Clinical Epidemiology
Hussein Hollands MD, FRCSC, MSc (Epid)
Simon Hollands MD, MSc (Epid)
Sanjay Sharma MD, MS (Epid), FRCSC, MBA
Introduction
It is now commonplace for physicians and patients to expect that clinical decisions made by physicians—especially when related to therapy or prevention of disease—are based on sound scientific evidence. The efficacy and effectiveness of new treatments, whether aimed at reducing symptoms, curing disease, or reducing the risk of disease or disease symptoms, must be established with results from a randomized controlled trial (RCT) to be considered for government approval. In addition, patients are becoming more likely to demand scientific reasoning in the form of valid clinical studies before undertaking therapy.
Clinical epidemiology can be thought of as the science of making predictions regarding individual patients using a sound scientific method.1 To accurately assess the evidence available for a particular therapy or preventative measure, it is necessary to understand the fundamentals of clinical study design. Depending on the strengths and inherent biases of different study designs, epidemiological evidence varies greatly in value when applied to clinical decision making. Evidence is classified from Class I through V, from the strongest to the weakest, respectively.1,2 Table 1.1 summarizes the various classes of evidence as described previously.2
In this chapter, we present a review of the common study designs, focusing specifically on the important issues in critical appraisal, interpretation of clinical research, and application to patient care. For each study design, basic measures of effect size and statistical tests will be cited. A more detailed review of biostatistics is provided in Chapter 2.
Observational Study Designs
Results from observational study designs are often frowned upon as evidence to support decision making in medicine, but in many situations experimental evidence is not available and an observational study provides important information to support clinical decisions. For instance, observational studies are usually the only possible study design to investigate environmental or dietary risk factors for disease. They are also useful after a treatment becomes commonplace in medicine and it becomes unethical to randomize a patient to not receive that standard treatment. Finally, although clinical trials are ideal, they are expensive and time consuming and are simply not performed in large numbers. In a typical year, 87% of clinical articles published in the Archives of Ophthalmology, Ophthalmology, British Journal of Ophthalmology, and Canadian Journal of Ophthalmology are observational study designs.3 Consequently, clinical decisions in ophthalmology are being made using evidence from both clinical trials and observational studies.
Case Reports and Case Series
A case report outlines an interesting or new treatment approach and follows up a patient outcome into the future, whereas a case series simply lists a series of patients treated similarly and followed up during treatment in time. Neither of these study designs employs control groups and is thereby considered the weakest form of clinical evidence (Class V and IV, respectively). In general, clinical decisions should not be made using data only from case reports or case series, but these study designs
do play an important role in evidence-based medicine by enabling the medical community to stay current with respect to new treatment options and by fueling ideas for more definitive future studies.
do play an important role in evidence-based medicine by enabling the medical community to stay current with respect to new treatment options and by fueling ideas for more definitive future studies.
TABLE 1.1 Summary of Hierarchical Levels of Evidence for Interventional and Observational Studies2 | ||||||||||||
---|---|---|---|---|---|---|---|---|---|---|---|---|
|
Analytic Cross-Sectional Studies
An analytic cross-sectional study is used to investigate a risk factor for disease as opposed to a treatment or intervention. In this design, a cross-section of people is investigated, simultaneously in time, as to their exposure status and their outcome or disease status. In the analysis, the prevalence of a given risk factor or exposure to it is compared between those who happen to have the outcome of interest and those who do not. Prevalence is defined as the fraction of people who have the condition at a certain point of time. It is important to distinguish prevalence from incidence; incidence is the fraction of people who develop the condition over a certain period of time.
