Purpose
To assess whether the 3-dimensional (3D) structural configuration of the central retinal vessel trunk and its branches (CRVT&B) could be used as a diagnostic marker for glaucoma.
Design
Retrospective, deep-learning approach diagnosis study.
Methods
We trained a deep learning network to automatically segment the CRVT&B from the B-scans of the optical coherence tomography (OCT) volume of the optic nerve head. Subsequently, 2 different approaches were used for glaucoma diagnosis using the structural configuration of the CRVT&B as extracted from the OCT volumes. In the first approach, we aimed to provide a diagnosis using only 3D convolutional neural networks and the 3D structure of the CRVT&B. For the second approach, we projected the 3D structure of the CRVT&B orthographically onto sagittal, frontal, and transverse planes to obtain 3 two-dimensional (2D) images, and then a 2D convolutional neural network was used for diagnosis. The segmentation accuracy was evaluated using the Dice coefficient, whereas the diagnostic accuracy was assessed using the area under the receiver operating characteristic curves (AUCs). The diagnostic performance of the CRVT&B was also compared with that of retinal nerve fiber layer (RNFL) thickness (calculated in the same cohorts).
Results
Our segmentation network was able to efficiently segment retinal blood vessels from OCT scans. On a test set, we achieved a Dice coefficient of 0.81 ± 0.07. The 3D and 2D diagnostic networks were able to differentiate glaucoma from nonglaucoma subjects with accuracies of 82.7% and 83.3%, respectively. The corresponding AUCs for the CRVT&B were 0.89 and 0.90, higher than those obtained with RNFL thickness alone (AUCs ranging from 0.74 to 0.80).
Conclusions
Our work demonstrated that the diagnostic power of the CRVT&B is superior to that of a gold-standard glaucoma parameter, that is, RNFL thickness. Our work also suggested that the major retinal blood vessels form a “skeleton”—the configuration of which may be representative of major optic nerve head structural changes as typically observed with the development and progression of glaucoma.
G laucoma is the leading cause of irreversible blindness worldwide. It is estimated that over 57.5 million individuals are suffering from primary open-angle glaucoma, with 10% being blind in both eyes. , There are often no symptoms until the disease reaches a late stage, and an estimated 50% of all glaucoma sufferers do not know they have it. Although there is no cure for glaucoma, vision can be preserved if it is diagnosed at an early stage. As a neuropathic eye disease that results in the progressive loss of retinal ganglion cells (RGCs), glaucoma causes measurable structural and functional damage to the optic nerve head (ONH) and retinal nerve fiber layer (RNFL). Increased intraocular pressure (IOP) is commonly associated with glaucoma, and it is the only modifiable risk factor. However, a significant population of glaucoma sufferers do not have increased IOP, and many of the functional tests used can only detect glaucoma after more than 30% of all RGCs are damaged. Other structural parameters such as the neuroretinal rim loss would result in only approximately 40% diagnosis accuracy. To improve the diagnostic accuracy of tests for glaucoma, there is a need to identify novel structural biomarkers.
Over the past few decades, our group (and others) have identified a plethora of structural parameters that could be used clinically as glaucoma biomarkers. For instance, morphological parameters of the ONH such as rim area, disc area, average cup-to-disc ratio, vertical cup-to-disc ratio, prelamina depth, and different retinal layer thicknesses could be used to determine glaucoma status. , , However, less emphasis has been put on the vasculature of the ONH. This may play an important role in glaucoma diagnosis because it is believed that the structural configuration of the main retinal vasculature provides mechanical strength to the ONH and could restrict glaucomatous structural changes in the ONH region. Varma and associates also showed that the central retinal blood vessel trunk and its branches (CRVT&B) shifts nasally over time as glaucoma progresses, and such shifts could be identified from serial photographs by human observers. In all, these studies suggest that the CRVT&B may play a secondary role, such as maintaining the structural integrity of the ONH. Furthermore, the CRVT&B experience important structural changes during the development and progression of glaucoma that could accelerate further damage, especially, in the temporal region where nerve tissues are more exposed. In other words, the 3D structural configuration of the CRVT&B could potentially be used as a glaucoma biomarker, and to date, this has never been assessed. Furthermore, ischemia has been proposed as a mechanism in glaucoma, but this has largely been underinvestigated.
