Purpose
To assess the efficacy of novel Digital spectacles (DSpecs) to improve mobility of patients with peripheral visual field (VF) loss.
Design
Prospective case series.
Methods
Binocular VF defects were quantified with the DSpecs testing strategy. An algorithm was implemented that generated personalized visual augmentation profiles based on the measured VF. These profiles were achieved by relocating and resizing video signals to fit within the remaining VF in real time. Twenty patients with known binocular VF defects were tested using static test images, followed by dynamic walking simulations to determine if they could identify objects and avoid obstacles in an environment mimicking a real-life situation. The effect of the DSpecs were assessed for visual/hand coordination with object-grasping tests. Patients performed these tests with and without the DSpecs correction profile.
Results
The diagnostic binocular VF testing with the DSpecs was comparable to the integrated monocular standard automated perimetry based on point-by-point assessment with a mismatch error of 7.0%. Eighteen of 20 patients (90%) could identify peripheral objects in test images with the DSpecs that they could not previously. Visual/hand coordination was successful for 17 patients (85%) from the first trial. The object-grasping performance improved to 100% by the third trial. Patient performance, judged by finding and identifying objects in the periphery in a simulated walking environment, was significantly better with the DSpecs ( P = 0.02, Wilcoxon rank sum test).
Conclusions
DSpecs may improve mobility by facilitating the ability of patients to better identify moving peripheral hazardous objects.
Peripheral visual field (VF) defects can be caused by several diseases, including glaucoma, cerebrovascular accidents, retinitis pigmentosa, and choroideremia. More than 75 million patients are projected to experience irreversible VF loss secondary to glaucoma by 2020. Visual impairments complicate the course of 72% of all cerebrovascular accidents. Binocular VF losses are associated with reduced quality of life activities for many patients. Peripheral vision losses affect postural stability, , motion estimation, and the ability to avoid hazardous peripheral obstacles. Reduced VF total area and narrower visual fields worsen mobility performance. Despite recent advances, no medical or surgical treatment can reverse existing damage associated with either glaucoma or stroke. Visual aids attempt to maximize patient functionality , yet often fail to achieve this goal. , Research to evaluate effectiveness of visual aids for patients with damaged peripheral visual field is limited. ,
Current visual aids relocate or minimize the captured scenes to fit within the assumed intact VF. To achieve these goals, investigators have used both optical and electronic/digital solutions. Optical approaches use prisms or magnifying components to expand the field of view. These strategies have not been widely accepted because they result in reduced resolution and overlapping image effects and require the patient to scan the environment using the head rather than eye movements.
Electronic or digital based visual aids apply enhancement techniques to improve central vision by adjusting image contrast, brightness, color, and edge properties with head-mounted displays (HMDs). , , Representative examples of this technology include Esight (Ontario, Canada), Jordy (Enhanced Vision Systems, Huntington Beach, California), Flipperport (Enhanced Vision Systems), and Oxsight (Oxsight, Oxford, UK) devices. Clinical trial results and studies performed with these aids are limited , and have focused on testing central vision tasks, such as writing, reading, and identifying objects. Although these tests showed improvements in central vision-related tasks, the ability to avoid collisions and restore mobility did not improve. , Failure to achieve this goal is likely related to the fact that current aids do not quantify patient-specific visual field defects or apply a unique strategy to augment visual function. , , Some investigators have used HMDs to measure or screen monocular VFs , but did not use this information to provide patient-specific solutions. A uniformly applied criterion to define the benefit of a specific visual aid for improving mobility, independence, and safety would facilitate comparisons among devices. Consequently, a need exists for a new low-vision aid to improve mobility.
This paper reports the development and use of Digital Spectacles (DSpecs) that measured binocular VF defects and applied a digital image processing strategy unique to each patient to create a visual augmentation profile. Real-time augmentation was performed by rescaling and shifting video images of patients’ scene to fit within the remaining intact VF. To demonstrate the patients’ improved ability to avoid and identify moving objects located in the periphery, the device was tested in a dynamic walking simulation environment specifically designed to assess mobility improvements.
