Purpose
Selecting reliable visual field (VF) test takers could improve the power of randomized clinical trials in glaucoma. We test this hypothesis via simulations using a large real world data set.
Design
Methodology analysis: assessment of how improving reliability affects sample size estimates.
Methods
A variability index (VI) estimating intertest variability was calculated for each subject using the residuals of the regression of the mean deviation over time for the first 6 tests in a series of at least 10 examinations for 2,804 patients. Using data from the rest of the series, we simulate VFs at regular intervals for 2 years. To simulate the neuroprotective effect (NE), we reduced the observed progression rate by 20%, 30%, or 50%. The main outcome measure was the sample size to detect a significant difference ( P < .05) at 80% power.
Results
In the first experiment, we simulated a trial including one eye per subject, either selecting randomly from the database or prioritizing patients with low VI. We could not reach 80% power for the low NE with the available patients, but the sample size was reduced by 38% and 49% for the 30% and 50% NE, respectively. In the second experiment, we simulated 2 eyes per subject, one of which was the control eye. The sample size (smaller overall) was reduced by 26% and 38% for the 30% and 50% NE by prioritizing patients with low VI.
Conclusions
Selecting patients with low intertest variability can significantly improve the power and reduce the sample size needed in a trial.
G laucoma is the second leading cause of world blindness, estimated to affect more than 100 million persons during the next decades. Therefore, even modest improvements in glaucoma treatments would prevent blindness in thousands of patients. , Presently the only proven treatment for ameliorating glaucoma progression is lowering of intraocular pressure (IOP). New treatments might entail better ways for lowering IOP (surgery/sustained delivery) or a neuroprotective agent. Although interest in neuroprotective approaches is increasing, any new treatment needs to be scrutinized by a clinical trial before it can become widely adopted. Primary outcomes for these trials need to be sensitive enough to detect glaucoma progression in the relatively short time span of the trial. Some investigators, often on the insistence of regulatory bodies, are adopting patient-reported outcome measures as a primary trial outcome. Yet patient-reported outcome measures have been shown to be insensitive to detecting the small changes in visual function that might occur over the short period of time of a clinical trial.
Currently, the best candidate for a primary outcome, one approved by the United States Food and Drug Administration for example, is measurement of the visual field (VF) using standard automated perimetry—an established technology that has been in clinics for more than 30 years. Yet, VF assessment is onerous for some individuals and the measurements themselves can be noisy. This could result in the specification of disease progression being challenging in these patients. As a result, the use of VF worsening as the primary outcome in neuroprotection trials has been considered to require large numbers of persons over several years’ time by some investigators. The fact that one large clinical trial of neuroprotection oral treatment was apparently unsuccessful seemingly reinforced this pessimistic viewpoint, though the use of VF testing as an outcome was not the primary reason for study failure.
A variety of solutions to improve the chances of detecting VF progression in a clinical trial have been suggested. If trials were to extract information from every single participant by measuring the rate of VF loss, the outcome can be more adequately assessed than if event-based outcomes (patients defined as progressing or not) are used. Modeling experiments (computer simulations) have been used to show that sample sizes required for trials can be substantially reduced by evaluating differences in the rates of VF loss between groups using linear mixed effects models. Another way to improve the power of glaucoma trials is to increase the frequency of VF testing during follow-up or schedule clusters of tests at the beginning and end of the trial period. , Such methods were used successfully in the UK Glaucoma Treatment Study and were proven effective in a trial duration of just 2 years.
Some have suggested that selective patient inclusion could produce a more rapid outcome, perhaps by recruiting only those patients who are more likely to progress: the elderly, those with exfoliation, or those with higher baseline IOP. However, evidence from clinical trials shows that highly selective recruitment criteria leads to excessively long pretrial period and the need for many recruiting sites. Others suggest recruiting patients who show rapid VF progression in the recent past. This has an ethical and practical flaw, in that once one knows the patient has recently worsened, IOP must be further lowered, making continued rapid progression less likely. Each of the selective recruitment strategies also is subject to the weakness that any result could fail to generalize to the overall open angle glaucoma population and require longer to recruit sufficient subjects, increasing the cost.
