Abstract
Purpose
For spatiotemporal analysis to become a relevant clinical tool, it must be applied to human vocal fold vibration. Receiver operating characteristic (ROC) analysis will help assess the ability of spatiotemporal parameters to detect pathological vibration.
Materials and Methods
Spatiotemporal parameters of correlation length and entropy were extracted from high-speed videos of 124 subjects, 67 without vocal fold pathology and 57 with either vocal fold polyps or nodules. Mann-Whitney rank sum tests were performed to compare normal vocal fold vibrations to pathological vibrations, and ROC analysis was used to assess the diagnostic value of spatiotemporal analysis.
Results
A statistically significant difference was found between the normal and pathological groups in both correlation length ( P < .001) and entropy ( P < .001). The ROC analysis showed an area under the curve of 0.85 for correlation length, 0.87 for entropy, and 0.92 when the 2 parameters were combined. A statistically significant difference was not found between the nodules and polyps groups in either correlation length ( P = .227) or entropy ( P = .943). The ROC analysis showed an area under the curve of 0.63 for correlation length and 0.51 for entropy.
Conclusions
Although they could not effectively distinguish vibration of vocal folds with nodules from those with polyps, the spatiotemporal parameters correlation length and entropy exhibit the ability to differentiate normal and pathological vocal fold vibration and may represent a diagnostic tool for objectively detecting abnormal vibration in the future, especially in neurological voice disorders and vocal folds without a visible lesion.
1
Introduction
The ability to measure and observe vocal fold vibration is essential to diagnosing and understanding vocal fold pathologies. Much can be learned from acoustic measurement and aerodynamic evaluation of the voice, but the need to visualize the vocal folds is imperative. Visualization of the vocal folds allows us to determine the etiology of the change in the acoustic or aerodynamic measurement and delineate an effective treatment plan for the patient. Pathologies such as vocal fold paralysis, nodules, Reinke edema, and many others may be difficult to diagnose without the use of vocal fold visualization. In the present study, we focus on vocal fold nodules and polyps. Both nodules and polyps can be caused by vocal fold irritation or trauma and have roughly the same visual appearance . Discriminating between the 2 pathologies has typically been done on the basis of size, polyps being larger and typically unilateral and nodules being smaller and typically bilateral . It has been suggested by Colton et al that the 2 pathologies may share etiology because polyps may represent an advanced stage of nodules that have been continually irritated or traumatized. Through vocal fold visualization, the vibratory properties of the vocal folds can be used to understand the changes due to vocal fold pathology .
High-speed digital imaging (HSDI) allows for the visualization and analysis of individual vibrations of the vocal folds regardless of the presence of pathology or a periodicity. New methods of edge detection and video extraction have made the use of objective parameters from HSDI more clinically feasible than previous analysis methods that were too time consuming to perform as a part of routine practice. The edge detection algorithm proposed by Zhang et al allows vocal fold vibratory patterns to be extracted faster and more accurately than previous methods such as the histogram method and active contour . During glottal closure, a contrast no longer exists between the glottis and the surrounding tissues. As a result, neither the histogram nor the active contour methods can accurately distinguish surrounding tissue as the glottis. The method from Zhang et al does not experience this drawback. In addition, it reduces computation time while providing a more accurate portrayal of vocal fold vibration.
Spatiotemporal analysis of HSDI has recently been proposed as a valid method of extracting more information from high-speed digital images to describe vocal fold movement during phonation . As the name implies, spatiotemporal analysis extracts the dynamics of the vocal fold along its entire length as well as through time. This is in contrast to kymography where only a single line of pixels from a video is analyzed through time. Spatiotemporal analysis allows researchers to understand the interrelationships between different parts along the anterior-posterior axis of the vocal folds . Two relatively unexplored spatiotemporal parameters that provide important information about vocal fold vibration are correlation length and entropy. These 2 parameters were introduced by Zhang and Jiang and describe both the correlation of vocal fold vibration between the midline and all other points along the anterior-posterior axis and the amount of disorder present in the vibratory activity, respectively. Pathological voices typically have higher entropy values and lower correlation length values than healthy voices.
Traditional diagnosis of nodules and polyps has been performed using head and neck examination in conjunction with patient history and endoscopy . Both diseases manifest themselves in patients as chronic hoarseness and discomfort. Nodules are typically characterized by symmetric, bilateral epithelial swelling and decreased glottal closure as a result of increased vocal fold mass and interruption of vibration . Polyps are typically unilateral and show more pronounced disruption of phonation than nodules. Both pathologies are treated first with behavioral modification; and if improvement is not observed, surgical intervention is often the next step . Sulica and Behrman note that clinicians are more likely to treat polyps surgically than nodules. Because the 2 lesions are treated differently in some circumstances, it may be helpful to differentiate between them via analysis of high-speed videos. This differentiation would be most beneficial when reactive lesions develop contralaterally to a vocal fold polyp because it could be confused with nodules.