Subjects and Methods
A commercial virtual reality (VR) HMD was used to develop the DSpecs visual aid (HTC Vive, Xindian District, New Taipei City, Taiwan). The HMD was integrated with an eye-tracking system (Tobii Technology, Danderyd, Sweden) that fed gaze data to the VF testing algorithm ( Figure 1 A). The visual aid was also equipped with 2 high-definition (HD) 2- megapixels miniature cameras (Camera sensor: OmniVision, Santa Clara, California) ( Figure 1 B). The 2 cameras provided a binocular view. The cameras provided a field of view of approximately 85° in diameter, which matched the 80-degree VF testing range of the DSpecs. The VR HMD and the eye-tracking system permit a 100-degree field of view (FOV) for each eye. The DSpecs is controlled by a VR-enabled laptop (Core i7, 2.8GHz Quad core, NVIDIA 1070, 32GB RAM; Intel, Miami, Florida) that runs the binocular VF testing program and video processing algorithm. The developed algorithms were implemented with MATLAB version R2018b software (MathWorks, Inc., Natick, Massachusetts) and C# under Unity (Unity Technologies, San Francisco, California).

Participants Recruitment
The University of Miami institutional review board approved the study before patient recruitment. Patients signed informed consent forms before commencing the study. The design was in accordance with the Declaration of Helsinki and all HIPAA regulations. A test group consisting of 20 patients recruited from glaucoma and neuroophthalmology clinics at the Anne Bates Leach Eye Center, Bascom Palmer Eye Institute, University of Miami Miller School of Medicine were examined during their regular follow-up visits. All patients had previously performed either the 24-2 or 30-2 testing strategy with the Humphrey Zeiss standard automated perimetry (SAP) (Carl Zeiss Meditec, Dublin, California).
Measuring the Binocular Visual Field
The VF perimetry method with the DSpecs was based on the SAP static technique with a fast threshold strategy. Locations of stimuli were determined by 52 points of a circular grid that covered the central 40-degree radius of both eyes, with an interspace of 10° between each stimulus location. Four contrast staircase stimuli sequences were presented ranging between 32 dB and 20 dB in steps of 4 dB. A value of 0 dB was assigned to the stimuli locations where the patient could not respond. This testing method used a bright background with an illumination of 100 lux, and the stimuli were dark dots.
This method of testing was relatively accurate for monocular and binocular VF testing. The patient was asked to fixate on a central target, and the eye-tracking system monitored fixation during the test. If the patient looked at the stimulus, the test was halted until fixation was restored based on feedback from the eye-tracking system.
Based on a normal human FOV, , , the common binocular area covered by both eyes is approximately the central 60-degrees radius. The VF test covered the central 40-degrees radius area, a subset region of the total binocular area. Our binocular VF test presented the stimuli to both eyes at the same visual location and time, so that either the left or right eye would detect the stimulus and the patient would respond accordingly. The stimulus would only be marked as “unseen” if both eyes were functionally defective at the same visual location in the binocular VF.
Visual Field Measurements Accuracy
To assess the accuracy of the DSpecs’ binocular VF testing method, an estimated binocular VF was first constructed based on the monocular SAP VFs of 20 patients. The estimated binocular VF was constructed by integrating the right and left eyes’ monocular VF. Monocular integration was performed by selecting the maximum sensitivity value at each position of the monocular VFs. This merging process was based on the maximum sensitivity merging model reported by Crabb and associates and the best location integrated binocular model reported by Nelson-Quigg and associates. The predicted binocular VF was used as a reference to evaluate the accuracy of the DSpecs’ binocular test. The most recent monocular SAP VF tests were used to construct integrated visual field (IVF) binocular plots. The central 24- or 30-degree area, depending on previous testing, were compared between the IVF and DSpecs binocular VF. Measurement errors were based on pointwise mismatches between the 2 methods.
Image Remapping
Image remapping required shifting and resizing operations on the 85- degrees FOV input video signals to fit within the intact regions of the measured binocular VF to increase the functional FOV. The remapping method used VF test data to estimate a new scale and new center for the output video signals to be presented. Geometric image properties of the measured intact binocular VF were calculated, including the areas in pixels, bounding pixels, and the weighted center of mass. If multiple intact regions existed in the field, only the largest area was considered for remapping to maximize utilization of the remaining VF. The geometric calculations were fed to a mathematical function to calculate a new center and scale for the video signals to be specifically presented to each patient. Calculation of the patient unique remapping parameters and video processing (shifting and resizing) was performed in real time through the control program. Processed video signals were displayed at a speed of 24 frames per second.
Figure 2 shows an example application of the video remapping process to fit input images within the intact region of a certain VF automatically. Figure 2 A shows a scene of a shopping mall as seen through normal vision ( Figure 2 B) is a VF with peripheral defects. Figure 2 C shows the scene without remapping where the escalator’s entrance could not be seen. Figure 2 D shows the remapped scene where the whole escalator can be noticed. VF was superimposed over the scenes in Figure 2 , C and D for demonstration purposes only.