A more effective method for inclusion of the least number of persons to detect a neuroprotective effect may be to identify and recruit patients with a lower intertest VF variability (sometimes referred to as between-test variability). The potential for reduction in sample size and study duration using such an approach was previously suggested on a theoretical basis, showing that, for particular levels of intertest field variability and neuroprotective effects, satisfactory sample sizes and study durations could be achieved. Throughout the present report, we presume that all persons, both in the new treatment and control arm, will have appropriate and similar IOP-lowering therapy. In the present report, we test this approach using modeling experiments based on thousands of real VFs extracted from 5 different glaucoma clinics in England. We aim to confirm the potential improvement in power obtained by recruiting people by their past VF reliability. We also propose a practical strategy for trial recruitment from an electronic medical record (EMR).
Methods
Data Set
VF data were extracted from an EMR (Medisoft; Medisoft Ltd, Leeds, UK) from 5 regional National Health Service Hospital Trust glaucoma clinics in England in November 2015 as described elsewhere. , All patient data were anonymized at the point of data extraction and subsequently transferred to a single secure database held at City, University of London. Subsequent analyses of the data were approved by a research ethics committee of City, University of London. The study adhered to the Declaration of Helsinki and the General Data Protection Regulation of the European Union. All VFs were recorded on the Humphrey Visual Field Analyzer (Carl Zeiss Meditec, Dublin, California, USA) using a Goldmann size III stimulus with a 24-2 test pattern and the Swedish Interactive Testing Algorithms (SITA Standard or SITA Fast). The aggregated database contained 576,615 VFs from 71,361 people recorded between April 2000 and March 2015.
For this study, we selected all patients with at least 10 VFs recorded over at least 4 years in one or both eyes. We excluded any patient whose EMR contained ocular surgery other than cataract removal during this follow-up period. The use of IOP-lowering medications was not consistently reported. However, given that all these patients are being followed up in glaucoma clinics, their IOP would be managed according to standard clinical practice. Qualifying subjects had a mean deviation (MD) worse than –2 dB in at least 2 VFs. , It seems likely that subjects with this level of damage and frequency of VF testing were either strong glaucoma suspects or persons with glaucomatous optic neuropathy. Our selection yielded 5,149 eyes from 3,732 people (68,812 VF tests). We then excluded patients with at least 1 VF test felt to be unreliable because of false positive errors (FP ≥ 15%). No exclusion criteria were applied based on fixation losses or false negative errors. The final selection included 37,281 VF tests from 2,804 subjects. (Of these, 922 patients had sufficient numbers of fields that met the inclusion criteria for both eyes [1,844 eyes, 24,316 VF tests].)
Variability Index
To be widely applicable in clinical trial design, the variability index (VI) used for patient selection needs to be easily calculated from readily available clinical data. We used the variability of the MD of the first 6 VFs in the series. Simply put, for each subject we fitted a linear regression on the MD values over time (years) of the first 6 VF tests. The differences between the actual MD value at each point in time and the corresponding predicted value from regression were then calculated (residuals). The VI is simply the standard deviation of these residuals. A higher VI therefore indicated larger intertest variability in a patient’s VF follow-up. MD was preferred to the other global indices such as the Visual Field Index (VFI) for comparability of our results with the literature and its simplicity of calculation in the simulated VF (see below). Moreover, the values used to calculate the VFI can change from pattern deviation to total deviation according to the level of damage and this could introduce inconsistencies in the results of the simulations. Pattern standard deviation was also avoided because it does not have a monotonic relationship with the level of damage, reverting back toward normal values for more advanced glaucoma. Finally, previous reports have shown MD to be superior to both VFI and pattern standard deviation in detecting glaucoma progression.
Simulation of the VF Series in a Clinical Trial Protocol
In trials, as opposed to everyday clinical practice, VFs are typically measured at regular intervals with a precise sampling scheme. Our simulations followed the sampling scheme (patient visits) used in the UKGTS trial, namely, 16 fields over 2 years. Specifically, VF tests were performed at baseline and then at 2, 4, 7, 10, 13, 16, 18, 20, 22, and 24 months, with clustering of 2 fields (test-retest) at baseline and at 2, 16, 18, and 24 months.
