Purpose
To apply computational methods to model normal age-related changes in corneal parameters and to establish their association with demographic factors, thereby providing a framework for improved detection of subclinical corneal ectasia (SCE).
Design
Cross-sectional study.
Methods
One hundred seventeen healthy participants were enrolled from Centre for Eye Health (Sydney, Australia). Corneal thickness (CT), front surface sagittal curvature (FSSC), and back surface sagittal curvature (BSSC) measurements were extracted from 57 corneal locations from 1 eye per participant using the Pentacam HR. Cluster analyses were performed to identify locations demonstrating similar variations with age. Age-related changes were modeled using polynomial regression with sliding window methods, and model accuracy was verified with Bland–Altman comparisons. Pearson correlations were applied to examine the impacts of demographic factors.
Results
Concentric cluster patterns were observed for CT and FSSC but not for BSSC. Sliding window analyses were best fit with quartic and cubic regression models for CT and FSSC/BSSC, respectively. CT and FSSC sliding window models had narrower 95% limits of agreement compared with decade-based models (0.015 mm vs 0.017 mm and 0.14 mm vs 0.27 mm, respectively), but were wider for BSSC than decade-based models (0.73 mm vs 0.54 mm). Significant correlations were observed between CT and astigmatism ( P = .02-.049) and FSSC and BSSC and gender ( P = <.001-.049).
Conclusions
The developed models robustly described aging variations in CT and FSSC; however, other mechanisms appear to contribute to variations in BSSC. These findings and the identified correlations provide a framework that can be applied to future model development and establishment of normal databases to facilitate SCE detection.
The cornea forms the primary refractive surface of the eye and plays a pivotal role in maintaining clear focus at the retina. Keratoconus, a progressive condition that is characterized by corneal protrusion and thinning, can therefore result in significant visual impairment. Early detection of keratoconus is essential to identify individuals who are at risk of developing visual symptoms and those who are unsuitable for refractive surgery. However, the earliest stage of keratoconus, sometimes termed “forme fruste” or subclinical corneal ectasia (SCE), remains difficult to differentiate from normal corneas, even with the advent of advanced corneal imaging techniques. This is likely related to significant interindividual variation in corneal parameters within the normal population, with the wide range of normative values contributing to considerable overlap between normal and SCE populations.
Normal, age-related changes of corneal thickness (CT) and curvature (CC) are currently not well characterized, with several studies reporting relative corneal thinning and flattening with increasing age and others having found no such correlations. Similarly, consensus on the existence of systematic correlations between corneal parameters and other demographic factors, such as gender, ethnicity, and refractive error, have not been established. Measurement noise as a result of normal interindividual variability is once again likely to contribute to these inconsistencies because it may affect the ability of statistical tests to produce replicable and meaningful analyses. By clarifying if and how corneal parameters vary with age and other demographic factors, a narrower range of expected normative values could be generated given individual-specific demographic information, which may then facilitate the identification of measurements outside of normal limits and improve detection of SCE.
Cluster analysis methods have demonstrated success in minimizing interindividual variability via pooling of individual data points displaying statistically indistinguishable characteristics. They have been applied in various areas of vision science, including the characterization of aging changes in the macular ganglion cell layer, the identification of visual field isocontours, the optimization of indices to improve detection of glaucomatous visual fields, and the characterization of glaucomatous progression patterns. Given the ability of cluster analyses to meaningfully describe normative biologic data by reducing or redistributing measurement noise, applying these principles to corneal measurements may aid in clarifying the patterns of age-related change.
The present study used cluster methods to identify corneal locations showing similar changes in CT and CC with age in a normal cohort. From our understanding of corneal variations with increasing eccentricity and our previous work on macular ganglion cell layer aging, we hypothesize that normal corneal aging follows similar concentric patterns that may be revealed using a cluster analysis approach. The investigated cluster patterns facilitated the development of regression models to identify patterns of age-related change in corneal parameters, and we sought to validate these models to confirm their robustness. We also examined systematic correlations between CT and CC and demographic factors. This work will improve our understanding of age-related corneal changes and the impacts of demographic factors on variations in corneal measurements, thereby aiding in the establishment of normal corneal databases and future model development and has the potential to be applied to algorithms for improved detection of SCE.
Methods
Participant Recruitment
This was a cross-sectional study where data were retrospectively collected from patients attending the Centre for Eye Health (CFEH) in Sydney, Australia for corneal assessment or anterior eye imaging from January 2017 to December 2017. CFEH is an intermediate-tier, referral-based clinic primarily servicing patients referred by community optometrists in the metropolitan Sydney region. The clinical protocol for corneal assessment at CFEH includes thorough ocular and medical histories, visual acuity measurement with habitual spectacle correction, slit-lamp examination, Pentacam HR Scheimpflug imaging (Oculus, Wetzlar, Germany), Medmont E300 corneal topography (Medmont, Nunawading, Australia), Irx3 wavefront aberrometry (Imagine Optic, Orsay, France), and Cirrus or Spectralis anterior optical coherence tomography (Carl Zeiss Meditec, Inc., Dublin, California, USA, and Heidelberg Engineering, Heidelberg, Germany). Refractive error information was obtained from referral data, while self-reported demographic information and medical history were extracted from intake forms completed before the clinical examination. Signed consent to use clinical and demographic data for research purposes was obtained for each participant as per the ethics protocols approved by the University of New South Wales Australia Human Research Ethics Advisory panel, and this study adhered to the tenets of the Declaration of Helsinki.
