Purpose
To perform a quantitative analysis of choriocapillaris (CC) flow deficits (FDs) in patients with uveitis.
Design
Retrospective cross-sectional study.
Methods
Swept-source optical coherence tomography based angiography (SS-OCTA) macular volume scans (3 × 3 mm and 6 × 6 mm) were obtained using the Plex Elite 9000. En face CC images were generated and analyzed using an automated FD identification algorithm. Three quantitative metrics were determined for each eye: FD number (FDN), mean FD size (MFDS), and FD density (FDD). Quantitative metrics were compared between uveitis and control eyes. The uveitis cohort was further subdivided by the presence or absence of choroidal involvement, and quantitative metrics were compared between subgroups and normal control subjects.
Results
A total of 38 eyes from 38 control subjects and 73 eyes from 73 uveitis subjects were included in this study. Eyes with uveitis have significantly larger CC MFDS (3- × 3-mm scans; P < .0001; 6- × 6-mm scans; P < .0001) and higher FDD ( P = .0002; P = .0076, respectively) compared to control eyes. Additional analysis determined that these differences were due to the choroidal disease subgroup, which demonstrates significantly larger MFDS (3 × 3 = 1,108 μm 2 ; 6 × 6 = 1,104 μm 2 ) compared to both normal control eyes (752 μm 2 ; P < .0001; 802 μm 2 ; P < .0001, respectively) and uveitis patients without choroidal involvement (785 μm 2 ; P < .0001; 821 μm 2 ; P < .0001, respectively). No significant differences were found between the quantitative metrics of control subjects and patients without choroidal involvement.
Conclusions
Automated quantification of CC can identify pathological FDs and provide quantitative metrics describing such lesions in patients with uveitis. Posterior uveitis patients have significantly larger CC FDs than patients with other forms of uveitis.
Highlights
- •
Automated analysis of en face swept-source optical coherence tomography images identifies significant differences in choriocapillaris blood flow between patients with posterior uveitis and control subjects, by using 3 quantitative metrics.
Optical coherence tomography angiography (OCTA) is a noninvasive imaging modality that has demonstrated utility in studies of retinal vasculature disease. In patients with uveitis, including intermediate, posterior, and panuveitis, spectral domain OCTA (SD-OCTA) has identified both qualitative and quantitative retinal vascular flow abnormalities. , , However, in certain types of uveitis, such as birdshot chorioretinopathy or punctate inner choroidopathy, pathology may also involve or be entirely limited to the choroid and choriocapillaris (CC). , , , Although case reports and case series using SD-OCTA have identified CC flow abnormalities in patients with some forms of posterior uveitis, the vascular beds below the retinal pigmented epithelium (RPE) complex have not been thoroughly investigated, and quantitative analysis of CC abnormalities is limited.
The emergence of swept-source OCTA (SS-OCTA) technology allows for more in-depth characterization of choroidal blood flow in healthy and diseased eyes. SS-OCTA imaging can provide improved visualization of blood flow in the choroid and CC due to better depth penetration through the RPE/Bruch’s membrane (BM) complex and lower sensitivity roll-off than SD-OCT systems. Additionally, acquisition speeds of more than 100 kHz facilitate wide angle imaging, a feature important for diseases such as uveitis that frequently manifest clinically significant lesions in the extrafoveal macula. Finally, segmentation of SS-OCTA data into choroidal or CC en face slabs is showing promise for the detection of inflammatory disease activity without a need for fluorescein or indocyanine green based angiography. , In order to bring these benefits of SS-OCTA imaging into more widespread clinical use, image acquisition will need to be coupled with automated image analysis for the rapid, reproducible, and quantitative detection of relevant disease associated abnormalities. This study tested an automated en face CC OCTA image analysis approach for the ability to detect and quantify CC in patients with uveitis.
Subjects and Methods
This single-institution retrospective cross-sectional study was approved by the Institutional Review Board at the University of Washington. Written informed consent regarding the nature of this research study was obtained for all subjects before imaging. This study was performed in accordance with the tenets of the Declaration of Helsinki and the Health Insurance Portability and Accountability Act of 1996.
Study Population
Patients 18 years or older, with a diagnosis of anterior, intermediate, posterior, panuveitis, or retinal vasculitis were recruited for SS-OCTA imaging between August 2016 and July 2018 at the University of Washington Harborview Medical Center Eye Institute. Patients with active and inactive uveitis were recruited. Upon entry in the study, subject data including sex, age, uveitis diagnosis, and involvement of 1 or both eyes were collected. Anatomic location of uveitis and determination of location of disease activity were diagnosed by using the criteria established by the standardization of uveitis nomenclature criteria based on clinical examination and additional imaging modalities such as color fundus, fluorescein angiography, indocyanine green angiography, SD-OCT, and fundus autofluorescence at the discretion of the examining physician. Results of laboratory testing, chest radiographs, biopsy, or magnetic resonance imaging, when performed, were used to further classify uveitis diagnosis by underlying cause.
