Abstract
Purpose
Oropharyngeal cancer (OPC) is the eighth most common cancer worldwide, however the genes involved in the development of OPC have been reported few. We constructed a co-expression network to extend knowledge of the molecular biomarkers in OPC development.
Materials and methods
Microarray data of HPV-active, -inactive, -negative OPC and normal benign tissue (uvula, tonsil) (Series GSE55550) were retrieved from NCBI GEO DataSets. We performed co-expression analysis of OPC transcriptome data by the Pearson correlation coefficient (PCC) method with the mutual rank (MR)-based cut-off using 13 guide genes.
Results
The OPC subnetwork contained three clusters: cell cycle (62 node genes and 125 edge genes), immune system (44 node genes and 70 edge genes) and organ morphogenesis (128 node gene and 215 edge genes) process separately.
Conclusion
Our co-expression analysis includes separated transcriptomes of OPC, which is a useful resource for OPC researchers to elucidate important and complex biological events, to prevent and to predict cancer.
1
Introduction
Oropharyngeal cancer (OPC) is the eighth most common cancer worldwide, whose incidence rates vary in men from 1 to 10 cases per 100,000 population in many countries. However, up to 60% of OPCs are associated with human papillomavirus (HPV), predominantly HPV16. Viral oncoproteins are most likely capable of transforming primary human keratinocytes from oral epithelia in vivo, which leads to a genetic progression to OPC by disrupting cell-cycle regulatory pathways . A promising strategy for the treatment of OPC and other cancers is molecular targeted therapy, which requires the understanding of specific molecular events of carcinogenesis and the different pathological pathways. It is also necessary to extend knowledge of biomarkers for prediction of OPC to allow a better classification and characterization of the tumor, which might help to specify individual multimodal therapy with increased efficiency and improve the appraisal of clinical outcome .
To study candidate biomarkers in carcinoma, one effective method is systematically analyzing the interactions and functions of genes with similar expression pattern during OPC using co-expression network. Recently, large-scale transcriptome data about OPC have become available from NCBI, which are valuable for comprehensive understanding of biological events and biomarker genes mining. Eight hallmarks of cancer have been reported during multistep development of human tumors, including sustaining proliferative signaling, evading growth suppressors, resisting cell death, enabling replicative immortality, inducing angiogenesis, activating invasion and metastasis, reprogramming of energy metabolism and evading immune destruction . Schmalbach et al. reported that the expression of complement component 1 (C1QB) was upregulated significantly in metastatic tumors with respect to both nonmetastatic tumors and normal mucosa samples . Cancer angiogenesis plays a key role in the metastasis of carcinoma, during which matrix metalloproteinases (MMPs) are essential for extracellular matrix degradation . MMP9 produced by tumor cells drives malignant progression and metastasis of basal-like triple negative breast cancer . MMP2 is involved in renal carcinoma , breast cancer and ovarian carcinoma . It was also reported that the invasion of human prostate cancer cell could be inhibited by finasteride though MMP2 and MMP9 downregulation . MMP3, also known as Stromelysin-1, promotes mammary carcinogenesis . Lee et al. demonstrated that MMPs could extracellularly regulate vascular endothelial growth factor (VEGF) bioavailability through intramolecular processing . It is believed that PI3K-Akt/PKB 3 pathway plays a critical role in cell survival, whose activation is linked to tumorigenesis . Up-regulation of PI3K has been reported in various human malignancies . Deregulated expression of c-myc oncogene appeared to be contributory to the development of numerous classes of tumors . BIRC5, also called Survivin, is preferentially expressed in human cancer cells and mediates cancer cell survival and tumor maintenance by influencing cell division and proliferation as well as inhibiting apoptosis . Callender et al. reported that cyclin D1 (CCND1) oncogene amplification was frequently found in primary head and neck squamous cell carcinoma . Upregulated expression of cyclooxygenase (Cox)-2 (also known as PTGS2), a key-inducible enzyme involved in the production of prostaglandins and other eicosanoids, may play a significant role in carcinogenesis . Recent studies have suggested that RhoA, RhoB and RhoC might have different roles in cancer cell invasion and migration . S100 proteins, a family of intracellular calcium-binding proteins, occur in various tumors . It was reported by Shang et al. that S100A10 might be a possible biomarker in colorectal cancer . Proliferating cell nuclear antigen (PCNA), a cofactor of DNA polymerases, determines tumor progression through its modifications and interaction partners . All of these genes might play important roles in tumor progression.
