Purpose
To determine classification criteria for intermediate uveitis, non–pars planitis type (IU-NPP, also known as undifferentiated intermediate uveitis).
Design
Machine learning of cases with IU-NPP and 4 other intermediate uveitides.
Methods
Cases of intermediate uveitides were collected in an informatics-designed preliminary database, and a final database was constructed of cases achieving supermajority agreement on the diagnosis, using formal consensus techniques. Cases were split into a training set and a validation set. Machine learning using multinomial logistic regression was used on the training set to determine a parsimonious set of criteria that minimized the misclassification rate among the intermediate uveitides. The resulting criteria were evaluated on the validation set.
Results
Five hundred eighty-nine of cases of intermediate uveitides, including 114 cases of IU-NPP, were evaluated by machine learning. The overall accuracy for intermediate uveitides was 99.8% in the training set and 99.3% in the validation set (95% confidence interval 96.1, 99.9). Key criteria for IU-NPP included unilateral or bilateral intermediate uveitis with neither snowballs in the vitreous humor nor snowbanks on the pars plana. Other key exclusions included multiple sclerosis, sarcoidosis, and syphilis. The misclassification rates for IU-NPP were 0% in the training set and 0% in the validation set.
Conclusions
The criteria for IU-NPP had a low misclassification rate and seemed to perform well enough for use in clinical and translational research.
T he intermediate uveitides encompass several diseases characterized by the vitreous humor being the primary site of clinically evident inflammation and an absence of choroiditis or retinitis. Intermediate uveitides may be due to infections, such as Lyme disease or syphilis, or associated with systemic diseases, such as sarcoidosis or multiple sclerosis. In the absence of a demonstrable infection or related systemic disease, they are presumed to be eye-limited and immune-mediated. One specific intermediate uveitic disease, pars planitis, was described in 1960 and was characterized by vitritis and pars plana snowbank formation (a collection of fibrinoinflammatory debris). However, not all cases of noninfectious intermediate uveitis without a systemic disease have snowbanks; and these cases sometimes have been lumped with pars planitis and sometimes not, leading to confusion as to what represents pars planitis. At the First International Workshop of the Standardization of Uveitis (SUN) Working Group, it was decided by a supermajority of participants to classify noninfectious intermediate uveitides unassociated with a systemic disease as pars planitis if there were snowballs or snowbanks, and as intermediate uveitis, non–pars planitis type, if there were not. An alternative term for intermediate uveitis, non–pars planitis type would be undifferentiated intermediate uveitis. Intermediate uveitides, including pars planitis, account for up to 15% of uveitis cases in series from tertiary eye care referral centers.
The SUN Working Group is an international collaboration that has developed classification criteria for 25 of the most common uveitic diseases using a formal approach to development and classification. Among the diseases studied was intermediate uveitis, non–pars planitis type.
Methods
The SUN Developing Classification Criteria for the Uveitides project proceeded in 4 phases, as previously described: (1) informatics, (2) case collection, (3) case selection, and (4) machine learning.
Informatics
As previously described, the consensus-based informatics phase permitted the development of a standardized vocabulary and the development of a standardized, menu-driven, hierarchical case collection instrument.
Case Collection and Case Selection
De-identified information was entered into the SUN preliminary database by the 76 contributing investigators for each disease, as previously described. , Cases in the preliminary database were reviewed by committees of 9 investigators for selection into the final database, using formal consensus techniques described in the accompanying article. , Because the goal was to develop classification criteria, only cases with a supermajority agreement (>75%) that the case was the disease in question were retained in the final database (ie, were “selected”). ,
Machine Learning
The final database then was randomly separated into a training set (∼85% of cases) and a validation set (∼15% of cases) for each disease, as described in the accompanying article. Machine learning was used on the training set to determine criteria that minimized misclassification. The criteria then were tested on the validation set; for both the training set and the validation set, the misclassification rate was calculated for each disease. The misclassification rate was the proportion of cases classified incorrectly by the machine learning algorithm when compared to the consensus diagnosis. For intermediate uveitis, non–pars planitis type, the diseases against which it was evaluated were multiple sclerosis–associated intermediate uveitis, pars planitis, sarcoid intermediate uveitis, and syphilitic intermediate uveitis. Too few cases of Lyme disease uveitis (14) were collected in the database for analysis by machine learning.