Before commencing the walking simulation, we tested the effectiveness of image remapping to augment the functional FOV with static test images of incoming cars in different image quadrants. The test images were first presented without remapping, then with remapping activated. We asked patients to identify the exact nature of the object in the images.
Walking Simulation Test
A computer-based walking simulator was designed and constructed for testing the visual aid. The walking simulator ensured the patient safety, where no fall could occur, before testing the use of DSpecs in a walking track.
Hand coordination test
It was determined whether the DSpecs adversely affected hand coordination before performing the walking simulation test. Patient visual/hand coordination was tested with the DSpecs while activating the binocular video remapping strategy to ensure cognitive coordination between hand movements and visual perception with the remapped video signals. In this test, 3 objects with different sizes (height × width: 23 × 5, 13 × 4, and 7.5 × 3 cm, respectively), were randomly placed at different distances (51, 62, and 70 cm, respectively) on a table in front of the patient. Patients were required to grasp these 3 objects one at a time, and the number of grasp trials was recorded for each object.
Walking simulation environment
We constructed a walking computer simulation test environment to project an 80 × 60-inch image on the wall. The simulation environment was constructed geometrically with SketchUp software (Trimble, Sunnyvale, California) and then converted to three-dimensions and animated using SimLab VR software (Simulation Lab Software, Amman, Jordan). The image of the projector screen covered 80 degrees of the FOV, which matched the DSpecs VF testing range.
Participants performed the simulation while seated in front of the screen, where a scene portrayed a long corridor with an equivalent length of 72 meters. Average simulated walking speed was 2.45 m/s. The participants were able to navigate with a gaming joystick. All participants were instructed to look at the end of the corridor (center of the screen) as a fixation target to limit the confounding effect of eye and head movements scanning. We asked patients to identify the type and shape of 8 peripheral objects located above the central horizontal line of sight in the walking corridor. These shapes were initially hidden but appeared when the virtual walker was within approximately 2 meters of each object. The objects located on the walls included common shapes, for example, a large clock and paintings with basic shapes (circles, triangles, and the letter “X”). The shapes were projected to test the 2 superior VF quadrants in which each quadrant was stimulated 4 times during the test, yielding 8 static shapes ( Figure 3 A).

Six moving obstacles were created to block the passage of the patient from both sides (initially hidden obstacles: chairs, couches, and tables). The obstacles moved to block the virtual walker when within approximately 2 m of each obstacle. Participants were asked to stop walking when they noticed the obstacle ( Figure 3 B). The simulation software mimicked a collision situation and halted forward movements if the participant did not notice the moving obstacle. The moving obstacles were used to test functionality of the 2 inferior VF quadrants, where each quadrant was stimulated 3 times; therefore, the simulation environment had 6 moving obstacles.
A demonstration version of the walking simulator was presented that displayed sample obstacles and shapes before performing the complete track to demonstrate the test and describe the walking controls. The demonstration corridor was 28 m long, and patients took approximately 2 min to complete this learning phase, including explanations by the tester. The patients repeated the demonstration 2 to 4 times until they felt conformable with the simulation environment and understood how to respond. This step was undertaken to minimize the potential learning effect on the recorded visual identification responses.
Two walking testing strategies with different obstacles and shapes distributions were displayed. Immediately after completing the virtual walk with 1 strategy with the DSpecs, the patient would proceed through the other theme without the DSpecs. This was chosen to minimize memory or practice effects, so that the patient would not memorize landmarks from the first completed course. Patients were asked to walk through the corridor twice for each setup (with and without DSpecs). They performed 2 different themes with the DSpecs and 2 additional themes without the DSpecs. The order of the walking trials was randomly assigned. Each trial was completed in 1 to 2 min depending on how many times the patient stopped during the test and responded to the appearance of different obstacles and shapes. Both types of scores (obstacle avoidance/stopping scores and object identification scores) were recorded and calculated for all participants’ trials in both testing strategies by 2 observers to make sure that the correct scores were being recorded.
Descriptive and significance statistics were calculated using MATLAB R2018b software. Mean ± SD were used to describe VF measurement errors. Wilcoxon rank-sum tests were used to test for significance between the patients walking simulation scores, with and without the DSpecs. Pearson’s linear correlation tests were used to assess the correlation of 3 VF defect severity measurements and the walking simulation average scores. Mean deviation (MD), pattern standard deviation (PSD), and visual field index (VFI) metrics were included in the correlation analysis, because they are commonly reported VF defect characterization parameters. , For each measurement, the best eye’s value was included in the analysis. P values of less than 0.05 were considered statistically significant.