Differentiation of normal voices from pathological voices based on spatiotemporal parameters of vocal fold vibration represents a potential advance in diagnosis because it allows for the quantitative analysis of pathologies that have traditionally been diagnosed subjectively. Current clinical practice requires visualization of the vocal folds to identify a suspected vocal fold lesion. Therefore, obtaining high-speed video of the vibrating vocal folds for analysis would require only a minute increase in workload to the physician and no increased discomfort of the patient.
Little research has been dedicated to a quantitative understanding of the spatiotemporal changes inherent in pathological larynges. Several studies using excised canine larynx setups found that pathological larynges have higher entropy values and lower correlation length values than normal larynges . In our experiment, we seek to evaluate the utility of spatiotemporal analysis as a tool clinicians can use to distinguish between normal and pathological vocal folds in humans. Receiver operating characteristic (ROC) analysis of the spatiotemporal parameters correlation length and entropy will assess their diagnostic potential. These parameters may increase the level of objectivity in laryngeal pathology diagnosis, which may, in turn, increase the quality of patient care.
2
Materials and methods
A total of 124 subjects, 78 females and 46 males ranging from age 16 to 75 years with a mean age of 43 years, were used in this study. Of the 78 female subjects, 20 had vocal fold nodules, 14 had a unilateral polyp, and 44 had normal vocal folds. Of the 46 male subjects, 0 had vocal fold nodules, 23 had a unilateral polyp, and 23 had normal vocal folds. Diagnoses were made by an attending physician and were based on the subject’s medical history and an endoscopic examination of the vocal folds. This study was conducted under the approval of the Institutional Review Board of the University of Wisconsin-Madison and the Ethics Committee of the Fudan University Eye, Ear, Nose, and Throat Hospital.
A high-speed camera (KayPENTAX Fastcam MC2, Lincoln Park, NJ) was used to collect high-speed images of the vocal folds at a frame rate of 4000 frames per second and a resolution of 512 × 256 pixels. Images were obtained with a rigid 70° endoscope (Kay Elemetrics Model 9106) with a 300-W cold light source. The rigid laryngoscope was coupled to the high-speed digital camera head, and endoscopy was performed as in conventional videostroboscopy. The phonatory task was consistent for all recordings. Subjects produced an open vowel /i/ for 4 seconds at a comfortable effort. Figs. 1 and 2 display sequences of images from one cycle of vibration in normal and pathological voices, respectively.
A custom-designed MATLAB program (version 7.2.0.232 [R2006a], The Mathworks, Inc, Natick, MA) was used to crop the field of view in the videos to reduce the amount of visible superficial surrounding tissue. Sections of these videos (800–1000 frames in length) with minimal camera movement and sufficient lighting were chosen for automated edge detection. The MATLAB program uses a pixel threshold edge detection method to count the number of pixels in the glottis for each line of pixels perpendicular to the glottal axis in each frame. Because the lighting conditions change from recording to recording, an appropriate threshold for edge detection was determined for each video. In each frame, pixels with intensity greater than the threshold were considered to be tissue of the larynx illuminated by the light source; and those with subthreshold intensity were considered to be the glottis. A MATLAB program was designed to count the pixels composing the glottal width at each pixel line of each frame. The data are stored as a 2-dimensional matrix with the i -index as frame number, the j -index as anterior-posterior position (in pixels), and the glottal width as the elements. The data were visualized by plotting time and spatial position (anterior-posterior) on the x- and y-axes, respectively. Glottal width was color-mapped to the z-axis. Red denotes maximum glottal width, blue represents minimum glottal width, and intervening colors represent intermediate degrees of glottal width. Fig. 3 explains the features of a typical spatiotemporal plot of a single cycle of vibration in a normal subject. Numbers displayed in the figure refer to stages within the vibratory cycle shown in Fig. 1 . Stages 1 and 12 fall during the closed phase; and as a result, the spatiotemporal plot is dark blue at all spatial positions except the posterior glottal chink. Stages 4 and 10 occur during the early and late open phases, respectively. The light blue and green colors at these stages reflect the fact that glottal width is at an intermediate stage between maximum opening and maximum closure. Stage 7 falls at the maximum opening time of the vocal folds; and therefore, the spatiotemporal plot is red at most of the central spatial positions. From the 2-dimensional matrix, the spatiotemporal parameters of correlation length and entropy were calculated. Correlation length refers to the percentage of pixel lines perpendicular to the glottal axis whose pattern of opening and closing correlates with the vocal fold midline at a level of 90% or greater, as determined by the following calculations. Entropy refers to the amount of disorder present in the signal. Both parameters were defined previously by Zhang and Jiang . Selecting video samples with minimal camera movement and shadows is important for the accuracy of the spatiotemporal parameters of correlation length and entropy. Camera movement and shadows affect the pixel light intensity that plays an integral part in the determination of edges and glottal width. Because spatiotemporal parameters are calculated using glottal width and edge detection information, excessive camera movement and shadows can lead to inaccurate results.
