Purpose
To determine classification criteria for cytomegalovirus (CMV) anterior uveitis.
Design
Machine learning of cases with CMV anterior uveitis and 8 other anterior uveitides.
Methods
Cases of anterior uveitides were collected in an informatics-designed preliminary database, and a final database was constructed of cases achieving supermajority agreement on the diagnosis, using formal consensus techniques. Cases were split into a training set and a validation set. Machine learning using multinomial logistic regression was used on the training set to determine a parsimonious set of criteria that minimized the misclassification rate among the anterior uveitides. The resulting criteria were evaluated on the validation set.
Results
One thousand eighty-three cases of anterior uveitides, including 89 cases of CMV anterior uveitis, were evaluated by machine learning. The overall accuracy for anterior uveitides was 97.5% in the training set and 96.7% in the validation set (95% confidence interval 92.4, 98.6). Key criteria for CMV anterior uveitis included unilateral anterior uveitis with a positive aqueous humor polymerase chain reaction assay for CMV. No clinical features reliably diagnosed CMV anterior uveitis. The misclassification rates for CMV anterior uveitis were 1.3% in the training set and 0% in the validation set.
Conclusions
The criteria for CMV anterior uveitis had a low misclassification rate and seemed to perform sufficiently well for use in clinical and translational research.
W ith the advent of clinically available polymerase chain reaction (PCR) methodology for detecting cytomegalovirus (CMV) DNA, it became evident that CMV could cause an anterior uveitis in immunocompetent individuals. , The pathogenesis appears to be due to replicating CMV in the eye, as evidenced by the detection of CMV DNA and RNA in the anterior chamber using PCR on aqueous specimens obtained by paracentesis. The disease is distinct from CMV retinitis seen in immunocompromised patients. Although CMV anterior uveitis has been reported from multiple countries, most reports come from Asian countries. In the United States, it is estimated to cause ∼2% of cases of viral anterior uveitis. , Whether this regional variation represents environmental factors, genetic susceptibility, or a combination of factors is unknown at this time.
CMV anterior uveitis has several clinical appearances, including a recurrent acute hypertensive anterior uveitis consistent with Posner-Schlossman syndrome, a Fuchs uveitis syndrome (FUS)-like chronic anterior uveitis, and less frequently a recurrent or chronic anterior uveitis with iris atrophy. , , Adding to the difficulty of diagnosis is that none of these phenotypes is consistently attributable to CMV. In regions with a high prevalence of CMV anterior uveitis, ∼50% of Posner-Schlossman patients will have CMV anterior uveitis, and ∼40% of FUS-like eyes will have CMV anterior uveitis. No differences in phenotype can be detected between eyes with Posner-Schlossman syndrome with and without CMV, and although there are differences between eyes with FUS and with FUS-like CMV anterior uveitis, they do not seem to be consistent enough for reliable diagnosis. Eyes with FUS-like CMV anterior uveitis are more likely to have endotheliitis, nodular or “coin-like” endothelial lesions, and iris atrophy without heterochromia. , , , The importance of correct diagnosis is emphasized by the clinical response of CMV anterior uveitis to topical ganciclovir therapy, including better intraocular pressure control, inflammation control, and diminishing endothelial cell loss. ,
The Standardization of Uveitis Nomenclature (SUN) Working Group is an international collaboration that has developed classification criteria for 25 of the most common uveitides using a formal approach to development and classification. Among the anterior uveitides studied was CMV anterior uveitis.
Methods
The SUN Developing Classification Criteria for the Uveitides project proceeded in 4 phases, as previously described: (1) informatics, (2) case collection, (3) case selection, and (4) machine learning. , ,
Informatics
As previously described, the consensus-based informatics phase permitted the development of a standardized vocabulary and the development of a standardized, menu-driven hierarchical case collection instrument.
Case Collection and Case Selection
De-identified clinical information was entered into the SUN preliminary database by the 76 contributing investigators for each disease, as previously described. , Cases in the preliminary database were reviewed by committees of 9 investigators for selection into the final database, using formal consensus techniques described in the accompanying article. , Because the goal was to develop classification criteria, only cases with a supermajority agreement (>75%) that the case was the disease were retained in the final database (ie, were “selected”).
