Purpose
To analyze the relationship between rates of false positive (FP) responses and standard automated perimetry results.
Design
Prospective multicenter cross-sectional study.
Methods
One hundred twenty-six patients with manifest or suspect glaucoma were tested with Swedish Interactive Thresholding Algorithm (SITA) Standard, SITA Fast, and SITA Faster at each of 2 visits. We calculated intervisit differences in mean deviation (MD), visual field index (VFI), and number of statistically significant test points as a function of FP rates and also as a function of general height (GH).
Results
Increasing FP values were associated with higher MD values for all 3 algorithms, but the effects were small, 0.3 dB to 0.6 dB, for an increase of 10 percentage points of FP rate, and for VFI even smaller (0.6%-1.4%). Only small parts of intervisit differences were explained by FP ( r 2 values 0.00-0.11). The effects of FP were larger in severe glaucoma, with MD increases of 1.1 dB to 2.0 dB per 10 percentage points of FP, and r 2 values ranging from 0.04 to 0.33. The numbers of significantly depressed total deviation points were affected only slightly, and pattern deviation probability maps were generally unaffected. GH was much more strongly related to perimetric outcomes than FP.
Conclusions
Across 3 different standard automated perimetry thresholding algorithms, FP rates showed only weak associations with visual field test results, except in severe glaucoma. Current recommendations regarding acceptable FP ranges may require revision. GH or other analyses may be better suited than FP rates for identifying unreliable results in patients who frequently press the response button without having perceived stimuli.
WITH the introduction of computerized perimeters in the 1970s, 3 so-called “reliability parameters” were implemented with the hope of helping users judge whether test results were reliable and useful. These parameters were fixation losses (FLs), false negative (FN) responses, and false positive (FP) responses. FL responses are obtained using a method described in 1974 in which test stimuli are presented at the expected location of the physiologic blind spot of the tested eye. The method was originally designed to give a qualitative idea about fixation in an early computerized perimeter, where the operator could not see the tested eye. The method has been widely used in many or most automated perimeters, but has well-known shortcomings, especially in eyes where the blind spot is not situated in the assumed location. Today, various methods for gaze tracking can be considered superior to the blind spot technique, and at least one new testing algorithm relies by default upon gaze tracking and not FL estimates based on the blind spot method.
FN responses were intended to be an index of patient vigilance. FN rates usually are measured by displaying stimuli that should be easily visible, based upon threshold sensitivity measurements made at the chosen locations earlier in the test. However, in the 1980s it was reported that the percentage of FN answers depended more on the level of visual field damage than on patient vigilance. In Bengtsson and Heijl, this shortcoming was clearly demonstrated by testing both eyes of patients having unilateral glaucoma. It is now recognized that test results should not be discarded solely on the basis of elevated FN response rates.
While FL and FN rates have been considered decreasingly important over time, this has not been the case thus far for FP response rates. FP rate estimates are meant to identify “trigger-happy” testing behavior, ie, examinations in which patients too frequently pressed the perimeter’s response button without having perceived a stimulus. Classic “trigger-happy” fields, with very high-threshold sensitivity values and white patches in the grayscale maps, often have high percentages of FP answers, but this is not always the case ( Figure 1 ). FP rates were originally estimated using catch trials in which no stimulus was presented, noting if the patient erroneously pressed the response button. More recently, Swedish Interactive Thresholding Algorithm (SITA) testing programs have incorporated a different method of estimating FP rates that is based upon detection of patient responses during times when it is impossible or unlikely that a stimulus was seen.


The reason that high FP response rates are of interest is that they are expected to be associated with artifactually elevated threshold sensitivity values, with higher FP rates being associated with higher mean deviation (MD) values, both in perimetry-naïve normal subjects and in patients with glaucoma. In the first of these studies, the analysis was based on just a single visual field test per normal subject, and in the latter study the results were based on differences between predicted and observed MD values in eyes with suspect or manifest glaucoma. However, FP rates have also been reported to have almost no correlation to measurement variability in a cohort of patients with suspect or manifest glaucoma who underwent threshold visual field testing twice within approximately 1 week.
Recommended limits for clinically “acceptable” FP rates have evolved over time. In the 1980s, we used an arbitrary limit of 33%, which simply was the limit we had chosen as an exclusion criterion for the visual field tests used to define normative significance limits for the first Humphrey Statpac interpretation package. Later, we suggested that FP rates >15% might indicate unreliable test results, a recommendation that was based upon the distribution of FP levels seen in a sample of field test results. Thus, FP rates >15% were flagged because they were uncommon, not because tests with higher FP rates were unreliable.
