PURPOSE
To generate a prognostic model to predict keratoconus progression to corneal crosslinking (CXL).
DESIGN
Retrospective cohort study.
METHODS
We recruited 5025 patients (9341 eyes) with early keratoconus between January 2011 and November 2020. Genetic data from 926 patients were available. We investigated both keratometry or CXL as end points for progression and used the Royston-Parmar method on the proportional hazards scale to generate a prognostic model. We calculated hazard ratios (HRs) for each significant covariate, with explained variation and discrimination, and performed internal-external cross validation by geographic regions.
RESULTS
After exclusions, model fitting comprised 8701 eyes, of which 3232 underwent CXL. For early keratoconus, CXL provided a more robust prognostic model than keratometric progression. The final model explained 33% of the variation in time to event: age HR (95% CI) 0.9 (0.90-0.91), maximum anterior keratometry 1.08 (1.07-1.09), and minimum corneal thickness 0.95 (0.93-0.96) as significant covariates. Single-nucleotide polymorphisms (SNPs) associated with keratoconus (n=28) did not significantly contribute to the model. The predicted time-to-event curves closely followed the observed curves during internal-external validation. Differences in discrimination between geographic regions was low, suggesting the model maintained its predictive ability.
CONCLUSIONS
A prognostic model to predict keratoconus progression could aid patient empowerment, triage, and service provision. Age at presentation is the most significant predictor of progression risk. Candidate SNPs associated with keratoconus do not contribute to progression risk.
K eratoconus is a common corneal ectasia that causes irregular astigmatism, scarring, and loss of vision. Thinning and steepening can progress through childhood and early adulthood, but the shape of most eyes stabilizes by the third or fourth decade. Without intervention, keratoconus can lead to severe visual loss, with approximately 10% of eyes eventually requiring corneal transplantation. Corneal crosslinking (CXL) by topical application of riboflavin, followed by irradiation with UV-A light, can arrest progression of keratoconus in up to 88% to 100% of eyes even when there is relatively advanced disease. The potential benefit of CXL is to prevent visual deterioration with a relatively low risk procedure that is cost effective for health care providers.
However, CXL is usually not offered to all patients at presentation because the disease may have already stabilized. In the recent KERALINK study, 43% of children aged <17 years at presentation had not progressed after 18 months. The definition of progression also varies with the severity of keratoconus, but for early disease a common threshold is either an increase in the maximum keratometry (Kmax) of >1 diopter (D), a change in the manifest refractive spherical equivalent of >0.50 D, or an increase in manifest refractive cylinder of >1 D. , Depending on the rate of progression this threshold may be passed in a few months, years, or not at all. At the first assessment it can be a challenge to distinguish eyes that are at risk of rapid progression from those where it is safe to monitor. Unnecessary review visits are a burden to the patient and the care system.
We considered the date of numeric progression, as well as the date when CXL was performed, as alternative end points to define keratoconus progression. Although the use of keratometry as an end point may appear the more objective method, there is variability on the definition of progression reported in the literature and conclusions may vary with the definition that is adopted. Repeatability thresholds are not usually tailored to individual eyes (ie, an increase in Kmax by 1 D is not significant in all eyes) although there is growing evidence on the variability of measurements in more advanced disease and the need for tailoring numerical progression definitions to the disease state, and distinguishing real progression from inherent variability of measurement modalities.
Finally, patients who receive CXL prior to progression must be censored from the data set even though these eyes are likely to have been at risk of progression. This type of informative censoring creates a bias. In contrast, the time to CXL depends on several variables that include numeric disease progression, but also incorporates patient-specific risk factors for future progression. Its strength is that it is an easily comprehensible and meaningful end point for patients. It encompasses individual risk factors that are not considered when imaging is used in isolation and it has been used by others as defining the event of interest.