The study adhered to the principles of the Declaration of Helsinki. Institutional review boards at each participating center reviewed and approved the study; the study typically was considered either minimal risk or exempt by individual institutional review boards.
Results
Two hundred nine cases of intermediate uveitis, non–pars planitis type were collected, and 114 (55%) achieved supermajority agreement on the diagnosis during the “selection” phase and were used in the machine learning phase. These cases of intermediate uveitis, non–pars planitis type were compared to cases of other intermediate uveitides, including 112 cases of multiple sclerosis–associated intermediate uveitis, 226 cases of pars planitis type, 52 cases of sarcoidosis-associated intermediate uveitis, and 85 cases of syphilitic intermediate uveitis. The details of the machine learning results for these diseases are outlined in the accompanying article. The characteristics at presentation to a SUN Working Group investigator of cases with intermediate uveitis, non–pars planitis type are listed in Table 1 . The criteria developed after machine learning are listed in Table 2 . Key features are the presence of inflammation primarily in the vitreous humor, absence of snowballs and snowbanks, and the exclusion of syphilis, multiple sclerosis, and sarcoidosis. The overall accuracy for intermediate uveitides was 99.8% in the training set and 99.3% in the validation set (95% confidence interval 96.1, 99.2). The misclassification rate for intermediate uveitis, non–pars planitis type was 0% in the training set and 0% in the validation set.
Characteristic | Result |