Image Acquisition and Scanning Protocols
Research imaging was performed using a 100-kHz SS-OCTA Plex Elite 9000 (Carl Zeiss Meditec, Dublin, California), with a central wavelength of 1,060 nm, a bandwidth of 100 nm, an axial resolution of ~6 μm, and a lateral resolution of ~20 μm in retinal tissue. FastTrac (Carl Zeiss Meditec, Dublin, California) motion tracking was used during all scans to minimize possible motion artifacts during imaging. Volume scans of 3 × 3 mm and 6 × 6 mm centered on the fovea were obtained for both eyes of all subjects. For unilateral cases, the diseased eye was selected for further analysis, and for bilateral cases, the eye with the highest signal strength was selected. If both eyes were equal in signal strength, then the right eye was used. Images with severe motion artifacts, macular edema, and signal strength lower than 7 were excluded from further analysis.
The commercially available Plex Elite algorithm was used to segment the CC and generate en face CC flow images. Briefly, the RPE best fit line was determined using Plex Elite software, and manual corrections were performed in the case of failed automated segmentation. The CC was defined as the region from 16 to 31 μm below the RPE. The complex optical microangiography (OMAG c ) algorithm was used to generate OCTA volumes, and then maximum projection was applied to the segmented CC OCTA volumes to generate the en face images. After CC en face images were acquired, a previously published compensation strategy using structural OCT information was also applied using Matlab version R2016b software (MathWorks, Natick, Massachusetts) to correct the OCTA flow in the CC images for signal attenuation. Quantitative analysis was then performed of the en face image.
Definition and Quantification of CC FD
In this study the term flow deficit (FD) is used to describe areas where there is a lack of flow or the flow is below the detectable threshold of OCTA. , In normal control subjects, these areas are believed to represent the CC vascular walls and intercapillary spaces. In disease states, these areas are believed to represent choroidal perfusion or the presence of choroidal infiltrates leading to blood flow that is below the OCTA detection sensitivity. The similar term flow void has been used in other reports and is well described in the medical literature. FDs were defined on each en face image by using a customized algorithm through Matlab version R2016b (MathWorks), using a comprehensive thresholding strategy. This method is summarized in Figure 1 . Briefly, for each en face CC slab, a complex thresholding algorithm that used fuzzy C-means clustering was applied. Pixels that self-clustered into the lowest intensity group were segmented as the initial FDs. The image was then binarized such that areas of CC flow were bright, and the areas of absent flow (FDs) are dark ( Figure 1 , B and F). The final corrected FD map was generated after masking out areas of projection artifacts from overlying retinal vasculature and removing FDs that were subphysiological in size (i.e., less than the normal intercapillary distance) ( Figure 1 , C and G). Finally, quantitative analysis was performed using the corrected map.

Three quantitative metrics defined for each image were FD number (FDN), mean FD size (MFDS), and FD density (FDD) ( Figure 1 , J). FDN was defined as the total number of all individual FDs identified per image. MFDS was defined as the mean size (square micrometers [μm 2 ]) of all individual FDs identified per image. FDD was defined as the total area occupied by CC FDs-to-total image area ratio (minus projection artifacts).
Determination of Coefficient of Variation
Five control subjects and 5 subjects with posterior uveitis were scanned 3 times at the same visit by using both the 3- × 3-mm scans and the 6- × 6-mm scans. Three of the posterior uveitis patients had a diagnosis of birdshot chorioretinopathy, and the other two had a diagnosis of serpiginous choroiditis. Repeatability was calculated for each of the quantitative metrics, FDN, MFDS, and FDD, and reported as the coefficient of variation (CV).
Statistical Analysis
Statistical analyses were performed using Matlab R2016b software (MathWorks) and Prism software (GraphPad, San Diego, California). Cohort data were expressed as the median and interquartile range (IQR). The Mann-Whitney U test and Kruskal-Wallis test were used to compare control and uveitis cohorts; P values less than .05 were considered significant in the case of multiple comparisons; Bonferroni correction was performed such that P values less than .05/n were considered significant, where “n” was the number of comparisons made. Nonparametric Spearman correlation was used for correlation testing of groups with non-normal distributions. The receiver operating characteristic (ROC) curve was plotted, and the area under the curve (AUC) was calculated to compare the diagnostic power of each metric.