An example of a cross-sectional study investigated whether an association exists between the use of angiotensin-converting enzyme inhibitors (ACEIs) and the prevalence of age-related macular degeneration (AMD).4 Researchers reported that among a cross-section of 3,654 Australians, 1.3% of ACEI users had late AMD while 2.0% of people not using ACEI had late AMD (p < 0.05). A group of patients were observed at one point in time and each patient was defined on the basis of his or her exposure status (i.e., current use of ACEI) and on the basis of their outcome status (i.e., photographic evidence of AMD). Results of a cross-sectional study are generally given as the prevalence rate ratio (PRR), calculated as follows:
PRR = Prevalence rate (among the exposed)/Prevalence rate (among the unexposed)
In the example of AMD among ACEI users, the PRR can be calculated as follows:
PRR = Prevalence of AMD (among ACEI users)/Prevalence of AMD (among ACEI nonusers) = (73/3,654)/(47/3,654) = 1.53
Compared with more intensive observational study designs, a cross-sectional design has the advantage of being less time intensive and cheaper. In addition, it is usually relatively easy to obtain a representative population using this study design. There are, however, some drawbacks to cross-sectional studies.5 First, cross-sectional studies are designed to look at one point in time and cannot determine the temporal sequence (or cause and effect) between risk factor and disease. Second, since the outcome measure of a cross-sectional study is prevalence, there is a potential for incidence-prevalence bias whereby transitory or fatal disease may be preferentially missed. Third, associations made using current exposure status may not be indicative of past
exposure status and therefore may not fit with the established pathophysiology of the disease. Consequently, cross-sectional studies are considered weak Class III evidence for clinical decision making and should only be employed to study preliminary hypotheses.2
exposure status and therefore may not fit with the established pathophysiology of the disease. Consequently, cross-sectional studies are considered weak Class III evidence for clinical decision making and should only be employed to study preliminary hypotheses.2
Case-Control Studies
Unlike cross-sectional studies, case-control studies employ a true control group and can thereby make valid comparisons between groups of patients. In a case-control design, two sample patient populations are identified: those with the outcome in question (the cases) and those without the outcome (the controls). The study then looks backward in time to measure the frequency of past exposure.
Although case-control studies are considered retrospective, patients are theoretically followed forward from the time of exposure in the past to the time of known disease outcome in the present.5 In the analysis, cases are compared to controls with respect to the frequency of the exposure of interest to determine if a cause and effect relationship occurs between exposure and outcome.
An example of a recently published case-control study looked for an association between the use of cholesterol-lowering agents and AMD among a group of 15,792 people enrolled in the Atherosclerosis Risk in Communities study between 1987 and 1989.6 Cases were initially identified as those people found to have AMD after applying a standard definition to their fundus photographs. Controls were participants with no evidence of AMD on fundus photographs. Researchers then established previous exposure to cholesterol-lowering agents through a questionnaire. Exposed patients were those who had used cholesterol-lowering agents during the study period while unexposed patients had never used such agents. The results of case-control studies can be summarized in a simple 2×2 contingency table; an odds ratio (OR) is reported as the measure of association between the exposure and the outcome variable. An analytic example of the OR calculation, its interpretation, and the corresponding statistical tests are discussed in Chapter 2.
In a case-control study, selecting appropriate controls is perhaps the most difficult, yet important, methodological consideration since the control group defines what is normal and provides a basis for comparison. A control group ideally should be picked from a population that is similar to the group of cases in all ways except that they do not have the disease in question. Another methodological consideration in case-control studies is the accurate determination of exposure status. Objective exposures should be used whenever possible to minimize the chance of recall bias* and interviewers should be blinded to minimize the chance of observer bias.†
Since case-control studies are not randomized, there is potential for confounding variables to affect the results of the study. Confounding variables go with the risk factor being investigated and are significantly associated with the disease in question. Therefore, it may look as though the exposure in question causes the disease, when in fact the exposure is simply associated with a confounding factor that causes the disease. The most common example of a confounder in most clinical studies is age. In the example above,6 age is associated with AMD, and older people are also more likely to be on cholesterol-lowering agents. When designing the study and interpreting the results, one must take care to tease out whether cholesterol-lowering agents are temporally associated with AMD after accounting for the effect of age.
Confounding variables can be controlled for in a case-control study during the design phase by matching cases and controls on the basis of known prognostic (or confounding) factors. For instance, if age was thought to be an important confounder, as was the case in the study above,6 then each case would be matched with a control of the same or similar age. Gender is another common factor that cases and controls can be matched on. When matching cannot be accomplished,
stratification is a process of separating a sample into two or more subgroups on the basis of the specified level of a third variable (i.e., the potentially confounding variable) and can be used during the analysis phase of a study to assess the role of confounding. If stratified data are presented in a report, a Mantel-Haenszel OR, which is a combined measure of the stratum-specific ORs, should be reported. Most introductory epidemiology textbooks will provide additional information on this test. A comparable way of accounting for potentially confounding variables that can be a more powerful tool (especially when multiple confounders are considered) is to use a multivariable logistic regression analysis. The principal idea behind both the Mantel-Haenszel OR and multiple logistic regression (which also outputs an OR) is to tease out the effects of the exposure—independent of confounding factors—on the outcome of interest (i.e., provide an adjusted OR). Some basic statistical concepts of logistic regression are discussed further in Chapter 2.