This study looked at the impact of the CRVT&B on glaucoma diagnosis using deep learning techniques to extract the 3D structural configuration of the CRVT&B from optical coherence tomography (OCT) images and a novel method of excluding all the other tissues. Crude blackout maps to interrogate a convolutional neural network (CNN) of the whole of the ONH would not be able to deliver such sophisticated results as this.
Methods
Patient recruitment and OCT imaging
A total of 4108 subjects (1639 glaucoma and 2469 nonglaucoma) were recruited for this study at 3 different sites: the Singapore National Eye Centre (SNEC, Singapore), the Aravind Eye Hospital (Madurai, India), and the Vilnius University Hospital Santaros Klinikos (Vilnius, Lithuania). The study at SNEC had 6 different cohorts of Indian and Chinese ethnicities (cohort 1: 51 glaucoma and 52 nonglaucoma; cohort 2: 12 and 736; cohort 3: 7 and 1128; cohort 4: 193 and 0; cohort 5: 220 and 128; and cohort 6: 0 and 39), whereas the cohorts from India (cohort 7: 1046 glaucoma and 425 nonglaucoma) and Lithuania (cohort 8: 110 glaucoma and 0 nonglaucoma) included Indian and Caucasian ethnicities, respectively (see Table 1 for details). All subjects gave written informed consent. The study adhered to the tenets of the Declaration of Helsinki and was approved by the institutional review board of the respective hospitals. Subjects with an IOP of less than 21 mm Hg, healthy optic discs with a vertical cup-to-disc ratio (VCDR) less than or equal to 0.5, and normal visual field tests were considered as nonglaucoma, whereas subjects with glaucomatous optic neuropathy, VCDR >0.5, and/or neuroretinal rim narrowing with corresponding repeatable glaucomatous visual field defects were considered as glaucoma. A detailed description for glaucoma diagnosis is provided in our previous works. , Subjects with corneal abnormalities and cataract that have the potential to preclude the quality of the scans were excluded from the study.
Institute | Study | Ethnicity | Age (mean ± SD) | Sex (% of male) | Nonglaucoma Volumes | Glaucoma Volumes | Total |
---|---|---|---|---|---|---|---|
Singapore National Eye Centre | Cohort 1 | Chinese/Indian | 64.3 ± 7.1 | 49 | 788 | 51 | 839 |
Cohort 2 | Chinese | 59.6 ± 9.9 | 51 | 1316 | 15 | 1331 | |
Cohort 3 | Indian | 57.7 ± 9.9 | 50 | 2055 | 8 | 2063 | |
Cohort 4 | Chinese | 59.1 ± 8.9 | 52 | 0 | 494 | 494 | |
Cohort 5 | Chinese/Indian | 66.5 ± 5.5 | 58 | 128 | 220 | 348 | |
Cohort 6 | Chinese/Indian | 30.1 ± 4.0 | 80 | 39 | 0 | 39 | |
Aravind Eye Hospital, India | Cohort 7 | Indian | 56.8 ± 11.8 | 75 | 741 | 1970 | 2711 |
Vilnius University Hospital, Lithuania | Cohort 8 | Lithuanian/Caucasian | 67.3 ± 8.5 | 53 | 0 | 110 | 110 |
5076 | 2868 | 7935 |
A standard spectral-domain OCT system (Spectralis; Heidelberg Engineering, Heidelberg, Germany) was used to scan both eyes of each patient. During the scanning, patients were seated in a dark room and imaged using a single operator at each center. Each OCT volume obtained from patients consisted of 97 horizontal B-scans (32 µm distance between B-scans, 384 A-scans per B-scan), covering a rectangular area of 150 × 100 centered on the ONH.
Manual segmentation of the CRVT&B in OCT images as required for training
The CRVT&B (including both arteries and veins) were manually segmented from the 2D raw OCT B-scans by an expert observer (H.C.) and then reviewed by additional experts (T.A.T. and M.J.A.G.). Any disagreements were resolved by mutual discussions, and manual segmentations were corrected whenever needed. We manually segmented 3783 OCT images from 39 subjects (cohort 6 from SNEC) using Amira-Avizo (version 5.4; FEI, Hillsboro, Oregon, USA) to generate binary segmentation masks: blood vessels (arteries and veins) were labeled as 1 and the background and any other tissues as 0 (see Fig. 1 , a and b). The 2D segmentations were visualized in 3D to ensure continuity of the vessels ( Fig. 1 , c and d). Before the manual segmentation, all OCT images were postprocessed using compensation (with a contrast exponent of 4). This approach considerably enhanced the visibility and contrast of all major vessels.