The simulation must also account for the relationship between sensitivity (decibels) and variability at each VF location. A method to quantify this variability, proposed by Russell and associates, uses linear regression of sensitivity over time fitted at each individual VF location for each eye (pointwise linear regression). The residuals from each regression are used to quantify the variability for each sensitivity value predicted by the pointwise linear regression, which is known to be larger at lower sensitivities. However, simulating local noise alone is generally not sufficient accurately to reproduce the variability of the MD, which is mostly influenced by global fluctuations in the VF. , , Such fluctuations affect the VF as a whole rather than acting only on specific locations. They can be determined by a series of factors, but are usually well characterized as a random process. , , Wu and Medeiros introduced a method to capture such fluctuations, based on the use of “noise templates” mapped in a standardized probability space that is independent of the specific threshold values (see Supplementary Material). In our work, we wished to retain the relationship between the VI of each subject (from the standard deviation of the MD residuals of their first 6 tests) and their pointwise variability in the simulations. To this aim, we used the model proposed by Wu and associates with minor modifications. The full methodology is detailed in the supplementary material. Importantly, our simulations sampled (with replacement) noise templates derived only from the VF series of the subject being simulated. This ensured that the noise in the simulations better reproduced the behavior typical of that specific patient. Figure 1 shows our simulation paradigm, with 2 examples from 2 patients with different levels of noise.

Calculation of the Effect of Treatment
Next, to estimate the potential effect of therapy on VF worsening, we simulated multiple series of VFs for each subject using the simulation method described in the previous section. There were 4 possible series that were simulated for each patient: one series with no treatment effect (control) and 3 series with increasingly beneficial (hypothetical) neuroprotective effects on ameliorating speed (rate) of progression. These slowed the pointwise VF progression velocity by 0.10, 0.15, or 0.25 dB/y, respectively. These values represent therapeutic improvements of 20%, 30%, and 50% (small, medium, and large), respectively, based on the average progression rate among our included data set, which was –0.51 ± 1.04 dB/y in MD. This progression rate was calculated on VF tests after the sixth test in the series for each subject using 1 eye per subject, the same used for Experiment 1 (see later). To simulate a parallel 2-group clinical trial, subjects were randomly assigned to either the treatment arm (with one of the neuroprotective effect) or to a placebo arm (with no improvement). Following Wu and associates, we used a linear mixed effect model with random intercepts and slopes to make full use of the whole series of the 16 individual MD values calculated from the simulated VF tests. The MD for the simulated series was calculated using the visualFields package for R (R Foundation for Statistical Computing, Vienna, Austria). An interaction term in the model denoted the difference in progression slope between the 2 arms. The effect was detected when the P value for this interaction term was <.05. This procedure for the random assignment and the calculation of the P value was repeated 5,000 times for different sample sizes. The power at each sample size was then the percentage of realizations for which an effect of the selected magnitude was detected in 5,000 attempts. This overall method was used in 2 sets of experimental approaches.
Experiment 1: Using 1 Eye per Patient
We used the described methods to assess the possible effect of prior subject variability on the sample size needed to determine specific treatment outcomes in 2 ways. The first, presented here, was meant to simulate the effect of a systemic treatment, in which the 2 eyes cannot be treated separately. In this framework, only one eye per patient was simulated, and the comparison was performed between the average effect in the 2 independent arms of the trial. We ordered (ranked) all eyes according to their VI, as could be easily done in an EMR of a real clinic. Power curves were then calculated by recruiting a progressively increasing number ( n ) of subjects. Two recruiting approaches were compared: the first relied on random selection of n patients; the second selected the first n subjects in the database ordered by VI (ie, the n least variable subjects with minimum intertest variability). For each approach, the n subjects were then randomly split between the treatment and placebo arm, and the process was repeated 5,000 times for selected n and each selection methods. Ideally, this process should be repeated for every n from 1 to the half the size of the sample (1,402), so that the same n could be used for both arms of the trials. However, for the practical implementation of the procedure, the calculations were performed at defined n , from 25 to 1,402 every 50 subjects, with the last increment equal to 27 eyes.
Experiment 2: Two Eyes per Patient
In this experiment, we simulated a treatment that could be applied to only one eye of the patient, leaving the other untreated. In this case, the fellow eye could be used as an internal control. To apply the selection based on variability, we computed a VI for each subject as the average of the VIs of the 2 eyes. This subject VI was used for the selection process, which was identical to Experiment 1. The linear model used to compare progression rates had to be modified to account for this design. In particular, the random effect was applied only at the subject level, because the 2 eyes were included in the 2 different arms of the trial. The fixed effect part of the model was the same. However, to model the individual differences in progression rates between the 2 eyes, we included the interaction term as part of the random slope. The calculations of the power curves were identical to Experiment 1. For this experiment, the n values of the power curves were from 25 to 922 every 50 subjects, with the last increment equal to 47 subjects.