While in perimetry FP responses have traditionally been regarded as errors, signal detection theory provides a different perspective. In signal detection theory, FP responses are merely a reflection of the subject’s response criterion.
Recently, while developing the SITA Faster (SFR) test strategy, we noticed that the percentage of FP answers was higher with the new program than with SITA Fast (SF), and this has been subsequently reported by other investigators. It has been known for >20 years that FP rate estimates are typically slightly higher with SF than with SITA Standard (SS). Despite the higher FP rates with SFR, the results of a multicenter clinical trial showed almost identical SFR and SF threshold test results. We realized that further analysis of our multicenter SFR study data might provide an opportunity to study the relationship between FP answers and perimetric test results in greater detail. The distinctive advantage of using this recent study material was that all patients had been tested twice within such a short period of time, <2 weeks, that it was reasonable to postulate that no significant visual field progression would have occurred between the 2 tests. A second advantage was that we could simultaneously evaluate FP effects in all 3 SITA testing algorithms. Therefore, we hoped to determine the extent to which differences in FP measurements between the first and second tests were associated with observed differences in measured threshold sensitivity and associated metrics.
The aim of the current investigation was to analyze our recent multicenter data set, focusing on the relationship between FP rates and perimetric test results in each of 3 different testing strategies.
METHODS
SETTING
This prospective multicenter study was conducted at 5 centers located in 5 different countries in accordance with the Declaration of Helsinki and was approved by the Ethics Committee of the Gifu Prefecture Medical Association, the Ethics Committee of Tampere University Hospital, the Committee for Protection of Human Subjects of the University of California Berkeley, and the Hong Kong Hospital Authority Kowloon Central Research Ethics Committee. The study was also submitted to the Regional Ethics Review Board in Lund, Sweden. The Lund Board concluded that the study did not need their approval but that they saw no ethical issues.
STUDY POPULATION
The acquisition of study data has been previously described. The study included 126 patients with manifest or suspect glaucoma. No stages of glaucomatous visual field loss were excluded.
OBSERVATION PROCEDURE
All participants underwent Humphrey 24-2 visual field testing in a single study eye using 3 different threshold testing strategies (SFR, SF, and SS) in randomized order. All perimetric testing was repeated at a second visit, between 1 day and 2 weeks later, with testing order reversed. At each study site, participants underwent all testing on the same Humphrey 860 perimeter (Carl Zeiss Meditec, Dublin, California, USA).
If, during testing, the perimetrist observed patient gaze instability or results consistent with false responses, patient misunderstanding, or inattentiveness, the perimetrist was allowed to stop the test, reinstruct the patient, and restart the test from the beginning, thus discarding the interrupted test. However, once a test had been completed, it could not be deleted, and it was included in all statistical analyses.
MAIN OUTCOME MEASURES
From each visual field test we tabulated the percentage of FP responses, visual field index (VFI), and MD values, and the number of significantly depressed test points at the 1% and 0.5% significance levels in the total deviation (TD) and pattern deviation (PD) probability maps.
STATISTICAL ANALYSIS
First, we registered FP rates and MD and VFI values for the 3 algorithms. For each tested eye and each test strategy, we then calculated differences between visit 1 and visit 2 FP rates, as well as intertest differences in VFI, MD, and the number of significantly depressed test points. We then performed linear regression analyses with intrasubject FP differences as the explanatory variable and intrasubject differences in VFI, MD, and number of significantly depressed test points as the dependent variables. We also calculated intertest differences in general height (GH). , GH is the difference between the numerical TD values and the PD values in the Statpac program of the Humphrey perimeter. We then performed the same regression analyses with GH differences, instead of FP differences, as the explanatory variable.
We also performed regression analyses with FP differences as the explanatory variable and differences in MD, VFI, and in numbers of significantly depressed points with study eyes divided into 3 groups with early, moderate, or severe visual field loss using the MD values of the staging systems of Hoddap and associates and Mills and associates. The MD stage for each eye was defined as the average of the visit 1 and visit 2 MD values, for each test algorithm. Assumptions for linear regression were tested by residual analysis between differences in FP vs differences in MD and VFI. Histograms of residuals were produced, as were scatterplots of standardized residuals over standardized predicted values.
RESULTS
We analyzed test results from 125 patients, including 64 women (51%) and 61 men (49%). The mean age was 67 years (range 26-82 years). Results from 1 subject were excluded because testing of this patient had been interrupted because of the observation of large eye movements. The patient was reinstructed and a new test was started, but fixation stability was still considered unacceptable.