For these reasons, we have used demographic and serial tomography data from a large cohort of patients to generate a time-to-event model to predict the probability of an individual progressing to CXL. Because the Cox proportional hazards method does not generate smooth time-to-event curves, we used the Royston-Parmar model to achieve direction estimates of the hazard function. We also performed a further analysis of a subset of patients who had genetic data in the form of single-nucleotide polymorphisms (SNPs) generated as part of a study to determine keratoconus risk. 21
METHODS
COHORT
The study protocol was reviewed and approved by the Clinical Audit Assessment Committee of Moorfields Eye Hospital NHS Foundation Trust (reference CA17/CED/03). Institutional Review Board (IRB) approval was obtained, and individual patient consent was not required. The study conformed to the tenets of the Declaration of Helsinki. We identified from the Moorfields Eye Hospital electronic health record database (OpenEyes) patients aged ≥13 years diagnosed with clinical or suspected keratoconus who attended our Early Keratoconus Clinic between January 2011 and November 2020.
Clinical data included keratometry (Kmax, Front K1, Front K2, Back K1, Back K2), and pachymetry (minimum corneal thickness) captured by Scheimpflug tomography (Pentacam HR; Oculus GmbH). We only included scans with a quality score of “good” or “OK,” and where multiple scans were taken on the same day, we used the mean value. The date of all CXL procedures was recorded.
The protocol for offering CXL throughout the study period was as follows: (1) a documented history prior to referral to the Early Keratoconus Clinic of our hospital of significant recent disease progression, (2) a change in contemporary measurements of 95% above the repeatability limits of the baseline measurements as shown in Supplementary Table S1 (available at http://www.ajo.com ), or (3) a patient considered by a clinician to be at high risk of progression despite their not fulfilling the above 2 criteria. Exclusion criteria included pregnancy or breastfeeding, uncontrolled ocular surface disease, or a minimum corneal thickness less than 375 µm.
All the data used for model fitting started from the first appointment in the Early Keratoconus Clinic. Patient demographics included age, gender, smoking status (current or ex-/nonsmoker), ethnicity, and postcode. Ethnicity was coded as 1 for “Black” or “South Asian or South Asian British” and 0 for any other category (excluding missing values). Before model fitting, the pachymetry in micrometers was divided by 10 to generate a meaningful scale. For the primary analysis, eyes with any missing data were excluded. We also explored multiple imputation, which avoids data exclusion by generating multiple versions of the data set, with missing values replaced with values sampled from an appropriate distribution.
To see whether genetic data can help predict keratoconus progression, we used 28 candidate SNPs from a recent keratoconus genome-wide association study that contained 926 patients from Moorfields Eye Hospital. The SNP data were encoded as 0 (homozygous reference genotype), 1 (heterozygous genotype), or 2 (homozygous variant genotype). We chose to use an additive encoding; thus, the risk of disease increases additively with the degree of genetic variation. Anonymized data were then exported to Excel software for analysis (version 15.24 2016, Microsoft Corp).
MODEL FITTING AND COVARIATE SELECTION
A Royston-Parmar flexible parametric survival model was fitted to the data to predict the probability of an eye progressing to CXL. Initial analysis of the covariates was performed by univariate analysis using the same model characteristics as the multivariable model. When selecting covariates for the final multivariable model, we used backwards stepwise selection with a significance level of 0.05. We used linear covariates for ease of interpretation of our final model. To create a more parsimonious model we examined the effect on explained variation and discrimination of removing single variables from the model.
KERATOMETRIC PROGRESSION SENSITIVITY ANALYSIS
We included a sensitivity analysis in which we investigated keratometric progression as an alternative end point. Keratometric progression was defined using thresholds from Gore and associates. When using numerical thresholds to define progression, the appointments for eyes beyond the date of CXL cannot be used. However, censoring these eyes at the date of CXL represents informative censoring. Based on the recommendations of Clarke and associates for investigating the impact of informative censoring, we generated a “best case” data set where eyes were censored at the CXL date and a “worst case” data set where patients were assumed to progress at the CXL date.
The corresponding Kaplan-Meier curves were plotted to provide a visual comparison of the 2 data sets. A Royston-Parmar model was then fitted on both data sets. We used the same techniques (backward stepwise selection, significance level of .05) as described in the previous section to fit the model and compare the explained variation and hazard ratios.