The following criteria determined eligibility for inclusion in this study: absence of corneal ectasia in both eyes confirmed by 2 experienced clinicians based on clinical examination and normal imaging results, including corneal topography and tomography scans of adequate quality that did not show patterns suggestive of ectasia, visual acuity better than 20/25 (logMAR >0.1), spherical equivalent refractive error between +3.00 diopters (D) and −6.00 D and refractive astigmatism <2.00 D, for participants ≤60 years of age based on the critical age range for the detection of ectatic changes. Suitable threshold values for summary parameters, such as the Belin/Ambrósio enhanced ectasia D value, to distinguish normal and SCE eyes with high sensitivity and specificity, are yet to be established in the literature, and therefore we did not rely on such parameters in isolation to confirm absence of keratoconus. In addition, corneal astigmatism is an instrument-specific metric and corneal topography and tomography scans were manually inspected in a holistic manner for signs suggestive of ectasia, and therefore corneal astigmatism was not identified as an individual inclusion/exclusion criterion in addition to refractive astigmatism. Exclusion criteria were defined as any history of contact lens wear or ocular surgery, a history of diabetes or other systemic neuropathies, current pregnancy or hormone therapy, including contraceptive pill and hormone replacement therapy, and an unavailable complete medical history.
Pentacam Measurements
Corneal measurements were obtained from the Pentacam HR. For eligible participants, scan quality was manually assessed by an experienced clinician (J.T.); those that did not show an OK quality score according to the Examination Quality Specifications provided by the instrument review software (Pentacam Version 6.08r2; Oculus) or with data missing as a result of imaging artefacts, most commonly shadowing from eyelids, were excluded from analyses. One eye per participant was included at random where both eyes met inclusion criteria, otherwise the eye meeting inclusion criteria was included in this study.
CT and CC maps were directly exported from the instrument review software. CT, front surface sagittal curvature (FSSC), and back surface sagittal curvature (BSSC) measurements were extracted from the default 57-point locations on the “large color map” setting according to the instrument review software, covering a total central corneal diameter of 8.00 mm ( Figure 1 ). While Montalbán and associates reported significant correlations between mean front and back corneal radius in normal eyes, similar relationships have not been established for pointwise measurements, and given the importance of the posterior corneal surface in early detection of corneal ectasia we chose to consider BSSC measurements obtained in addition to FSSC measurements. FSSC and BSSC were chosen over corneal elevation measurements because they do not rely on reference sphere calculations that may vary between examinations. All left eye scans were transposed to right eye format by horizontally transposing data across the vertical midline to allow for direct location-specific comparisons between scans.

Cluster Methods
Measurements were grouped by participant age in decades (≤10 years, 11-20 years etc., up to 51-60 years; Table 1 ), and average CT, FSSC, and BSSC were calculated for each decade bracket at each of the 57-point locations. Cluster analyses were performed using SPSS software (version 25.0; IBM Corp., New York, New York, USA). Hierarchical clustering uses an agglomerative technique to group statistically similar measurements into successively larger clusters that were defined through within-groups linkage and squared Euclidean distance to compute distances between clusters. The separability of the initial clusters obtained using hierarchical clustering was calculated using the d’statistic :
d’=|x1−x2|0.5×(σ12+σ22)
Cohort | n | Age (y) ± SD | Gender, M:F | Eye Tested, OD:OS | SE ± SD, Diopters | Astigmatism ± SD, Diopters |
---|---|---|---|---|---|---|
All participants (y) | 117 | 32.90 ± 16.00 | 57:60 | 65:52 | −0.69 ± 1.69 | −0.66 ± 0.64 |
≤10 | 9 | 9.21 ± 0.67 | 5:4 | 4:5 | −2.15 ± 1.24 | −0.58 ± 0.71 |
11-20 | 25 | 16.32 ± 2.69 | 12:13 | 12:13 | −0.99 ± 1.52 | −0.53 ± 0.61 |
21-30 | 24 | 26.03 ± 3.32 | 16:8 | 14:10 | −1.60 ± 1.98 | −0.97 ± 0.75 |
31-40 | 19 | 34.54 ± 3.29 | 11:8 | 10:9 | −0.55 ± 0.82 | −0.67 ± 0.66 |
41-50 | 19 | 46.27 ± 2.45 | 7:12 | 11:8 | +0.02 ± 1.31 | −0.57 ± 0.56 |
51-60 | 21 | 57.05 ± 3.17 | 6:15 | 14:7 | +0.58 ± 1.51 | −0.58 ± 0.54 |
Age-corrected cohort | 98 | 32.58 ± 17.42 | 46:52 | 55:43 | −0.72 ± 1.81 | −0.66 ± 0.65 |
Clusters were systematically merged, starting with the cluster pair with the lowest d’ value, until all comparisons reached our threshold for statistical separability set at d’ >1 at a final number of clusters k .