In this study, we retrieved the HPV-active, -inactive, -negative OPC and normal benign tissue (uvula, tonsil) microarray data from NCBI GEO datasets and performed co-expression network analysis based on 13 guide genes (C1QB, MMP9, PIK3CG, PCNA, BIRC5, MMP2, MMP3, MYC, CCND1, PTGS2, VEGFA, RHOC and S100A10) involved in OPC or other cancer development. Our analysis took 2 steps out from each guide gene and extracted a network containing three subnetworks about cell cycle (62 node genes and 125 edge genes), immune system (44 node genes and 70 edge genes) and organ morphogenesis (128 node gene and 215 edge genes) process separately. All of the genes obtained from co-expression network of this study could be resources of candidate biomarker genes for further research about cancer prevention and control.
2
Materials and methods
2.1
Microarray data
We retrieved the microarray data of oral and oropharyngeal cancers from NCBI GEO DataSets, which contain gene profiles of HPV-active, -inactive, -negative OPC and normal tissue (uvula, tonsil), a total of 155 samples (Series GSE55550).
2.2
Gene ontology (GO) and expression coherence (EC) analyses
GO terms were obtained for oral and oropharyngeal cancer genes to classify their biological processes (BP) and molecular functions (MF). GO terms for GO enrichment analysis and EC analysis were retrieved from DAVID ( http://david.abcc.ncifcrf.gov/ ). For GO enrichment analysis, the statistical significance of GO enrichment within co-expressed genes was evaluated against a background set consisting of 15,438 genes with at least one connection from the dataset of human co-expression networks using the hypergeometric distribution without a multiple-testing correlation, and P values of BP < 0.01 and P values of MF < 0.1 were set as the significant threshold. EC analysis was performed as previously described: genes with the same GO term (contained 20 to 100 genes) were used as a set of predefined functional genes . To calculate the EC of gene pairs per GO category, the PCC was used to measure expression similarity, whose threshold of 0.573 corresponded to the 99% of random PCC distribution derived for 1000 random genes (approximately 1000*999*0.5 gene pairs) . To calculate the random EC for GO categories, random gene sets were sampled with the same size as the category under investigation.
2.3
Co-expression analysis
To construct co-expression networks, we calculated the PCC values for all combinations of unique 28492 probes present on the GSE55550 series matrix. We estimated two PCC thresholds of 0.573 and 0.757, corresponding to the 99th percentile and 99.9th percentile of random PCC distribution derived for 1000 random genes (approximately 1000*999*0.5 gene pairs). Given that a PCC value of 0.757 was too high for co-expressed gene, we set a PCC threshold of 0.573 corresponding to the 99th percentile of random PCC distribution as described above . We also calculated MR values between gene pairs as another value of co-expression measure to further reduce the number of false positives, according to a previous report . Only the gene pair whose absolute value of MR was lower than 10 was considered as a significant connection for the co-expression network. The calculations were performed by the R/Bioconductor. To extract oral and oropharyngeal cancers subnetwork datasets, we took 2 steps out from a guide gene to extract the network vicinity, as described previously by Mutwil et al. . The network was illustrated using the program Cytoscape.
2
Materials and methods
2.1
Microarray data
We retrieved the microarray data of oral and oropharyngeal cancers from NCBI GEO DataSets, which contain gene profiles of HPV-active, -inactive, -negative OPC and normal tissue (uvula, tonsil), a total of 155 samples (Series GSE55550).
2.2
Gene ontology (GO) and expression coherence (EC) analyses
GO terms were obtained for oral and oropharyngeal cancer genes to classify their biological processes (BP) and molecular functions (MF). GO terms for GO enrichment analysis and EC analysis were retrieved from DAVID ( http://david.abcc.ncifcrf.gov/ ). For GO enrichment analysis, the statistical significance of GO enrichment within co-expressed genes was evaluated against a background set consisting of 15,438 genes with at least one connection from the dataset of human co-expression networks using the hypergeometric distribution without a multiple-testing correlation, and P values of BP < 0.01 and P values of MF < 0.1 were set as the significant threshold. EC analysis was performed as previously described: genes with the same GO term (contained 20 to 100 genes) were used as a set of predefined functional genes . To calculate the EC of gene pairs per GO category, the PCC was used to measure expression similarity, whose threshold of 0.573 corresponded to the 99% of random PCC distribution derived for 1000 random genes (approximately 1000*999*0.5 gene pairs) . To calculate the random EC for GO categories, random gene sets were sampled with the same size as the category under investigation.