---|---|
Number of cases | 114 |
Demographics | |
Age, median, years (25th, 75th percentile) | 37 (23, 52) |
Sex (%) | |
Male | 37 |
Female | 63 |
Race/ethnicity (%) | |
White, non-Hispanic | 68 |
Black, non-Hispanic | 5 |
Hispanic | 4 |
Asian, Pacific Islander | 3 |
Other | 8 |
Missing | 12 |
Uveitis history | |
Uveitis course (%) | |
Acute, monophasic | 4 |
Acute, recurrent | 4 |
Chronic | 86 |
Indeterminate | 6 |
Laterality (%) | |
Unilateral | 29 |
Unilateral, alternating | 0 |
Bilateral | 71 |
Ophthalmic examination | |
Keratic precipitates (%) | |
None | 82 |
Fine | 13 |
Round | 3 |
Stellate | 0 |
Mutton fat | 1 |
Other | 1 |
Anterior chamber cells, grade (%) | |
0 | 59 |
½+ | 17 |
1+ | 16 |
2+ | 7 |
3+ | 2 |
4+ | 0 |
Hypopyon (%) | 0 |
Anterior chamber flare, grade (%) | |
0 | 82 |
1+ | 16 |
2+ | 3 |
3+ | 0 |
4+ | 0 |
Iris (%) | |
Normal | 91 |
Posterior synechiae | 9 |
Sectoral iris atrophy | 0 |
Patchy iris atrophy | 0 |
Diffuse iris atrophy | 0 |
Heterochromia | 0 |
IOP, involved eyes | |
Median, mm Hg (25th, 75th percentile) | 14 (12, 17) |
Proportion of patients with IOP > 24 mm Hg either eye (%) | 4 |
Vitreous cells, grade (%) a | |
0 | 3 |
½+ | 14 |
1+ | 39 |
2+ | 35 |
3+ | 9 |
4+ | 1 |
Vitreous haze, grade (%) a | |
0 | 31 |
½+ | 14 |
1+ | 34 |
2+ | 17 |
3+ | 3 |
4+ | 2 |
Vitreous snowballs b | 0 |
Pars plana snowbanks b | 0 |
Peripheral retinal vascular sheathing or leakage | 19 |
Macular edema | 47 |
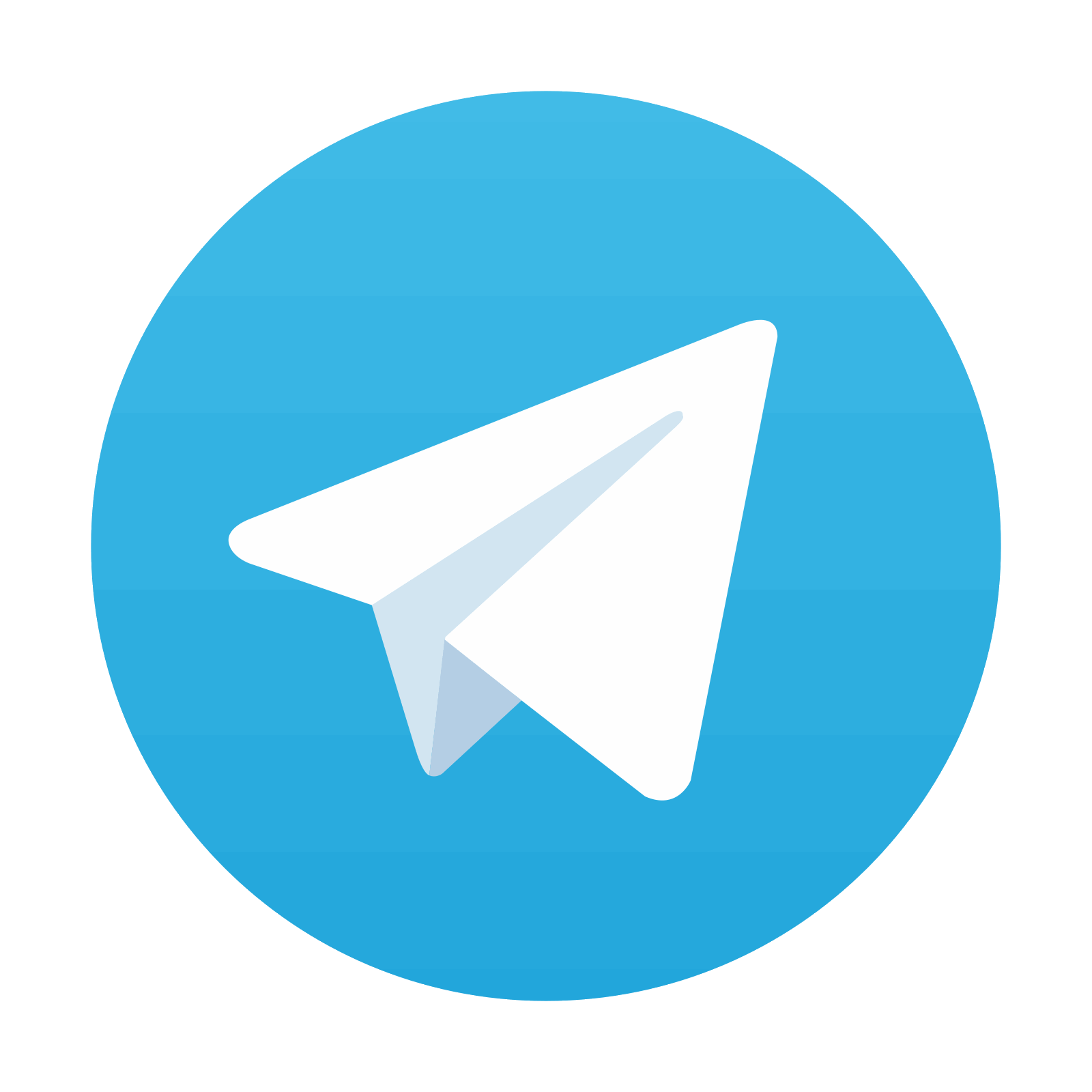
Stay updated, free articles. Join our Telegram channel

Full access? Get Clinical Tree
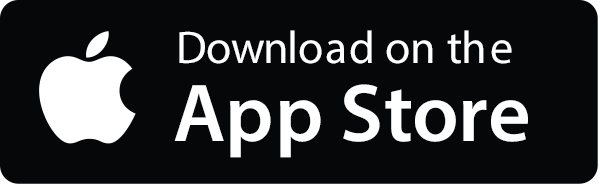

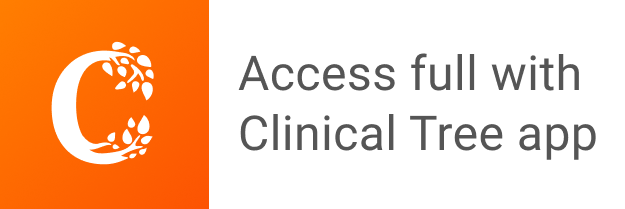