The 3 test strategies showed significantly different FP rates, while MD and VFI values were very similar ( Table 1 ). Intervisit differences shown in Table 1 were all distributed normally.
Visit 1 Mean; Median (Minimum, Maximum), Skewed Distribution | Visit 2 Mean; Median (Minimum, Maximum), Skewed Distribution | Intrapatient Difference (Visit 1 – Visit 2), Mean (SD), All Gaussian | |
---|---|---|---|
FP (%) SS | 2.8; 2 (0, 28) | 2.8; 2 (0, 13) | 0.0 (4.1) |
FP (%) SF | 3.3; 2 (0, 41) | 3.65; 2 (0, 32) | −0.4 (5.5) |
FP (%) SFR | 4.9; 0 (0, 39) | 5.0; 3 (0, 43) | −0.1 (9.5) |
MD (dB) SS | −8.5; −6.0 (−28.3, 0.56) | −8.5; −6,4 (−28.7, 0.58) | −0.1 (1.3) |
MD (dB) SF | −8.6; −6.2 (−28.7, 1.33) | −8.4; −6.1 (−28.9, 0.8) | −0.2 (1.6) |
MD (dB) SFR | −8.4; −5.8 (−28.5, 1.9) | −8.5; −6.4 (−28.2, 2.9) | 0.1 (1.5) |
VFI (%) SS | 75.9; 83 (8, 100) | 75.9; 83 (6, 100) | −0.0 (3.7) |
VFI (%) SF | 76.6; 82 (9, 100) | 77.1; 84 (11, 100) | −0.5 (4.6) |
VFI (%) SFR | 77.6; 85 (11, 100) | 77.1; 85 (11, 100) | 0.4 (4.6) |
For each of the 3 strategies, intervisit differences in FP explained only a small part of the intervisit differences in MD and VFI, despite reaching statistical significance in half of the analyses ( Table 2 ). Statistical significance may have been reached simply because of the relatively large number of observations. The coefficients of determination— r , the variability in the dependent variable that is explained by the explanatory variable—were small for all strategies for FP vs MD and even smaller for FP vs VFI. Higher FP rates were associated with greater increases in MD values, as expected, but the effects were small (0.4-0.5 