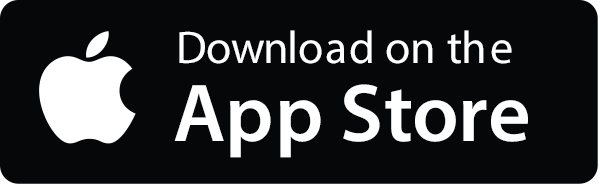
