Introduction to the extrastriate cortex
The goal in this chapter is to develop a basic understanding of the function of extrastriate cortex. This is a collection of brain regions concerned with visual processing and that receive strong driving input from the primary visual cortex (V1). The function of these areas has historically been inferred from studies of patients with punctate lesions. In these patients, the visual deficits are highly specific, causing impairments in functions such as face recognition (prosopagnosia) or color perception (achromatopsia). These impairments are quite different from those caused by V1 lesions, which typically result in phenomenological blindness in affected portions of the contralateral visual field.
Extrastriate cortex regions are found proximally anterior to the primary visual cortex ( Fig. 31.1 ). They can be identified anatomically in some cases, or more frequently by the presence of a distinct retinotopic map. As noted in Chapter 30 , this is a point-by-point mapping of retinal space onto the cortical sheet, and it is important to appreciate that the cortex contains dozens of these maps. In extrastriate cortex, the purpose is to analyze specific aspects of the visual scene, and this localization of function explains the highly specific nature of the corresponding deficits following extrastriate lesions.

Although there are many different extrastriate areas, it was pointed out nearly 40 years ago that they can be categorized into two functional networks ( Fig. 31.2 ). This “two-stream” hypothesis was developed based on anatomical studies, as well as behavioral studies that showed a dissociation between perception and action. The behavioral studies yielded a particularly intriguing set of observations suggesting that people experience visual stimuli quite differently depending on whether they are physically interacting with them or simply recognizing them passively.

The first network is located ventrally, neighboring the hippocampus, and it is concerned with visual analyses that might be considered as the “passive” formation of visual memories. The second network is located dorsally, neighboring parietal and premotor cortex, and it is concerned with visual analyses necessary for actions such as navigating and grasping. Both are involved in visual perception, with the ventral network being most concerned with static images, and the dorsal network with moving ones. Each network comprises dozens of cortical regions arranged into a hierarchy, with each region processing visual signals and relaying them to the next. Along these “feedforward” pathways, regions proximal to V1 are often described as “early” stages in visual processing, and distal regions as “late” or “deep” stages. This picture is complicated somewhat by the existence of numerous “feedback” pathways that send signals in the opposite direction (from “late” to “early” stages), and whose function is poorly understood. There are also “lateral” connections within areas and across the two networks. In the following sections, we will briefly examine evidence from lesion studies that illustrate the differences between these networks, and then we will consider how each network performs particular visual functions, with an emphasis on the feedforward processing of signals along each pathway.
The ventral visual network
Here we describe the primary functions of a major portion of extrastriate cortex called the ventral network, which encompasses large regions of the occipital and temporal lobes. Ventral network dysfunction can result in agnosias related to form and color. This is well illustrated by the example of subject J, a 30-year-old woman who contacted research investigators at Bielefeld University in Germany circa 2018, presenting with a chief complaint of an inability to recognize faces, including those of her family, her husband, or even her own face in the mirror. J suffers from developmental prosopagnosia, a lifelong debilitating condition with serious social and socioeconomic consequences: “If you have a job where you sit in your office and people come to you at previously appointed times, it is easy. But if you have to actively approach people…you can’t do it if you don’t know who is who.” J suffered several job losses because of her condition. Although the first case was only formally recognized as recently as 1976, developmental prosopagnosia is now known to occur at an estimated prevalence of 2.5% across the population. Although the pathophysiology behind developmental prosopagnosia is poorly understood, cases of acquired prosopagnosia can be directly related to damage to inferior occipital and temporal cortex, a region that functional imaging has confirmed responds most strongly to pictures of faces.
The best-known cases of prosopagnosia show little to no recognition impairment of other object categories (e.g., cars, tools), but this is relatively rare; most cases of face recognition impairments are associated with general recognition deficits. This reflects the underlying architecture of the ventral network: it comprises a set of cortical areas, each with relative functional specializations, yet still highly interconnected. In the macaque monkey brain, ventral network areas include seven main regions: primary visual cortex (V1), V2, V3, V4, posterior inferotemporal cortex (PIT, sometimes labeled as TEO in the early anatomy literature, this latter term’s origins are uncertain but often interpreted as temporo-occipital), and central/anterior inferotemporal cortex (C/AIT, or temporal area E), with parts of the ventral temporal pole (PG). Human ventral network regions also include areas V1–V3, a V4/V8 complex, followed by the lateral occipital complex (LOC) and ventral occipitotemporal cortex (VOT). In the monkey, these ventral network areas have been defined using anatomical tracing, single-cell electrophysiology, targeted lesions, and imaging, whereas in humans most of these areas have only been identified using functional imaging. For this reason, this section will focus mostly on the monkey ventral network.