A segmentation network to isolate the CRVT&B structure
The segmentation network consisted of a DeepLabV3 model with a ResNet-101 backbone from the torchvision model zoo for segmentation, with the number of output channels reduced to 1 (see Supplemental Appendix for a detailed architecture). , The network was trained on a Nvidia 1080Ti GPU card for approximately 96 hours until convergence was reached. To assess segmentation performance, Dice coefficients (DC) were calculated by comparing the network predicted labels with those obtained from manual segmentations. The DC was defined as DC = 2TP/(2TP+FP+FN), where TP is the number of correctly predicted vessel pixels, FP the number of wrongly predicted vessel pixels, and FN is the number of wrongly predicted nonvessel pixels. A value of 1 for the DC indicates a perfect match between the network prediction and the manual segmentation, and a value of zero indicates no overlap. We also calculated the Jaccard index (JI) to determine the performance of our segmentation network. The Jaccard index was defined as JI = TP/(TP+FP+FN).
Data cleaning and preparation for glaucoma diagnosis
The segmented images of the CRVT&B, as generated by the network ( Fig. 2 , a-c), contained information only about the structure of the CRVT&B and no other structures such as ONH tissue layers or image artifacts. These segmented images generated by the first network were processed by a second neural network for glaucoma diagnosis. To examine the 3D structure of the CRVT&B, at first, we used a deep learning network with 3D convolutional layers (3D-CNN). However, 3D convolutional networks are computationally expensive and difficult to train as compared with deep learning networks with 2D convolutional layers (2D-CNN). Therefore, to exploit the efficacy of the 2D-CNN and to make the diagnosis computationally less expensive, we also proposed a novel method for diagnosis using the orthographic projections of the 3D CRVT&B structure. An orthographic projection is a way of representing a 3D object by using several 2D views of the object. To this end, the 3D structure of the CRVT&B was projected orthographically onto the sagittal, frontal, and transverse planes ( Fig. 2 , d). We believe that the use of projected 2D images could facilitate the deep learning analysis as we found them easier to interpret. For instance, it is possible to interpret cupping or bowing just by looking at the projections in the sagittal and transverse planes. Other structural features such as thin vessels and nasalization of the trunk should in principle also be easier to decipher in the 3 proposed planes.

Use of 3D-CNN and 2D-CNN for glaucoma diagnosis
Glaucoma diagnosis using the full 3D structural configuration of the CRVT&B
For this task, we designed a custom 3D-CNN based on EfficientNet-B0. We refer to this network as EfficientNet3D. The input to this network was the complete 3D structure of the CRVT&B, as shown in Figs. 2c and 3a , and the output was a binary classification (glaucoma/nonglaucoma).
To make the computational process less expensive, each 3D volume was first down-sampled to 128(depth) × 64(height) × 128(width) voxels. We used the same optimizer as that of the 2D variant of EfficientNet, that is, stochastic gradient descent with 0.9 momentum and a 0.0001 learning rate. The EfficientNet3D architecture is shown in Fig. 3 , a, where k is the kernel size of each operation. MBConv represents an inverted residual block with a squeeze-and-excitation block. The first MBConv had an expansion ratio (exp) of 1, whereas the rest had expansion ratios of 6. Sigmoid linear units (SiLU) were used in each stage as a nonlinear activation function. All convolutional layers were 3D convolutions. The MBConv block architecture is shown in Fig. 3 , b, where A represented the number of input channels of the MBConv block, and B is the number of output channels. Layers 4 and 5 in Fig. 3 , b, are the squeeze and excitation layers, respectively. Skip connections and drop sample layers were added whenever A was equal to B. Drop sample layers randomly zero out samples in a minibatch and multiplies the intensities of the remaining samples by 2.
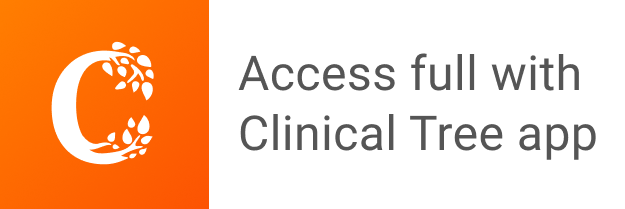