Results
Median number of VF tests for included patients was 13 (interquartile range [IQR]: 11, 15). Baseline values for patients were defined as those at the point of their sixth VF test, with a median (IQR) age of 68 years (60, 75) and MD of –6.14 dB (–11.05, –3.51). Rate of progression was –0.13 ± 0.81 dB/y (mean ± SD) during the first 6 VF tests and –0.51 ± 1.01 dB/y from subsequent examinations. Median (IQR) VI per eye was 1.09 dB (0.72, 1.67) when calculated on the first 6 VFs and 1.08 dB (0.74, 1.63) when calculated on the rest of the series. There was a significant correlation between the log 10 -transformed VIs calculated using the 2 parts of the series (correlation coefficient = 0.26, P < .001). Because the data may have been influenced by whether subjects had undergone cataract surgery during follow-up, we present the data stratified by cataract surgery in Table 1 . For Experiment 2, we could only use patients who had both eyes meeting the inclusion criteria. Descriptive statistics for this subset of eyes are also reported in Table 1 . This subset included 1,844 eyes from 922 subjects, with a median (IQR) number of VF tests of 12 (11, 15). Median (IQR) VI per subject (used in the simulations for Experiment 2) was 1.14 dB (0.82, 1.69) when calculated on the first 6 VFs, and 1.18 dB (0.84, 1.72) when calculated on the rest of the series. There was a significant correlation between the log 10 -transformed subject VIs calculated with the 2 parts of the series (correlation coefficient = 0.32, P < .001) and between the log 10 -transformed VIs of the 2 eyes from the same subject (correlation coefficient = 0.46 for the first 2 VFs, 0.41 for the rest of the series, P < .001).