Areas of the ventral network are interconnected with other brain regions involved in memory (hippocampus and parahippocampal regions), action association (striatum), and flexible planning (prefrontal cortex); these different outputs suggest that the larger ventral network comprises multiple subspecialized pathways. However, the overall functions of these subnetworks are all constrained by three major principles: (1) they are bounded by topographical maps present at birth ; (2) neurons in anterior cortical regions (e.g., anterior inferotemporal cortex [IT], temporal area G or TG) show larger receptive field (RF) sizes than those in more posterior regions (e.g., V1–posterior IT [PIT]); and (3) neurons with larger RFs tend to respond to more complex images, such as faces or places, in ways that are reminiscent of perception. We review these principles next.
Topographical features of the ventral network
From birth, the ventral network is defined by topography. The ventral network comprises retinotopic maps like the one defining area V1—maps of neighboring neurons in cortex also respond to neighboring regions of the visual field. These retinotopic maps are arranged back-to-back along the anterior-posterior axis, aligned such that their corresponding foveal representations merge along confluences , where, for example, V1 neurons with foveal RFs are located close to V2 neurons with foveal RFs (both sets being along the lateral occipitotemporal lobes). As in V1, the rest of the ventral network devotes more neurons to processing information at the fovea, both because V2, V4, and IT inherit architectural constraints from V1 and likely because shape- and texture-based recognition tasks require high-acuity vision for fine discrimination. Subsequently, both at the higher spatial scale detectable by functional magnetic resonance imaging (fMRI) and at the level of single-electrode electrophysiology, the entire ventral network shows stronger responses when objects are presented at or near the fovea versus the periphery.
There are at least three major foveal confluences within the occipitotemporal lobes (see Fig. 31.4 , top right) : one confluence is shared by the retinotopic maps of areas V1, V2, and V3, another confluence by the maps of V4 and the PIT, and the last one is contained within the central and anterior IT.
Besides being organized around these three supra-areal confluence maps, ventral network neurons also share other features as a whole, including a bias for responding to objects presented in the contralateral visual field. Posterior ventral network neurons have RFs fully contained within the contralateral visual field, and although anterior network neurons can also respond to stimuli in the ipsilateral hemifield, extending their RFs past the vertical midline of the visual field, they remain heavily biased in responding largely to contralateral stimulation. In fact, this bias is present throughout all visually responsive regions of the brain, including associative regions like prefrontal cortex. One final common topographical feature across the visual recognition network is that neurons responding to the upper visual field are situated in more ventral cortex, whereas neurons responding to the lower field are located more dorsolaterally on the occipital and temporal cortex ( Fig. 31.3 ); this anatomical segregation may provide a substrate for functional differences among neurons, even within the same area —such as some neuronal RFs becoming more selective for face-like patterns and others becoming more selective for body-like patterns, or some RFs becoming more sensitive for some colors over others.

