Fig. 2.1
Flow diagram of the MATLAB Auditory Periphery (MAP) model. The lower boxes on the left refer to activity driven by low spontaneous rate (LSR) fibres and forming (speculatively) part of the acoustic reflex (AR) circuit. The boxes on the right are driven by high spontaneous rate (HSR) fibres and form part of the MOC efferent circuit. CN cochlear nucleus
Of course, such a model is only as good as its components. Fortunately, the output of individual modules can be evaluated against published physiological data. The output of each stage is expressed in terms of measurable variables such as stapes displacement, basilar membrane (BM) displacement, inner hair cell (IHC) receptor potential, auditory nerve (AN) firing rate and the pattern of firing in individual brain stem neuronal units. The architecture of the model allows us to carry out pseudo physiological experiments by applying acoustic stimulation while measuring the response at the output of a particular stage and then checking against corresponding published data.
Figure 2.2 shows the output of the model at a number of stages in response to the word ‘twister’ presented at 50 dB SPL. Successive panels show the stimulus, the stapes response, a 21-channel BM response as well as three levels of neuronal response; the AN, cochlear nucleus (CN) chopper response and a second-level brainstem response. Figure 2.2b shows the multichannel activity in the MOC efferent. The AR is not activated at this stimulus intensity. Each panel represents an ‘inspection window’ for the corresponding stage.
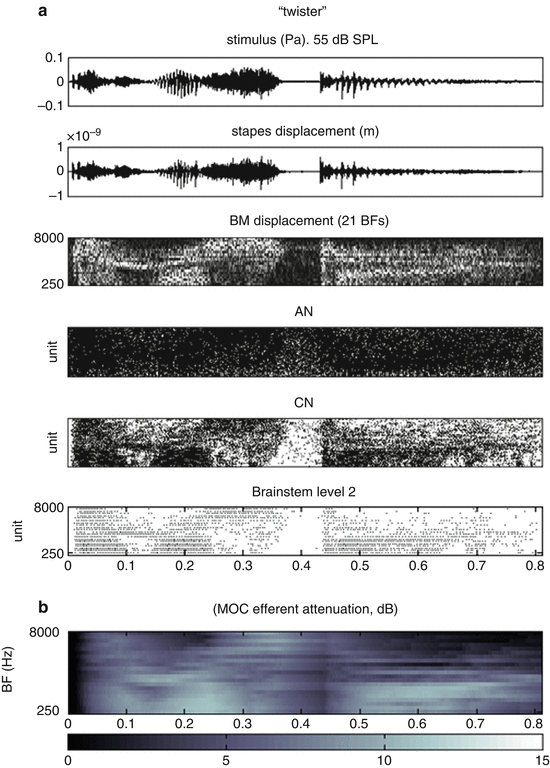
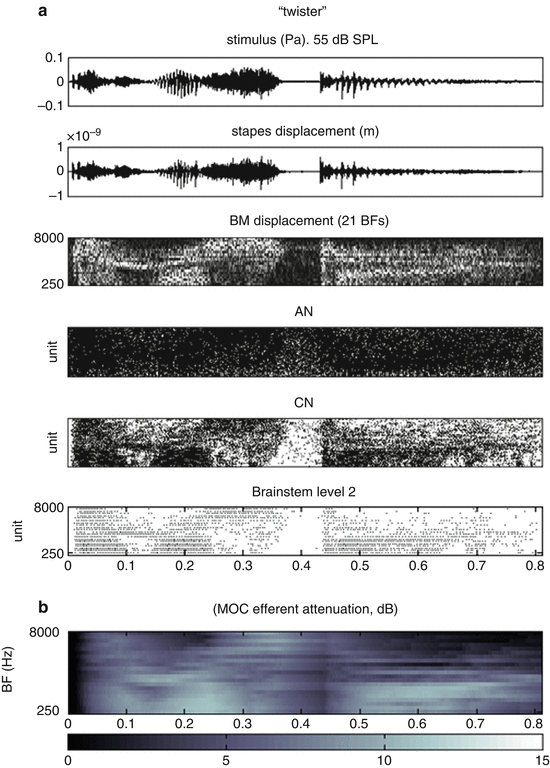
Fig. 2.2
Output from the auditory model. (a) Stimulus and output from five stages of the afferent part of the model (stapes, BM, AN, CN chopper, 2nd-level brainstem units). X-axis is time. (b) Activity in the efferent pathway of the model; time x channel attenuation of nonlinear DRNL input
3 Model Applications
The model is not just a computerised visual display. It has a number of applications. One is to use the AN spiking pattern as the ‘front end’ to another system that represents a theory of how sensory decisions are made. In the past we have used it as the input to an autocorrelation model of pitch processing and segregation of simultaneous vowels presented with different pitches. Indeed, the majority of requests from potential users of the model concern the need for a front end of this type.