stratification is a process of separating a sample into two or more subgroups on the basis of the specified level of a third variable (i.e., the potentially confounding variable) and can be used during the analysis phase of a study to assess the role of confounding. If stratified data are presented in a report, a Mantel-Haenszel OR, which is a combined measure of the stratum-specific ORs, should be reported. Most introductory epidemiology textbooks will provide additional information on this test. A comparable way of accounting for potentially confounding variables that can be a more powerful tool (especially when multiple confounders are considered) is to use a multivariable logistic regression analysis. The principal idea behind both the Mantel-Haenszel OR and multiple logistic regression (which also outputs an OR) is to tease out the effects of the exposure—independent of confounding factors—on the outcome of interest (i.e., provide an adjusted OR). Some basic statistical concepts of logistic regression are discussed further in Chapter 2.
Case-control studies are most helpful in assessing cause and effect relationships. Logistically, these studies tend to be relatively inexpensive and quick to perform. They are especially well suited for studying rare diseases where incidence rates are low because cases can be selected at the outset. Furthermore, multiple potential risk factors may be studied simultaneously among the same group of cases and controls.
A number of important weaknesses are inherent in case-control studies. First, true incidence rates in exposed and unexposed participants cannot generally be determined.5 Second, case-control studies are not useful in studying rare exposures. Third, information on past exposures or potential confounders may be unknown or incomplete or available information may be different among cases and controls. Finally, it may be difficult to obtain a comparable group of control subjects.
Given these inherent weaknesses, well-designed case-control studies are considered moderate level III evidence.2 However, they can be very useful in clinical decision making regarding cause and effect when used with caution. The case-control study design is appropriate for hypothesis testing, leading to future definitive research, and making inferences about risk factors for rare diseases when controlled clinical trials are unethical or impractical.
Cohort Studies
A cohort is a group of people who are followed up to look for an outcome of interest. In a cohort study, groups of people are identified at the start of the study and are classified as to their exposure status. The exposure of interest could be an environmental factor, a dietary factor, a pharmaceutical treatment, or any intervention.
After the exposure status of each person in the study has been established, two cohorts are naturally formed: a cohort of exposed persons and a cohort of unexposed persons. The two cohorts are then followed up in time to assess the outcome. The outcome measure in a cohort study is the rate or incidence of disease, or the fraction of the cohort that develops disease over a certain period of time. This design differs from a cross-sectional study where the exposure and disease are identified simultaneously and from a case-control study where the outcome is identified first and the presence of past exposure status is compared between the groups.
Cohort studies may be either prospective cohort studies (PCSs) or retrospective cohort studies (RCSs). In a PCS, exposure status is obtained at the beginning of the study and the cohort is observed forward in time for outcomes of interest to occur. At the beginning of the study, participants must be free from the disease outcome and be at risk for developing the outcome sometime in the future. An RCS is similar to a PCS except for the fact that in the exposed cohort the exposure occurred in the past and participants are then traced from the past to the present for disease development. Constructing an RCS requires records of exposure status from the past and appropriate follow-up to obtain outcome status through medical records or disease registries.
In a PCS, the control group is usually the portion of the cohort that is not exposed; this represents an internal comparison. If an
internal comparison group is not possible (for example, among a group of people exposed to an infectious pathogen), then an external comparison group can be used. In an RCS, the comparison group is usually external because a group of exposed persons from the past is followed up to act as the basis for the study. When an external comparison group is used in any study, it is essential that this unexposed group be similar to the exposed group in all ways except for the exposure of interest.5
internal comparison group is not possible (for example, among a group of people exposed to an infectious pathogen), then an external comparison group can be used. In an RCS, the comparison group is usually external because a group of exposed persons from the past is followed up to act as the basis for the study. When an external comparison group is used in any study, it is essential that this unexposed group be similar to the exposed group in all ways except for the exposure of interest.5
An example of a recently published PCS investigated the association between C-reactive protein (CRP) levels (exposure) and the development of AMD (outcome).7 A cohort of 261 patients who had some signs of nonexudative AMD was identified. Inflammatory biomarkers were then collected on each patient, and the cohort was grouped into quartiles on the basis of the CRP level. Patients within the highest quartile of CRP level were defined as exposed, whereas those within the lowest quartile of CRP level were defined as unexposed. The outcome was the incidence of progression of AMD, confirmed on the basis of standardized fundus photographs followed up over a period of 4.6 years.
When critiquing a cohort study, as with any nonrandomized study design, the potential for bias and confounding should be sought out. Bias is minimized by using objective exposure and outcome measures, blinding to exposure and/or outcome status during data collection, and using uniform methods to collect information. Confounding factors must be clearly identified in the study design phase, and data for these factors must be obtained either prospectively or through available records. Confounding variables should then be controlled for either during the design phase of the study or through stratification in the analysis phase with multivariable regression analysis.