MULTIVARIABLE MODEL VALIDATION
We validated the model using internal-external cross validation in which we split the data set by geographical region. , For the k th region, the model is fitted on the full data set excluding region k , and then Kaplan-Meier curves and predicted survival curves were generated for region k . Seven geographical regions were created based on the patient’s postcode as shown in Supplementary Figure S1 (available at http://www.ajo.com ).
To quantitatively assess the validation, Royston and Sauerbrei’s D statistic was calculated for both the model fitted from data excluding region k ( D ( k ) ) and also the model applied to region k ( D k ). The difference between these 2 discrimination metrics ( D k – D ( k ) ) was calculated with its corresponding standard error to assess the predictive ability of the model. To demonstrate how the model could be used in practice, we include 3 hypothetical patients’ eyes with different progression risk profiles (high, medium, low risk) and plot the predicted time-to-event curve for each shown in Figure 2 .
STATISTICAL ANALYSIS
The event of interest was defined as the date that the eye underwent CXL. We calculated the time-to-event as the difference between the first appointment in our service and the date of CXL (or the last patient appointment in the case of censoring). Because we had paired observations (eyes), we used variance-corrected models to account for correlation between eyes and to ensure that robust SEs were produced. The choice of scale and selection of degrees of freedom for the Royston-Parmar model was informed by inspecting the Akaike information criterion and Bayes information criterion, and the results of this were balanced with ease of interpretation. See Supplementary Table S2 and Supplementary Material S1 (available at http://www.ajo.com ) for further explanation.
Royston and Sauerbrei’s D statistic was used as a measure of discrimination and R 2 D as a measure of explained variation (both calculated on the natural scale of the model). Although all of the primary results were generated from a complete case analysis, we performed an additional analysis using multiple chained imputation (predictive mean matching approach with 5 nearest neighbors). Model fitting was performed in Stata 13 (StataCorp LP), and the Royston-Parmar model was fitted using the stpm2 package from Stata 13.
RESULTS
COHORT
From a potential of 9341 eyes (4316 pairs of eyes and 709 individual eyes), the final model used 8701 eyes of 4823 patients, with 3232 eyes that had CXL. The mean age was 28.3 years with SD of 7.1 years. We excluded 640 eyes with missing data. Table 1 summarizes the available covariates along with missing data percentages. See Supplementary Material S2 and Supplementary Table S3 (available at http://www.ajo.com ) for a description of the multiple imputation results.
Covariate | Type | Mean | SD | No. of Eyes | Missing No. (%) |
---|---|---|---|---|---|
Front K1 (D) | Numeric | 45.31 | 3.86 | 8813 | 528 (5.7) |
Front K2 (D) | Numeric | 48.39 | 4.85 | 8839 | 502 (5.4) |
Back K1 (D) | Numeric | –6.53 | 0.75 | 7949 | 1392 (14.9) |
Back K2 (D) | Numeric | –7.23 | 0.93 | 8702 | 639 (6.8) |
Kmax (D) | Numeric | 54.14 | 8.01 | 8834 | 507 (5.4) |
Pachymetry (μm) | Numeric | 462.92 | 46.15 | 8946 | 395 (4.2) |
Age (y) | Numeric | 28.28 | 7.10 | 9341 | 0 (0) |
Genetic data a | Ordinal | N/A | N/A | 1141 | 8020 (85.9) |
Self-reported Black or Asian ethnicity b | Categorical (59.9% Black or Asian) | N/A | N/A | 4889 | 4452 (47.7) |
Male gender | Categorical (67% male) | N/A | N/A | 9341 | 0 (0) |
Smoker c | Categorical (4.5% smoker) | N/A | N/A | 9341 | 0 (0) |
a Genetic data composed of 28 SNPs and was encoded in an additive fashion (0, 1, 2).
b 1 = Black or Asian, 0 = otherwise.