Receptive fields and visual selectivity
Primary visual cortex contains neurons that respond to stimulation in locations as eccentric as ±80 degrees along the horizontal axis, and 40 to 60 degrees along the vertical axis. Although this rather large visual field appears perceptually continuous, neurons in every visual area sample this space discretely—for any given neuron, this visual field region is defined as its RF. Neurons in anterior IT can have RFs that capture large regions of the visual field (with RF sizes between 10 and 40 degrees of visual field, with size defined as the square root of the RF area), compared with 5 degrees in PIT and V4, and 2 degrees in V2. Although it is unclear whether every cortical area captures the same retinotopic coverage as V1 does (this partially depends on how one defines a cortical area), it is clear that every retinotopic location is covered along different stages of the ventral network. ,
Neurons with large RFs are also activated by more complex visual images. This was well characterized by a series of experiments by Tanaka and others, in which they stimulated neurons along the ventral network using simple images such as bars, discs, and other simple geometric patterns, and with physical objects and images of animals, foodstuffs, and other multifeature stimuli. They showed a gradual, continuous increase in the amount of visual information necessary to maximally activate neurons, starting from the posterior (caudal) to anterior (rostral) direction of the ventral network. This suggests a generalization of the fundamental principle postulated by Hubel and Wiesel about V1 (see previous chapter), where primary visual cortex neurons derive their selectivity as an elaboration of simpler inputs from neurons in earlier visual regions—a selectivity to oriented contours derived through spatially aligned inputs from the lateral geniculate nucleus. This recombination of inputs from neurons encoding simpler motifs gives rise to RFs that respond to more elaborate visual stimuli, and this algorithm appears to occur repeatedly over the ventral network. The result is neurons that combine simple oriented contours to derive sensitivity to curvature and broader spatiotemporal frequencies (present in V1, V2, and V4 ), followed by neurons that combine curvature, colors, and textures (in V4 and PIT ), and finally followed by neurons that respond maximally to photographs of real-world objects such as faces, body parts, and places (in central and anterior IT). This clear, unidirectional increase in both RF size and in stimulus complexity has led many investigators to refer to the ventral network as the ventral stream or ventral pathway , viewing it as a feedforward hierarchy of regions culminating in cortical stages of neurons with functional properties that approximate abstract perceptual judgments. Neurons at the end of this pathway then serve as inputs for more flexible, multisensory neurons in associative regions that truly encode abstract concepts, such as those in the medial temporal lobe that respond not only to images of a given individual but also to images of the individual’s written name (as exemplified by neurons tuned to information as specific as those related to the actor Jennifer Aniston ).
When it comes to the ventral stream, the leading edge of knowledge stops at the question of what visual attributes are meant to be encoded by IT neurons. What are the real-world features that best trigger responses from IT neurons? We have described these neurons as being attuned for the presence of “faces,” “body parts,” and “places,” and related their common absence or dysfunction to category-specific visual impairments such as prosopagnosia. Yet much like the idea of describing a highly interconnected recurrent network as a “stream,” this is only a correct first approximation that eventually runs into trouble.
Just as disorders of face perception are frequently associated with other kinds of object recognition issues, studies of neurons at the individual level reveal that neurons do not respond exclusively to images defined by semantic, categorical labels. For example, neurons that are strongly activated by photographs of faces will also respond to photographs of objects that are round and containing curved and straight contours, such as clocks or cut oranges. Most commonly, neurons will respond to randomly selected natural images belonging to no semantic category. Whereas there are strong arguments that the organization of the human ventral stream can be understood semantically (at least partially, by mapping neuronal function to word-based atlases ), it is not clear how this organization arises in nonhuman primates and other taxonomic groups lacking language. It is possible that language has developed around the preexisting organization of visually selective neurons in the ventral stream; this makes elucidating the fundamental principles behind this organization one of the most exciting current lines of work in this field.
These lines of work spring from several overlapping questions. One issue, raised previously in the chapter, relates to whether the functional organization is based on semantic, conceptual objects of social and ecological significance (“faces,” “animate” ), or if it is based on lower-level visual attributes correlated to but not identical to objects. Another question is whether these visual attributes are linked by a simple yet-undiscovered parametric relationship, as simple as the orientation-dependent topography of V1, the curvature-dependent topography of V4, or the direction-dependent topography of MT neurons (see next section). It is possible that instead, IT neurons are organized around a set of learned attributes defined by low-level visual similarity, much like current deep-learning models (i.e., convolutional neural networks) abstract the visual world.
How distributed is coding?