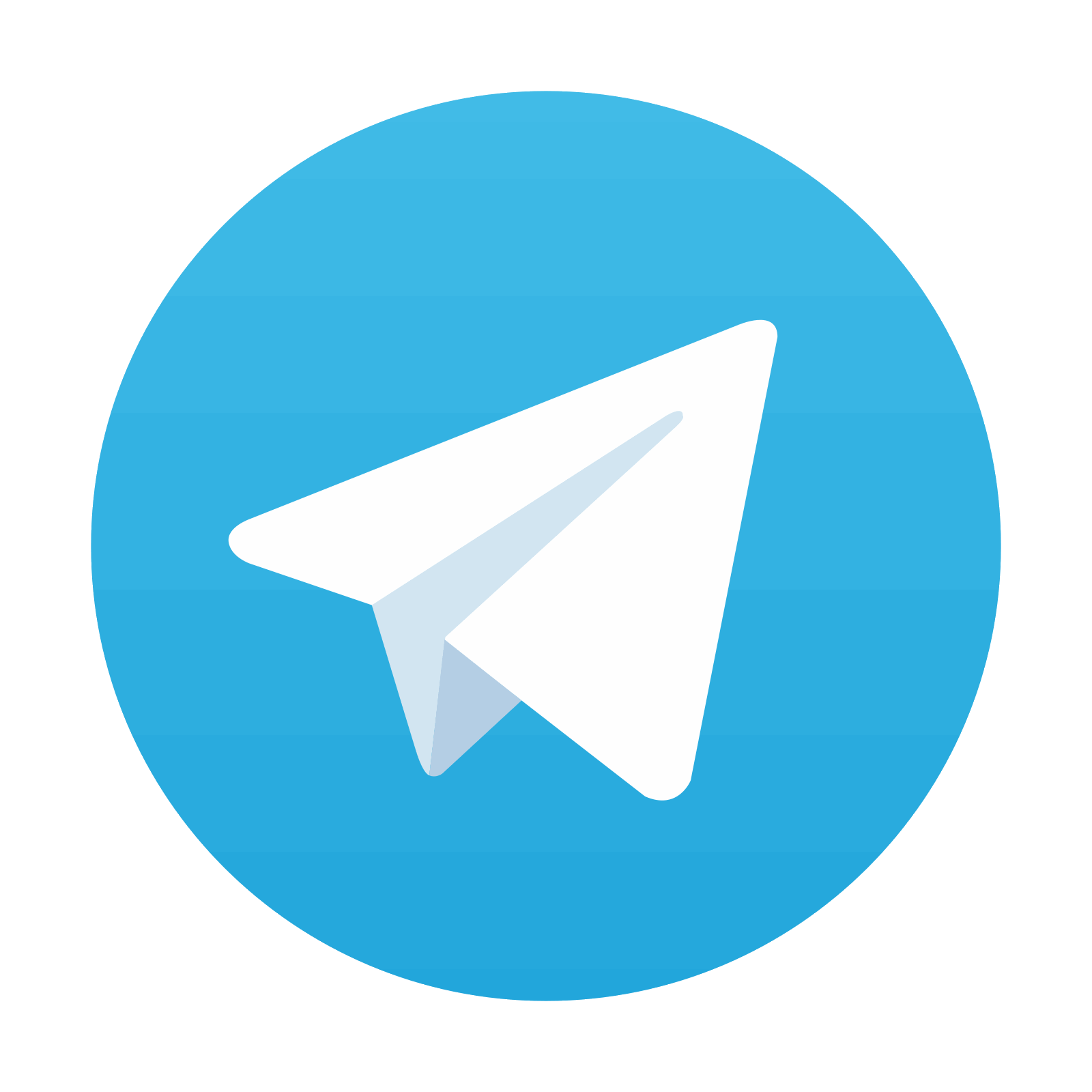
Stay updated, free articles. Join our Telegram channel

Full access? Get Clinical Tree
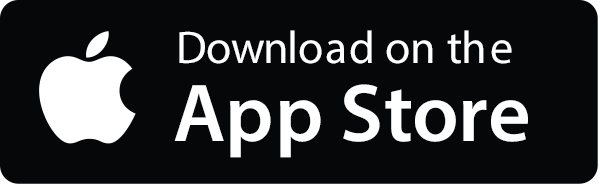