Briefly, we calculated the cross-correlation function as:
where L g denotes the glottal length. i 1 and i 2 represent the 2 spatial points at which C max ( i 1 ) and C max ( i 2 ) are decreased to 0.9 with respect to the reference point. Correlation length L measures the size of the spatially correlated structure. A higher value of L corresponds to a larger size of the spatially ordered pattern. Data with complete spatial consistency has L = 1; however, the correlation length of random spatiotemporal data approaches zero because any 2 spatial points are uncorrelated. To further quantify the spatiotemporal complexity of the vocal fold vibrations, we apply eigenmode analysis via Karhunen-Loeve decomposition, which decomposes the input data of a spatially extended system into an orthonormal set of eigenmodes. For the vibratory signal u ( j , t ) extracted with high-speed digital imaging, we can calculate the spatial covariance matrix as:
where i , j = 1, 2, …, N is the spatial index. C ij is a symmetric matrix whose eigenvalue λ j and eigenvector j satisfy CQ j = λ j j . The eigenvalue λ j measures the energy captured by the corresponding eigenvectors j . The relative energy E k of the k -theigenmode can be described as E k = λ k /∑ j = 1 N λ j , and the global entropy S can be calculated as:
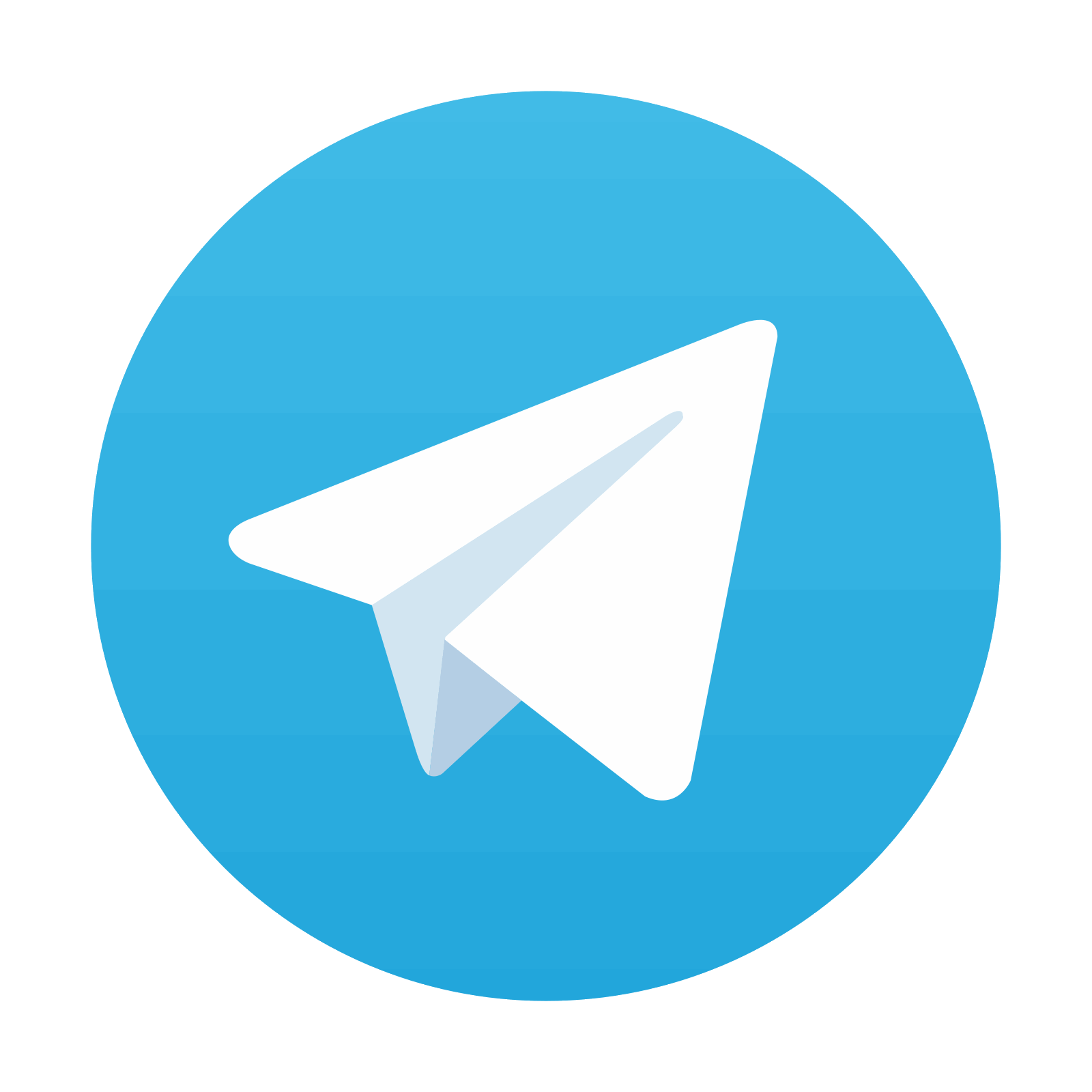
Stay updated, free articles. Join our Telegram channel

Full access? Get Clinical Tree
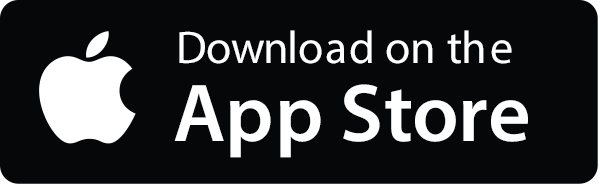