Cataract surgery | |||
---|---|---|---|
One eye per subject (Experiment 1) | |||
None (n = 2,001) | Before the 6th VF test (n = 406) | After the 6th VF test (n = 329) | |
Baseline age, y | 67 [57, 74] | 71 [65, 76] | 73 [66, 78] |
Baseline MD, dB | –5.91 [–10.63, –3.39] | –7.22 [–12.85, –4.38] | –6.58 [–12.39, –3.52] |
Variability Index, dB | 1.07 [0.69, 1.63] | 1.34 [0.91, 1.89] | 1.00 [0.68, 1.66] |
Number of tests | 12 [11,15] | 14 [11,16] | 12 [11,14] |
Rate of progression, dB/y | |||
First 6 tests | –0.07 [–0.41, 0.25] | –0.18 [–0.56, 0.13] | –0.12 [–0.49, 0.19] |
After the 6th test | –0.33 [–0.79, –0.02] | –0.34 [–0.79, 0.02] | –0.46 [–1.04, –0.07] |
Two eyes per subject (Experiment 2) | |||
None (n = 1,276) | Before the 6th VF test (n = 309) | After the 6th VF test (n = 261) | |
Baseline age, y | 68 [58, 75] | 73 [66, 77] | 75 [68, 79] |
Baseline MD, dB | –5.95 [–10.79, –3.35] | –7.69 [–13.00, –4.51] | –6.58 [–12.45, –3.58] |
Variability Index, dB | 1.12 [0.71, 1.66] | 1.39 [0.93, 1.94] | 0.95 [0.65, 1.71] |
Number of tests | 12 [11,15] | 13 [11,16] | 12 [11,14] |
Rate of progression, dB/y | |||
First 6 tests | 0.01 [–0.35, 0.34] | –0.14 [–0.53, 0.21] | –0.10 [–0.44, 0.21] |
After the 6th test | –0.29 [–0.77, 0.01] | –0.40 [–0.82, 0] | –0.47 [–0.97, –0.08] |
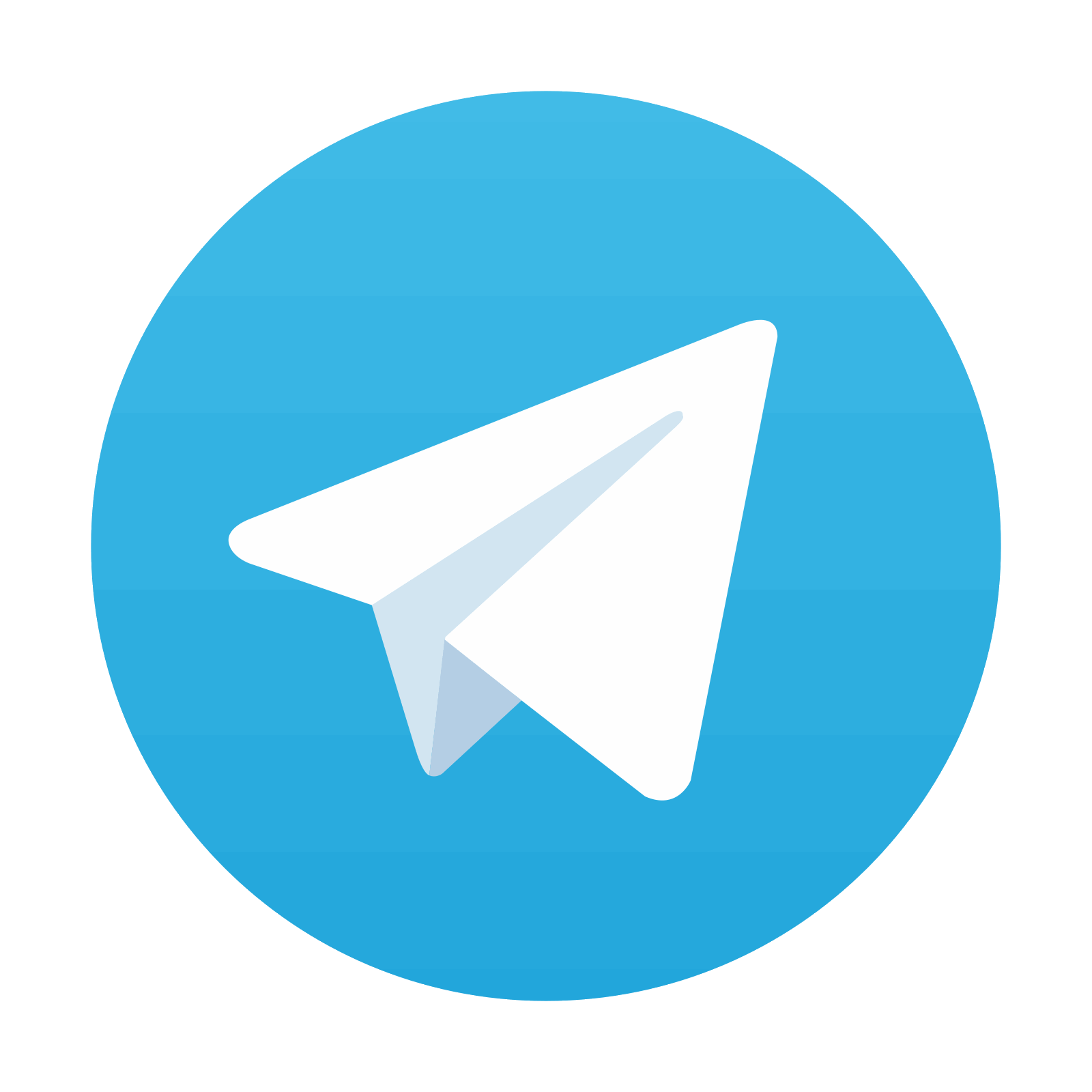
Stay updated, free articles. Join our Telegram channel

Full access? Get Clinical Tree
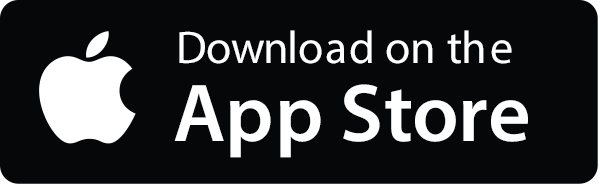

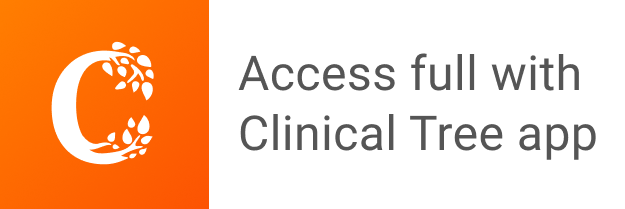