One might expect that a good auditory model should make an ideal front end to an automatic speech recogniser with recognition performance close to human levels. Good performance can be achieved for speech presented in quiet but performance declines substantially in the presence of background noise. This has led us to include a simulation of the peripheral efferent system in the model because it moderates the strength of the system’s response in proportion to the intensity of the background. This reduces the spread of excitation across frequency channels and produces a more stable representation. The model components representing the efferent system were first evaluated against the physiological data and then tested in studies using automatic speech recognition (ASR) techniques. The modelled efferent system includes both a MOC arrangement and a simulation of the acoustic reflex. It was possible to compare speech recognition as a function of signal-to-noise ratio (SNR) both with and without the benefit of the closed-loop multichannel efferent reflex. The unfilled squares in Fig. 2.3 show how poorly the unimproved model works as an auditory front end. A 50 % recognition rate requires 15-dB SNR. However, when the efferent pathway is enabled, performance is greatly improved. At 10-dB SNR the recognition rate rises from 30 to 90 %. The modelling exercise does not prove that the MOC is critical for perception of speech in noise, but it does illustrate how modelling can be used to explore the hypothesis. The results also show that human performance remains much better than that of the model!
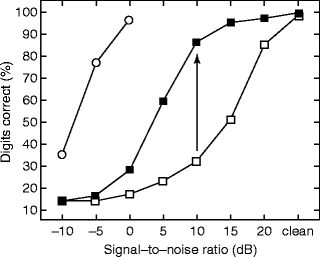
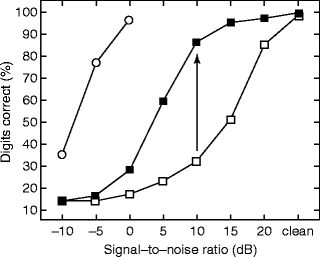
Fig. 2.3
ASR performance (% correct) as a function of SNR. The speech was connected digit triplets using both male and female talkers. The ‘noise’ is 20-talker babble. Representative human performance on the same test is shown as unfilled circles. Model performance without the efferent system is shown as unfilled squares. Improved performance using the efferent system is shown as filled squares
4 Psychophysics
Models can help understand the relationship between hearing and the underlying physiology by comparing model performance with that of human listeners in psychophysical experiments. Of course, some principle must first be established to convert the model multichannel output to a simple psychophysical response. For example, in a single-interval, adaptive tracking paradigm, the output must be converted to a ‘yes’ or ‘no’ response. Simple tasks such as detecting a tone against a silent background can be performed by creating neuronal units that never (or very rarely) spike in silence. Any response in any one of them can, therefore, be used to indicate that something has been detected.