Results
A total of 78 uveitis subjects and 38 control subjects were recruited and imaged. Five uveitic subjects were excluded, 3 because of the presence of macular edema and 2 because of insufficient scan quality. No control subjects were excluded. For the remaining subjects, both eyes of 73 uveitis patients and 38 control subjects were imaged and examined. One eye per subject was selected for further analysis as (see Subjects and Methods). The average age of uveitis patients was 49 years old and the majority were women (71%). Similar age and sex distribution were present in the control group ( Table 1 ). In the uveitis group, the majority of patients had diagnoses of posterior uveitis (n = 40; 54.8%), but patients with anterior uveitis (n = 20; 27.4%) and intermediate (n = 8; 11.0%) and panuveitis (n = 5; 6.8%) were also represented.
Control | Uveitis | P Value | ||
---|---|---|---|---|
Number | 38 | 73 | NA | |
Mean ± SD age, y (range) | 54 ± 22 (32-76) | 49 ± 15 (22-75) | .221 | |
Females | 53% | 71% | .057 | |
Anatomic classification | Anterior | 20 | NA | |
Intermediate | 8 | NA | ||
Posterior | 40 | NA | ||
Panuveitis | 5 | NA |
CC FD Analysis in Uveitis and Healthy Controls
A total of 222 en face CC images were generated from the 3- × 3-mm and 6- × 6-mm scans of the 111 study eyes. Representative CC en face slabs from control subjects and uveitis subjects are shown in Figure 2 . The FD maps for control subjects reveal numerous, small, relatively evenly spaced FDs throughout the macula. Images from uveitis patients demonstrated a range of findings. For example, images from patients with anterior and intermediate uveitis lacked qualitative lesions and appeared similar to images from control subjects ( Figure 2 , E through H). In contrast, in patients with posterior uveitis, abnormalities of the FD map were grossly apparent. In a patient with Vogt-Koyanagi-Harada disease, multiple enlarged FDs can be appreciated on both the 3- × 3-mm and 6- × 6-mm images ( Figure 2 , I through L). An additional example is provided by a patient with multifocal choroiditis that demonstrates large areas of abnormal flow signal consistent with widespread loss of the CC ( Figure 2 , M through P).

To determine the differences between control and uveitic eyes quantitatively, the 3 CC flow metrics, FDN, MFDS and FDD, were compared between the control and uveitis groups. Prior to intergroup comparisons, metric repeatability was established by using a subset of both control and uveitis images. CVs ranged from 1.29% to 6.65% ( Table 2 ), with MFDS having the lowest CV among the 3 metrics ( Table 2 ). The summary of the results for each metric in the uveitis and normal control images are presented in Table 3 . In control eyes, the median FDNs were 417 (Q 3 −Q 1 : 123) per 3- × 3-mm image and 2,365 (Q 3 −Q 1 : 681) per 6- × 6-mm image. No significant differences in FDN were identified between control and uveitis patients. In control subjects, the median MFDS was 751.7 μm 2 (IQR: 69.6 μm 2 ) per 3- × 3-mm image and 802.0 μm 2 (IQR: 42.1 μm 2 ) per 6- × 6-mm image. In uveitis images, the median MFDS was significantly larger than that of control subjects in both the 3- × 3-mm scans (median: 838.4 μm 2 ; IQR: 310.2 μm 2 ; P < .0001) and the 6- × 6-mm scans (median: 870.9 μm 2 ; IQR: 274.1 μm 2 ; P < .0001). Uveitis patients also demonstrated a significantly higher FDD than that in control subjects (3- × 3-mm scans, P = .0002; 6- × 6-mm scans, P = .0076). Thus, this analysis revealed that CC FDs were larger and more densely clustered in the uveitis patients than in control subjects.