One well-established way to think about functional organization in the ventral stream is to ask whether a given visual percept is encoded by a small group of neurons in a sea of many otherwise silent neurons, or whether it is encoded in the distributed, concurrent activity of a much larger population of neurons. We previously described the existence of individual neurons in the human medial temporal lobe that responded when a subject was shown images or written references to the actor Jennifer Aniston. This finding is reminiscent of a philosophical concept made famous by Jerzy Konorski—the gnostic or “grandmother” cell , a theoretical neuron whose activity would reflect a subject’s perception of a given concept, such as their grandmother. In this scenario, an independent observer would only need to monitor the activity of that single cell to predict a subject’s responses. In the opposite scenario—that of a fully distributed code—the observer would never be able to ascertain the subject’s perceptual responses without monitoring the full population of neurons in a given region. Neither (extreme) scenario finds many defenders in the field of visual neuroscience—both scenarios are rendered implausible by the problem of efficiency: if the visual brain operated with gnostic cells, there would not be enough neurons to represent the astronomical number of concepts and patterns forming our perception. Similarly, if the visual brain required all of its neurons to represent every concept, energy costs would likely set up a lethal disadvantage to the organism. However, there remains abundant disagreement about intermediate scenarios. The idea that a given neuron represents particular values within a population-wide code (e.g., an axis-based, parametric space such as orientation) is prevalent in many research programs ; this idea is reminiscent of classic distributed coding views. In contrast, there is the idea that a given neuron’s activity represents the presence of a learned visual pattern; this idea is reminiscent of the grandmother cell hypothesis, only substituting a very complex abstract concept (grandmother) with a local combination of visual attributes (a given oriented contour). Convolutional neural networks have shown how difficult it is to defend either intermediate scenario as mutually exclusive of the other. Like mild versions of theoretical grandmother cells, hidden units in a neural network learn specific combinations of visual attributes from their training image sets, yet when presented with random photographs, nearly all of these hidden units can “respond” (i.e., emit nonzero outputs), providing information useful for downstream units. So do CNN hidden units implement distributed coding or more localist operations? The answer is that as convolutional filters, hidden units are capable of responding to any input but still show stronger responses to particular patterns. This is a combination of distributed and localist operations (see Box 31.1 ), and one that appears to be a good first-order description of neurons in the ventral stream.
Computational models are becoming integral to more and more biological vision research programs. Specifically, deep-learning models such as convolutional neural networks (CNNs), autoencoders, and vision transformers can serve as testing grounds for new hypotheses of neuronal function in the brain. As image-computable models, they can be used (1) to identify unexpected patterns or biases in preselected stimulus sets, (2) to streamline code for real-time closed-loop experiments, or (3) to perform companion in silico simulations complementing more expensive and time-consuming neuronal experiments. Although computational models are common in many other branches of neuroscience, CNNs have been particularly natural additions to vision studies because in fact they are algorithmic relatives of the mammalian visual system itself. One of the first image-computable models of vision was Kunihiko Fukushima’s Neocognitron , a deep-layer network directly inspired by Hubel and Wiesel’s findings in primary visual cortex (one of many of Fukushima’s neuroscience-inspired models ). Like V1, the Neocognitron comprised (1) units that worked like simple cells, combining geometrically simpler inputs to derive filters with elaborated selectivity, and (2) units that worked like complex cells, pooling the outputs of simple cells to attain invariance to position changes. The simple cell-like units also relied on a nonlinearity operation that limited their responses to positive values, like neurons show in their all-or-none, action potential–based responses. These three biologically inspired operations—filtering, pooling, and rectification, with the later addition of normalization—have been staples of CNNs from their earliest incarnations, and these operations have been generally accepted as mechanisms of neuronal function in the visual system. In addition to this overlap in local mechanisms, CNNs also share major architectural motifs characteristic of the visual system, including a hierarchical arrangement of layers (“areas”), and receptive fields that increase both in size and in shape complexity. However, one major difference between the biological visual system and CNNs is how they learn. CNNs are trained via supervised learning, specifically with backpropagation, an algorithm that adjusts each connection weight in the network based on errors between the CNN output and the label of the training example, and which naturally requires access to every weight in the model. Cortical areas do not show the type of precise, neuron-to-neuron symmetric projections that would make this algorithm biologically plausible—cortical feedback connections are diffuse, often linking nonvisuotopically corresponding regions. Although biologically plausible alternatives for CNN training are being explored, it does not seem as if any of them work as well as backpropagation. This raises two possibilities: either current theories lack the actual unsupervised learning mechanisms used by the brain, or current theories do have them (e.g., Hebbian plasticity–like algorithms, using rate of change of firing responses, feedback alignment ), which would mean that CNNs lack an important architectural feature that makes those learning mechanisms effective. This is one of many exciting questions at the intersection of neuroscience and machine learning.