2.3
Co-expression analysis
To construct co-expression networks, we calculated the PCC values for all combinations of unique 28492 probes present on the GSE55550 series matrix. We estimated two PCC thresholds of 0.573 and 0.757, corresponding to the 99th percentile and 99.9th percentile of random PCC distribution derived for 1000 random genes (approximately 1000*999*0.5 gene pairs). Given that a PCC value of 0.757 was too high for co-expressed gene, we set a PCC threshold of 0.573 corresponding to the 99th percentile of random PCC distribution as described above . We also calculated MR values between gene pairs as another value of co-expression measure to further reduce the number of false positives, according to a previous report . Only the gene pair whose absolute value of MR was lower than 10 was considered as a significant connection for the co-expression network. The calculations were performed by the R/Bioconductor. To extract oral and oropharyngeal cancers subnetwork datasets, we took 2 steps out from a guide gene to extract the network vicinity, as described previously by Mutwil et al. . The network was illustrated using the program Cytoscape.
3
Results
3.1
Biological significance of expression similarity
We first conducted an EC analysis using GO terms to examine the biological significance of expression similarity in the 155 microarray datasets of GSE55550 series in this study. EC analysis is a statistical analysis to detect whether the transcription profiles of genes belonging to a predefined functional gene set are correlated with each other . The EC score could measure the expression similarity within a predefined functional set of genes ; consequently, a higher EC score is obtained when most of genes with the same GO term are co-expressed with each other. In our EC analysis, the EC scores of all three GO groups (biological process, BP; molecular function, MF; and cellular component, CC) were higher than those of random sampling ( Fig. 1 ). Among the three GO groups, CC showed the highest expression similarity, in which approximately 16.4% of categories exhibited higher EC scores than random sampling at threshold EC score of 0.036, while approximately 10.7% and 9.5% did so in BP and MF, respectively ( Fig. 1 ).
3.2
Construction of the oropharyngeal cancer network
By using the Pearson correlation coefficient (PCC) method with PCC thresholds of 0.573, corresponding to the 99th percentile of random PCC distribution derived from 1000 random genes, we identified the final dataset of human OPC co-expression networks generated from all the 155 microarray data used in this study, which contains 28,130 genes (nodes) and 1,843,103 gene pairs (edges). From this entire dataset of the human OPC co-expression network, we are able to construct a subnetwork around gene(s) of interest input as guide genes and using the MR-based cut-off to obtain the genes with very close expression profiles (see Materials and methods).
To examine whether our co-expression network analysis can identify useful transcriptional networks related to human OPC development, we constructed a co-expression subnetwork centered on 13 genes (guide genes; green circles in Fig. 2 ), related with immune system (C1QB, MMP9, PIK3CG), cell cycle (PCNA, BIRC5), and organ morphogenes (MMP2, MMP3, MYC, CCND1, PTGS2, VEGFA, RHOC, S100A10) . The OPC network consisted of a large main cluster with the 13 guide genes ( Fig. 2 ), which in total contained 943 gene and 1583 gene interactions. The cluster of immune system contained several main genes such as C2 and C1QA, which are complement components, UBD (Ubiquitin D), CTLA4 (Cytotoxic T-lymphocyte-associated protein 4) and TNFRSF9 (Tumor necrosis factor receptor superfamily, member 9) ( Table 1 ). All of those genes are very important for human immune system, including both humoral immunity and cell-mediated immunity. The cluster of cell cycle process contained CENPF (centromere protein F) and CENPO (Centromere protein O), MCM2 (Minichromosome maintenance complex component 2), TRIP13 (Thyroid hormone receptor interactor 13) and KIF18A (Kinesin family member 18A) ( Table 1 ). All of those genes might participate in the cell cycle process for OPC development. The organ morphogenesis cluster of the OPC subnetwork contained genes involved cell-matrix adhesion (CTGF, Connective tissue growth factor), positive regulation of angiogenesis (ANGPTL4, Angiopoietin-like 4), tissue remodeling (VDR, Vitamin D receptor) and smooth muscle tissue development (PDGFRB and TSHZ3) ( Table 1 ). Those genes are very important for tissue remodeling, such as angiogenesis and epidermis development.
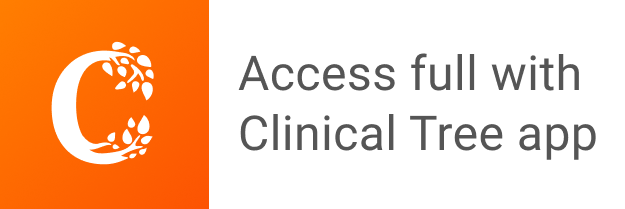