CV | FDN | MFDS (μm 2 ) | FDD |
---|---|---|---|
3 × 3 mm Control | 5.06% | 2.58% | 4.17% |
3 × 3 mm Uveitis | 6.57% | 5.57% | 6.65% |
6 × 6 mm Control | 3.85% | 1.29% | 3.79% |
6 × 6 mm Uveitis | 5.00% | 4.29% | 4.72% |
Median (IQR) | 3 × 3 mm | 6 × 6 mm | ||||
---|---|---|---|---|---|---|
FDN | MFDS (μm 2 ) | FDD | FDN | MFDS (μm 2 ) | FDD | |
Control | 417 (123) | 751.74 (69.64) | 0.037 (0.008) | 2365 (681) | 802.02 (42.13) | 0.058 (0.013) |
Uveitis | 442 (141) | 838.36 (310.25) | 0.042 (0.023) | 2323 (829) | 870.93 (274.14) | 0.063 (0.018) |
Mann-Whitney U test | P = .1061 | P < .0001 a | P = .0002 a | P = .6917 | P < .0001 a | P = .0076 a |
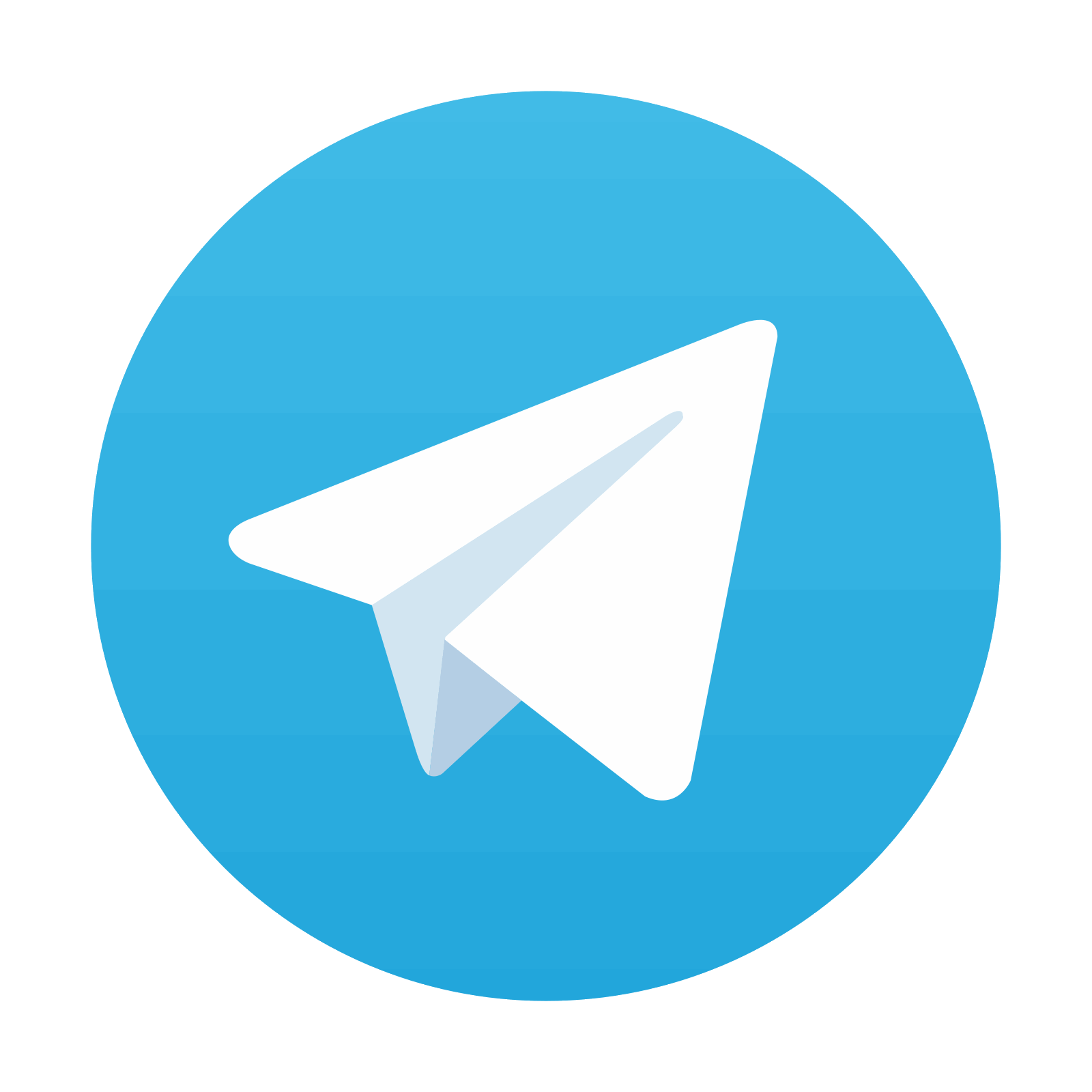
Stay updated, free articles. Join our Telegram channel

Full access? Get Clinical Tree
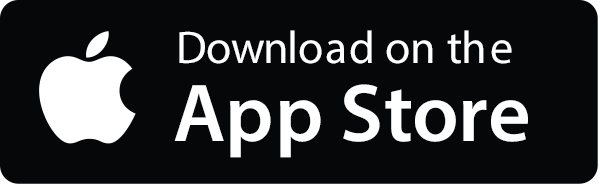

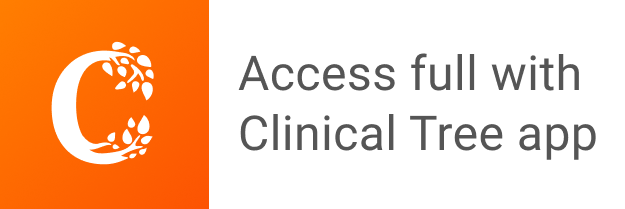