MODEL FITTING AND COVARIATE SELECTION (GENETIC DATA)
We analyzed patients with genetic data separately because these data were only available for ∼14% of patients. Of 926 patients (1852 eyes) with genetic data, 531 eyes were excluded with incomplete keratometry or CXL data, which left 1321 eyes, of which 665 had CXL. With univariate analysis of the 28 SNPs, only rs72631889 was found to be significant ( P = .01) (Supplementary Table S4 [available at http://www.ajo.com ]). We then produced a multivariable model via backward selection on this subset of eyes using corneal data, patient data, and rs72631889 as an additional covariate as shown in Supplementary Table S5 (available at http://www.ajo.com ). However, rs72631889, although significant ( P = .005), had a negligible contribution (0.3%) to the explained variation in the final model.
MODEL FITTING AND COVARIATE SELECTION (EXCLUDING GENETIC DATA)
The results of the univariate time-to-event analysis on the hazards scale using a Royston-Parmar flexible parametric model is shown in Table 2 . Genetic data was excluded from this analysis. All variables except smoking status were significant. The explained variation ( R 2 D ) and discrimination ( D ) were highest for age (17%) and Kmax (15%) with Front K1, Front K2, Back K1, Back K2, and pachymetry each explaining 6% to 10% of the variation. Notably, gender and ethnicity, although significant in the univariate analysis, did not contribute