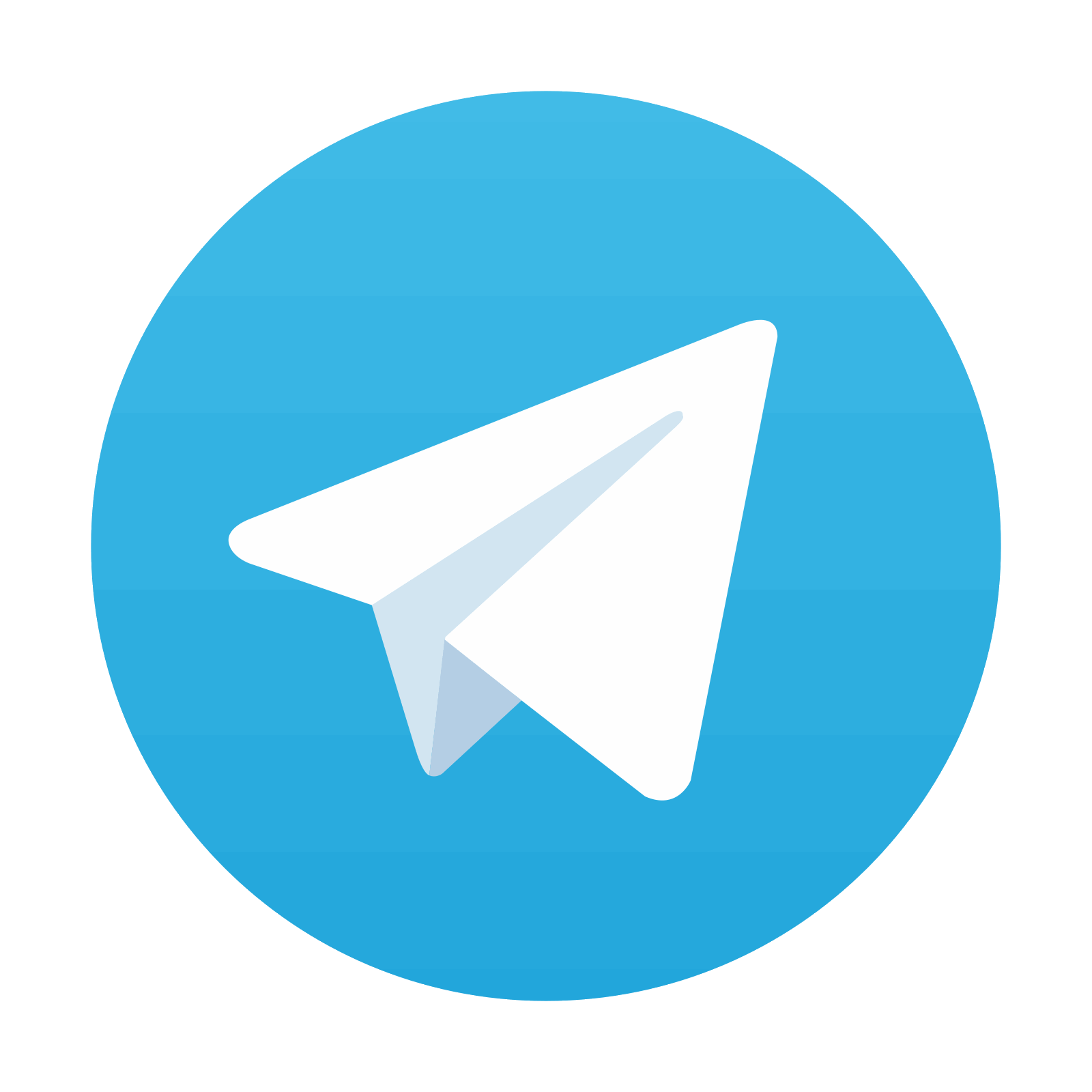
Stay updated, free articles. Join our Telegram channel

Full access? Get Clinical Tree
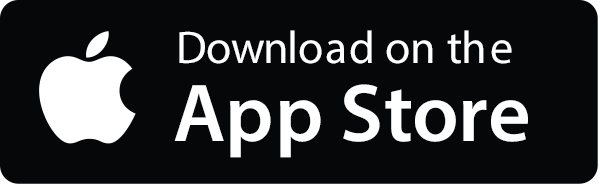