How does the ventral stream acquire its anatomical organization?
A third important question about the ventral network’s functional organization is whether it is present at birth, formed by yet-undiscovered mechanisms engrained genetically by natural selection, or whether it develops through experience, based on the individual animal’s exposure to the statistics and content of the visual world. This is an active debate in studies of the ventral stream. Definitive data are lacking to settle this question, but there are tantalizing discoveries on either side of this innateness (or nativist ) versus bottom-up ( or experience ) debate. Evidence for the nativist camp include the following observations: (1) V1 neurons appear to be arranged based on their preferences for orientation even before eye-opening (so it follows that selectivity-map-shaping mechanisms could also exist in deeper stages of the ventral stream); (2) a functional imaging study has shown that some temporal cortical regions will respond preferentially to photographs of faces in sighted subjects, and these same cortical regions will respond preferentially to touching of faces in subjects with full loss of sight ; and, finally, (3) clusters of neurons that share preferential responses to photographs of faces, body parts, and places—these clusters are frequently described as “patches” or “domains” , —tend to occur in relatively similar locations across all primates, something that would be unlikely if experience was the only way to seed the locations of these patches. One explanation for the systematic location of face and place patches ties back to the (less controversially innate) retinotopic maps that define the ventral stream. Face patches appear near the foveal confluences of the temporal lobe, whereas place patches appear along more peripheral representations of visual space ; this correlation is illuminated by the fact that in baby monkeys, the foveal bias precedes the development of face patches. One explanation for this relationship is that neurons that respond preferentially near the fovea also tend to have smaller RFs, better suited for processing high curvature, whereas neurons that respond preferentially at the periphery of vision tend to have larger RFs, better suited for extended contours. Thus neurons may not be innately tuned for faces, but rather will be more likely to develop face tuning because they have smaller RFs situated near the fovea. A second observation emphasizing the importance of experience is that animals that grow without early experience to faces will not develop face patches and instead develop other kinds of patches, such as some focusing on hands. Equally relevant is that given intensive experience, humans and monkeys can develop cortical patches for letters and numbers, a finding that cannot be explained by innate or genetic mechanisms.
Although these categorical patches are useful measures in the nativism versus experience discussion, they also raise the question of their functional purpose. Why does the ventral stream develop patches at all? It is a general principle of cortical organization that neurons with similar visual tuning properties tend to cluster into groups as small as tens of micrometers and up to several millimeters. Clusters could be the result of neurons receiving similar inputs, grouping owing to activity-dependent interactions. However, some theories suggest that clusters serve as functional subsystems, where neurons with similar but nonidentical response properties can work together to overcome noise and variability in the visual input, variability brought about, for example, by incidental changes in viewing distance or angle.
Invariance
Solving the problem of selectivity in the ventral stream is a primary concern in the field; another is the question of invariance. A given object in the real world can project to the retina in a practically infinite number of ways, depending on its position relative to the viewer, current light conditions, and partial occlusion. These nuisance variables can be overcome by perception—we can recognize and keep track of the same object as it moves through a scene, passing through shadows, for example. This raises the question of how the ventral stream achieves this so-called invariance at the level of neurons. Most tests of invariance have involved a given set of images that are then presented at various positions, sizes, or even viewpoints. A given neuron responds differently to each image, allowing for these images to be sorted by the neuron’s “preference.” The most common way to define invariance is by measuring if the neuron demonstrates the same preference ranking across different nuisance transformations. This has revealed that neurons of the ventral stream show limited invariance to position, size, texture, and rotation. However, no neuron shows as much robustness to nuisance changes as the full perceptual system does, suggesting that this is a distributed property across subnetworks of the ventral stream. Invariance to face rotation, for example, is achieved by a subnetwork of face patches spanning all of IT cortex.