Unlike hierarchical clustering, k -means clustering involves the assignment of points into a predetermined number of clusters that is chosen before the algorithm is performed. To enable direct comparisons with hierarchical cluster patterns, the desired number of clusters was set at k and separability of clusters was calculated in an identical fashion.
Regression Analysis
Average CT, FSSC, and BSSC were calculated per decade bracket and cluster and fitted with linear and second order polynomial (quadratic) regression curves, and sum-of-squares F tests were performed using Graphpad Prism software (version 7.04; Graphpad, La Jolla, California, USA) to compare goodness of fit of these models to the dataset. Sliding window analyses were also performed using decade windows, with measurements averaged over each window (ie, ≤10 years, 11-20 years…51-60 years, then ≤11 years, 12-21 years…52-60 years, and so on), to minimize the potential bias of using semiarbitrary decade groupings while maintaining the advantages of pooling similar measurements in reducing measurement noise. The results of the sliding window analyses were fitted with the most appropriate polynomial regression curves using Graphpad Prism. To determine whether regression models varied between clusters, 1-way analysis of variance with Tukey multiple comparison tests of the regression coefficients was performed for each cluster pattern and corneal parameter. The points of inflection for each polynomial regression model were calculated using Matlab (version R2017b; Mathworks, Natick, Massachusetts, USA) to identify time points where significant points of change in the investigated corneal parameters occurred.
Regression Model Validation
To verify the accuracy of the derived polynomial models, participants in the 31- to 40-year-old bracket were selected as a 35-year-old age-normative cohort ( Table 1 ), approximating the mean age of the entire cohort (mean 32.9 years; Table 1 ). The regression curves derived from the sliding window models and models derived from single averaged measurements per decade bracket (hereby referred to as decade-based models) were used to convert the 57-point measurements for CT, FSSC, and BSSC measurements from all other participants to 35-year-old equivalent data. Bland-Altman comparisons were then performed between the age-corrected and age-normative cohorts.
Statistical Analyses
Statistical analyses were performed using SPSS and GraphPad Prism. Normality of distribution of CT, FSSC, and BSSC at each location was determined using the D’Agostino and Pearson normality test. Correlations between point measurements of CT, FSSC and BSSC and ethnicity, spherical equivalent refractive error, and refractive astigmatism were analyzed using Pearson correlations, while correlations with gender were analyzed using unpaired Welch t tests, as previous studies have reported correlations between corneal parameters and these factors.
Results
Study Cohort
Of 117 participants included in this study, 51% were female ( Table 1 ). The most prevalent self-reported ethnicities were white (53%) and East Asian (23%); other less commonly reported ethnicities included Indian (5%), Middle Eastern (4%), Central or South American (3%), Pacific Islander (3%), Indigenous Australian (2%), and mixed descent (5%). In addition, only 8% of the entire cohort exhibited against-the-rule corneal astigmatism; although this sample was too small to investigate prevalence of against-the-rule astigmatism with increasing age, 56% of this sample were within the oldest age bracket (51-60 years), suggesting that the previously reported association of increasing against-the-rule astigmatism with age may also be present in the studied cohort. Meanwhile, 82% of the cohort with corneal astigmatism ≥0.75 D demonstrated with-the-rule corneal astigmatism ( Supplemental Table 1 ; Supplemental Material available at AJO.com ).
Cluster Analysis and Regression Models
Hierarchical clustering identified 7, 8, and 7 statistically separable clusters for CT, FSSC, and BSSC measurements, respectively. Each cluster represents groups of test locations demonstrating statistically similar age-related change, which are conveying using the colored cluster patterns in Figure 2 . Concentric patterns of age-related changes were observed for CT and FSSC measurements for both hierarchical and k -means cluster analyses; however, concentricity was not maintained in cluster patterns describing BSSC measurements.

Regression analyses were applied to average measurements pooled per decade bracket and per cluster according to the obtained cluster patterns to characterize age-related variation ( Figure 3 , A). Change per decade for clustered CT measurements was best fitted using linear regression, whereby the central clusters showed a gradual increase in CT and the peripheral clusters showed a gradual decrease with age; however, the goodness of fit of these models was superior for central clusters, as indicated by higher coefficients of determination ( R 2 = 0.002-0.62; Supplemental Tables 2 and 3 ; Supplemental Material available at AJO.com ). For FSSC and BSSC measurements, linear and quadratic regression models alternately provided superior fits for different clusters ( R 2 = 0.05-0.98 and R 2 = 0.006-0.85, respectively; Figure 3 , B and C ; Supplemental Tables 2 and 3 ; Supplemental Material available at AJO.com ).
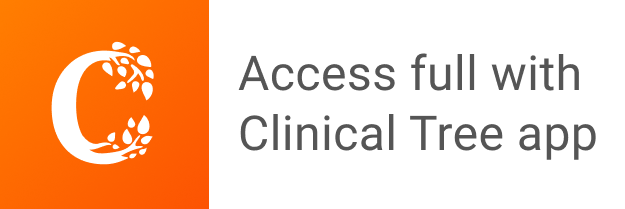