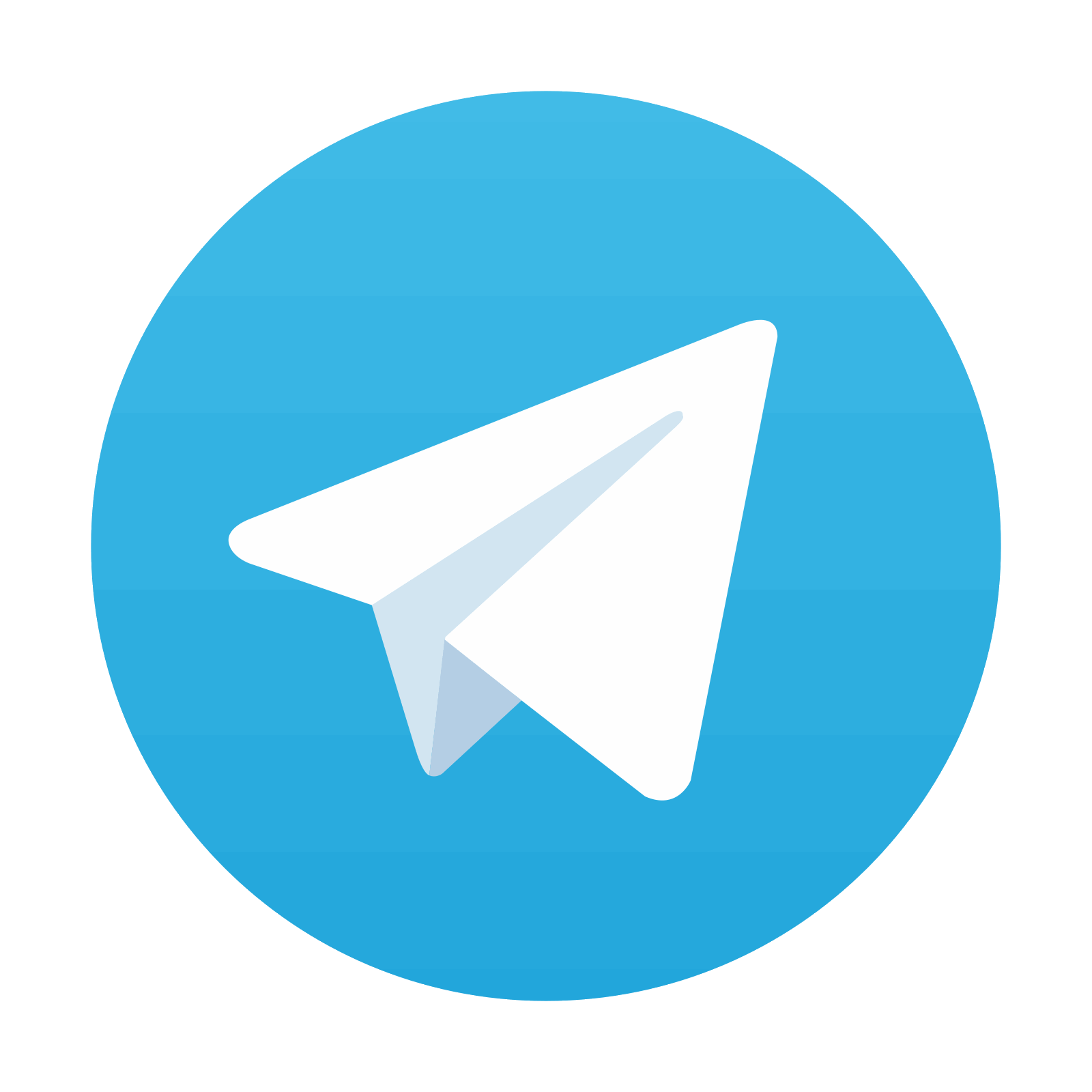
Stay updated, free articles. Join our Telegram channel

Full access? Get Clinical Tree
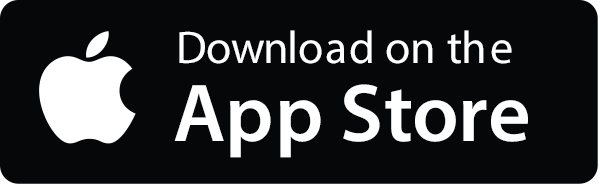