dB), depending upon testing strategy, for an increase of 10 percentage points in FP rates (for example, an increase in FP rate from 5% to 15%). Effects for VFI were even smaller 0.6%-1.4% (approximately corresponding to 0.2-0.4 dB), for an increase of 10 percentage points in FP rates. Similarly, the associations between FP intervisit differences and differences in numbers of significantly depressed test points were weak for all 3 test strategies, with many r 2 values close to 0. Most of those relationships were not statistically significant.
r | Slope (Change per Percentage Point Increase in FP Rate) and 95% CI | Effect per 10 Percentage Point–Increase in FP | |
---|---|---|---|
Diff MD/diff FP SS | 0.01 | 0.04 (−0.02 to 0.08) | 0.36 dB |
Diff MD/diff FP SF | 0.04 | 0.06 (0.01-0.11) a | 0.60 dB |
Diff MD/diff FP SFR | 0.11 | 0.05 (0.03-0.08) a | 0.51 dB |
Diff VFI/diff FP SS | 0.00 | 0.07 (−0.10 to 0.22) | 0.56% |
Diff VFI/diff FP SF | 0.03 | 0.14 (−0.01 to 0.28) | 1.37% |
Diff VFI/diff FP SFR | 0.04 | 0.10 (0.01-0.18) a | 0.95% |
Diff TD 1%/diff FP SS | 0.00 | −0.04 (−0.25 to 0.10) | −0.4 points |
Diff TD 1%/diff FP SF | 0.03 | −0.15 (−0.30 to 0.01) | −1.5 points |
Diff TD 1%/diff FP SFR | 0.04 | −0.09 (−0.17 to −0.01) a | −0.9 points |
Diff PD 1%/diff FP SS | 0.00 | −0.02 (−0.17 to 0.13) | −0.2 points |
Diff PD 1%/diff FP SF | 0.00 | 0.00 (−0.11 to 0.10) | 0.0 points |
Diff PD 1%/diff FP SFR | 0.00 | −0.02 (−0.09 to 0.05) | −0.2 points |
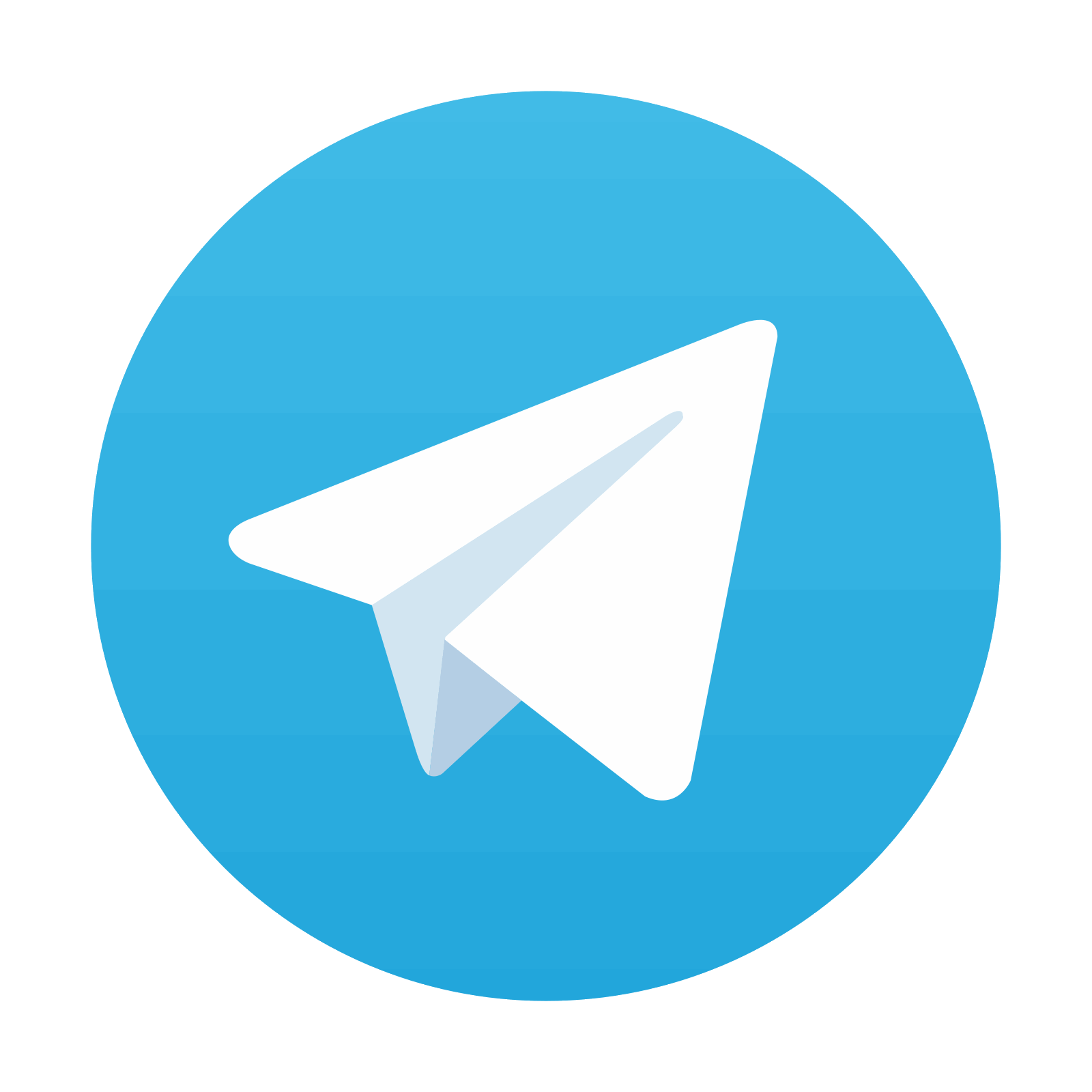
Stay updated, free articles. Join our Telegram channel

Full access? Get Clinical Tree
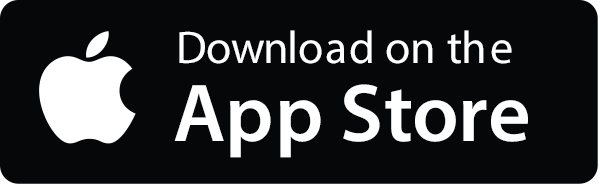

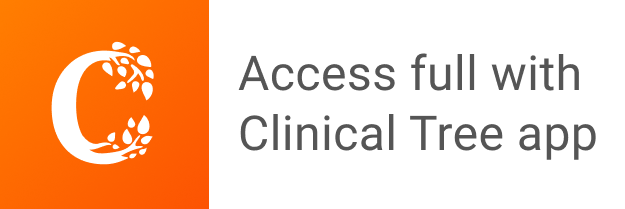