Applications
Beyond basic knowledge, what is to be gained by defining the mechanisms and representational content of the ventral stream? The second decade of the 21st century has seen an acceleration in the performance of neural networks and other machine intelligence (MI) models, many of which are trained to perform object classification, object detection and segmentation, and other visual recognition tasks. These computational models include a variety of architectures (such as convolutional neural networks, variational autoencoders, and vision transformers), and they have enormous potential to transform many industries, such as health care (assisting physicians in screening radiology and pathology imaging data), transportation (assisting drivers in monitoring hazardous situations), and security. If they worked as intended, an immediate concern would be their colossal potential for misuse, for example, by minimizing privacy. However, most current CNNs pose little threat because they do not work that well. Current models are not as robust as the visual system when presented with noisy or distorted images, and they can fail in unexpected ways when presented with images having different low-level statistical properties than those involved in their training image sets (for example, evaluating cartoon-like images if the networks were trained only with photographs). The visual system does not exhibit these issues. Further, these models can be vulnerable to so-called adversarial attacks , where photographs can be altered with nearly imperceptible noise patterns in order to change the classification offered by the models —a potentially disastrous vulnerability that could be exploited by bad actors in the context of self-driving cars, for example, causing models to ignore stop signs or misclassify pedestrians. Finding solutions to these problems is complicated by the fact that these multistage models have many degrees of freedom, learning features from data on their own—optimizing their internal weights according to relatively simple cost functions imposed at their output layer. Computer scientists and engineers are working to improve these systems using many approaches, and many believe that more improvements will arise from a better understanding of the brain’s ventral stream.
Fortunately, it is becoming easier and more commonplace to perform investigations of the ventral stream in the context of theories of MI. An increasing number of visual neuroscience laboratories are regularly using CNNs as part of their investigations of the brain, largely because both ventral stream and CNNs appear to converge to similar solutions. Investigators at the Massachusetts Institute of Technology showed that the more accurate a CNN is at classification, the more useful it is at fitting IT neuronal responses to photographs, presumably because accurate CNNs learn to represent similar visual features about photographs as the ventral stream does about the natural world. But this has not been easy to show, because as long sequences of functions, CNNs filter, recombine, and threshold pixel information, making it difficult to keep track of which visual features (which combinations of colors, shapes, textures) are ultimately emphasized or eliminated on their way to the output classification layer. This opaqueness has motivated engineers to develop methods for so-called explainable artificial intelligence (XAI), meant to provide rationales for the solutions found by deep networks. One popular XAI algorithm relevant to the ventral stream is DeepDream, which generates images that maximally activate units within a given CNN. This algorithm allows a reversal of the functionality of CNNs, where instead of propagating an image into the network to consequently measure a hidden unit’s response, one can perturb images at the pixel, input level using a function that optimizes the activation of that same hidden unit. This results in artificially reconstructed images containing the unique visual attributes that for a CNN make a dog a “dog,” for example, at least based on training data. In many ways, the goal of explaining artificial intelligence (AI) mirrors the goal of understanding neurons deep in the ventral stream, such as those in IT, and subsequently these XAI algorithms have been modified to be helpful in visual neuroscience studies. For example, one can fit pretrained CNNs to replicate the responses of given ventral stream neurons and then apply the DeepDream algorithm to the proxy CNN model, to visualize the visual features putatively learned by the neuron ( Fig. 31.4 ). Alternatively and more directly, one can bypass the CNN model and functionally link image-generating, deep-learning models with ventral stream neurons, even as deep in the hierarchy as IT. When applied to neurons in nonhuman primate IT cortex, this latter approach results in highly activating images that often resemble visual attributes commonly present in animals such as monkeys or dogs. However, more often, these neuron-guided synthetic images contain visual attributes that are not constrained to any given semantic category, and thus provide exciting new clues in the exploration about the types of visual information are encoded by IT neurons.
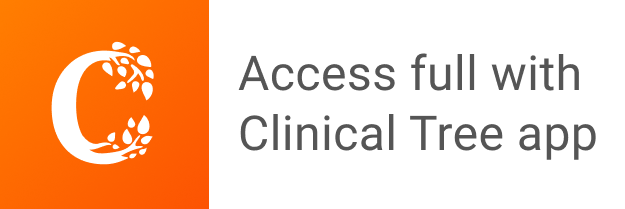