to explained variation. The hazard ratios for significant covariates indicate that increasing age at presentation, greater pachymetry, and flatter (less negative) posterior keratometry values decrease the risk of having CXL, whereas steeper anterior keratometry values and male gender increase the risk of having CXL.
Univariable XXXX (no. of eyes = 9341) | Multivariable XXXX (no. of eyes = 8701) | |||||
---|---|---|---|---|---|---|
Covariate | Hazard Ratio (95% CI) | P Value | R 2 D (%) | D | Hazard Ratio (95% CI) | P Value |
Ethnicity | 1.14 (1.02, 1.27) | .02 | 0.4 | 0.13 | N/A | N/A |
Smoker a | 1.07 (0.9, 1.28) | .46 | 0.1 | 0.05 | N/A | N/A |
Male gender | 1.11 (1.01, 1.21) | .02 | 0.2 | 0.10 | N/A | N/A |
Age at presentation | 0.91 (0.9, 0.92) | <.001 | 16.7 | 0.92 | 0.9 (0.90, 0.91) | <.001 |
Kmax | 1.06 (1.05, 1.06) | <.001 | 14.9 | 0.86 | 1.08 (1.07, 1.09) | <.001 |
Front K1 | 1.09 (1.08, 1.1) | <.001 | 7.0 | 0.56 | 0.93 (0.91, 0.94) | <.001 |
Front K2 | 1.08 (1.07, 1.08) | <.001 | 9.8 | 0.67 | N/A | N/A |
Back K1 b | 0.67 (0.64, 0.71) | <.001 | 5.9 | 0.51 | N/A | N/A |
Back K2 b | 0.7 (0.67, 0.72) | <.001 | 8.4 | 0.62 | N/A | N/A |
Pachymetry 10 b | 0.93 (0.92, 0.94) | <.001 | 7.5 | 0.58 | 0.95 (0.93, 0.96) | <.001 |
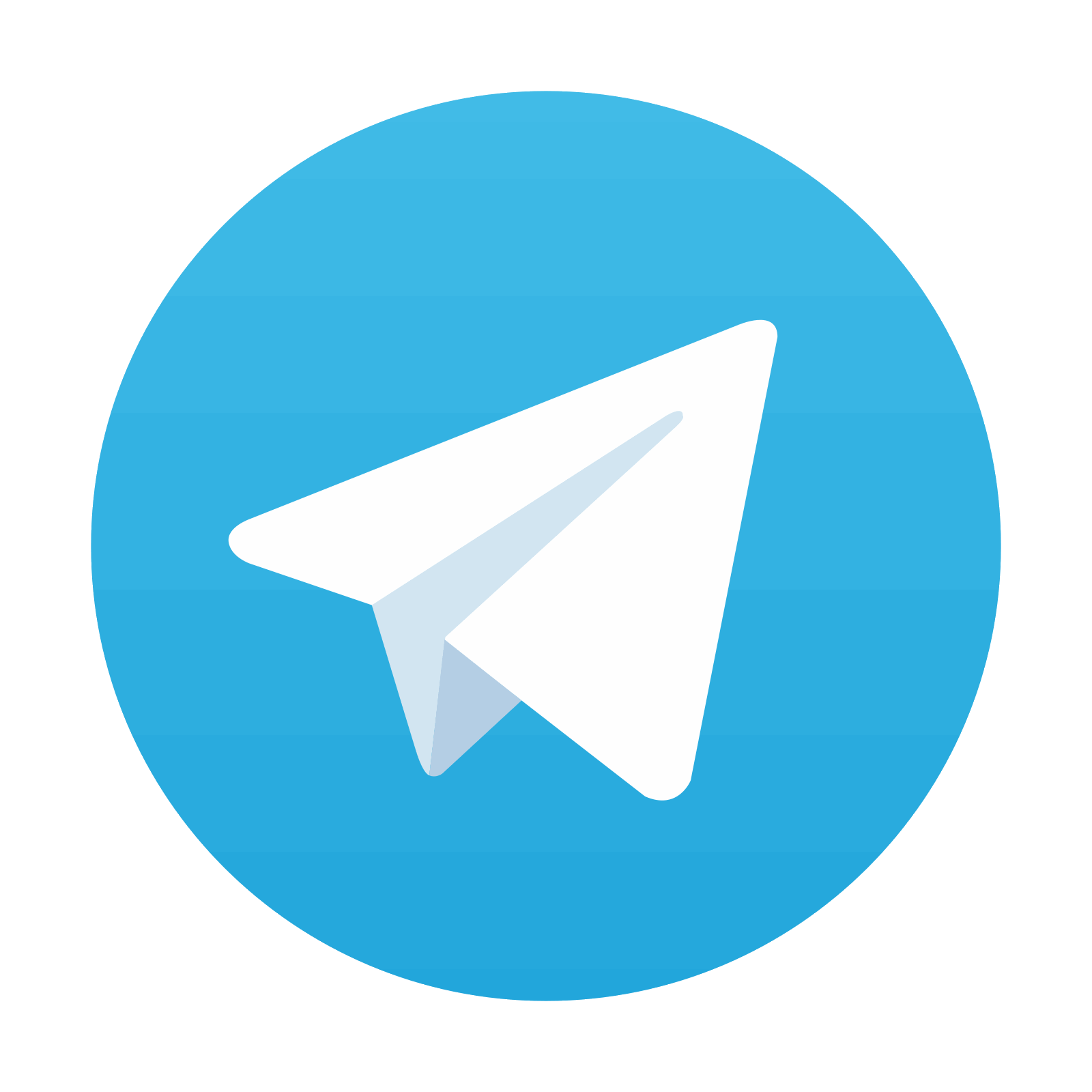
Stay updated, free articles. Join our Telegram channel

Full access? Get Clinical Tree
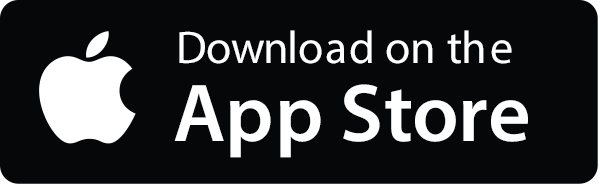

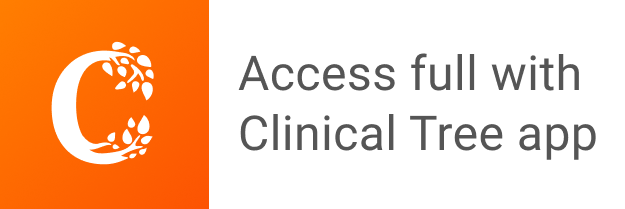