Machine Learning
The final database then was randomly separated into a training set (∼85% of cases) and a validation set (∼15% of cases) for each disease as described in the accompanying article. Machine learning was used on the training set to determine criteria that minimized misclassification. The criteria then were tested on the validation set; for both the training set and the validation set, the misclassification rate was calculated for each disease. The misclassification rate was the proportion of cases classified incorrectly by the machine learning algorithm when compared to the consensus diagnosis. For CMV anterior uveitis, the diseases against which it was evaluated were herpes simplex virus (HSV) anterior uveitis, varicella zoster virus (VZV) anterior uveitis, juvenile idiopathic arthritis–associated anterior uveitis, spondylitis/HLA-B27-associated anterior uveitis, tubulointerstitial nephritis with uveitis, FUS, sarcoidosis-associated anterior uveitis, and syphilitic anterior uveitis.
Comparison of Cases From Asian Patients and Non-Asian Patients
For categorical variables, comparison of cases of CMV anterior uveitis in Asian and non-Asian patients was performed with the χ 2 test or the Fisher exact test if a cell was less than 5. For continuous variables, the Wilcoxon rank sum test was used. P values are nominal and 2-sided.
The study adhered to the principles of the Declaration of Helsinki. Institutional review boards at each participating center reviewed and approved the study; the study typically was considered either minimal risk or exempt by the individual institutional review boards.
Results
One hundred twelve cases of CMV anterior uveitis were collected, and 89 cases (79%) achieved supermajority agreement on the diagnosis during the “selection” phase and were used in the machine learning phase. These cases of CMV anterior uveitis were compared to 994 cases of other anterior uveitides, including 123 cases of VZV anterior uveitis, 101 cases of HSV anterior uveitis, 146 cases of FUS, 202 cases of juvenile idiopathic arthritis–associated anterior uveitis, 184 cases of spondylitis/HLA-B27-associated anterior uveitis, 94 cases of tubulointerstitial nephritis with uveitis, 112 cases of sarcoidosis-associated anterior uveitis, and 32 cases of syphilitic anterior uveitis. The characteristics of the cases with CMV anterior uveitis at presentation to a SUN Working Group investigator are listed in Table 1 . The comparison of characteristics of Asian and non-Asian cases is shown in Table 2 . Differences between Asian and non-Asian cases included (1) slightly older age in Asian cases; (2) greater proportion of cases with chronic uveitis in Asian cases; and (3) greater proportion of cases with mutton fat or nummular keratic precipitates ( Figure 1 ) in Asian cases. The criteria developed after machine learning are listed in Table 3 . No phenotypic features were selected, and detection of CMV in the aqueous humor with PCR was selected by machine learning as necessary for diagnosis. The 1 case selected by the selection committee for inclusion in the final database without a positive PCR for CMV had an acute hypertensive anterior uveitis with corneal edema and elevated intraocular antibodies to CMV on Goldmann-Witmer analysis of an aqueous humor sample obtained by paracentesis. The overall accuracy for anterior uveitides was 97.5% in the training set and 96.7% in the validation set (95% confidence interval 92.4, 98.6). The misclassification rate for CMV anterior uveitis in the training set was 1.3% and in the validation set 0%.
Characteristic | Result |
---|---|
Number of cases | 89 |
Demographics | |
Age, median, years (25th, 75th percentile) | 53 (39, 62) |
Age category, years (%) | |
≤16 | 2 |
17-50 | 39 |
51-59 | 29 |
≥60 | 29 |
Sex (%) | |
Male | 65 |
Female | 35 |
Race/ethnicity (%) | |
White, non-Hispanic | 25 |
Black, non-Hispanic | 8 |
Hispanic | 2 |
Asian, Pacific Islander | 43 |
Other | 9 |
Missing/unknown | 13 |
Uveitis history | |
Uveitis course (%) | |
Acute, monophasic | 7 |
Acute, recurrent | 37 |
Chronic | 55 |
Indeterminate | 1 |
Laterality (%) | |
Unilateral | 97 |
Unilateral, alternating | 0 |
Bilateral | 3 |
Ophthalmic examination | |
Cornea (%) | |
Normal | 80 |
Corneal edema | 19 |
Keratitis | 1 |
Keratic precipitates (%) | |
None | 10 |
Fine | 27 |
Round | 33 |
Stellate | 8 |
Mutton fat | 17 |
Numular | 6 |
Anterior chamber cells, grade (%) | |
½+ | 28 |
1+ | 34 |
2+ | 22 |
3+ | 2 |
4+ | 0 |
Missing | 14 |
Hypopyon (%) | 0 |
Anterior chamber flare, grade (%) | |
0 | 61 |
1+ | 29 |
2+ | 10 |
3+ | 0 |
4+ | 0 |
Iris (%) | |
Normal | 69 |
Posterior synechiae | 9 |
Sectoral iris atrophy | 2 |
Patchy iris atrophy | 9 |
Diffuse iris atrophy | 15 |
Heterochromia | 2 |
IOP, involved eyes | |
Median, mm Hg (25th, 75th percentile) | 18 (14, 30) |
Proportion of patients with IOP > 24 mm Hg either eye (%) | 54 |
Vitreous cells, grade (%) | |
0 | 93 |
½+ | 3 |
1+ | 1 |
2+ | 2 |
3+ | 0 |
4+ | 0 |
Laboratory | |
Aqueous PCR positive for CMV a (% cases) | 99 |
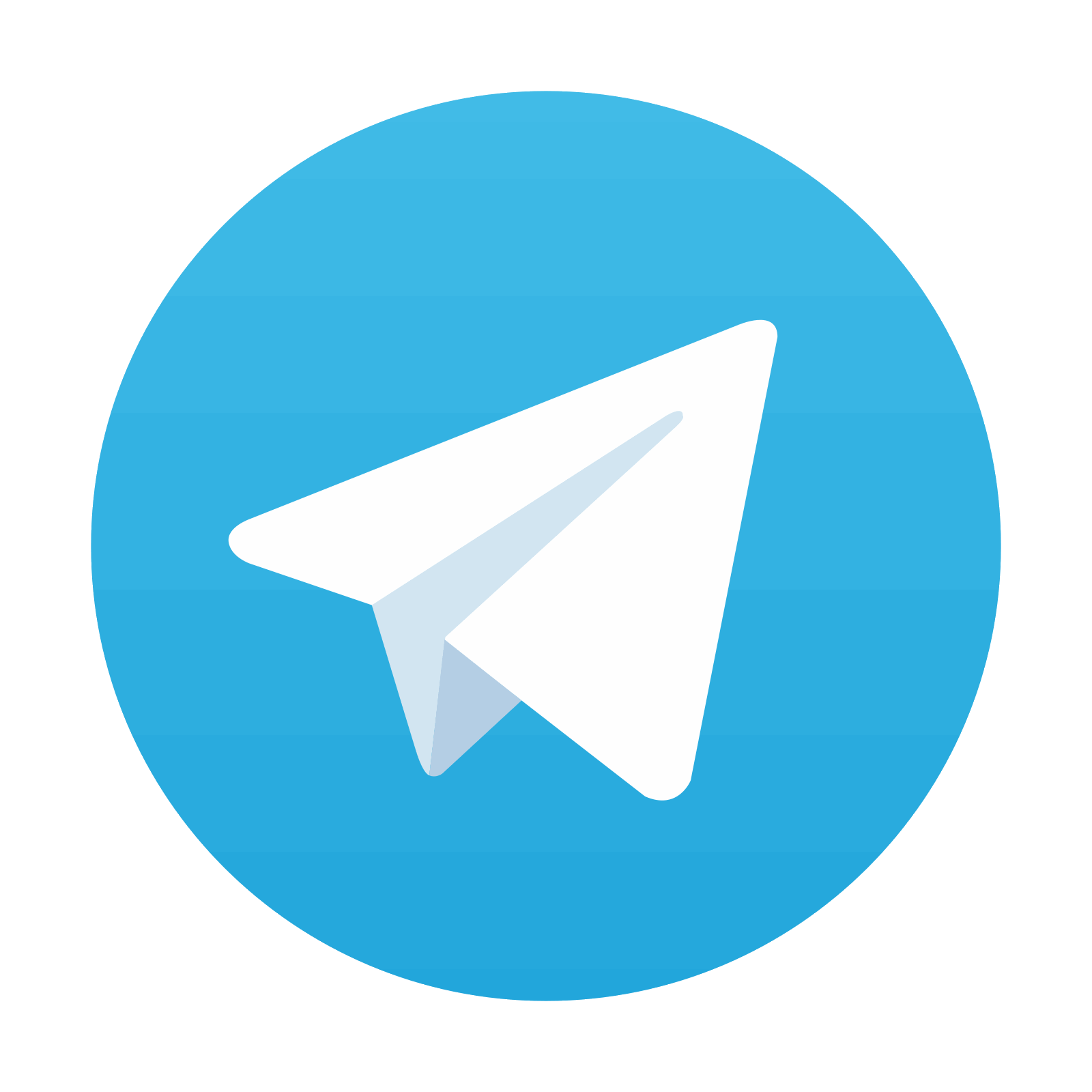
Stay updated, free articles. Join our Telegram channel

Full access? Get Clinical Tree
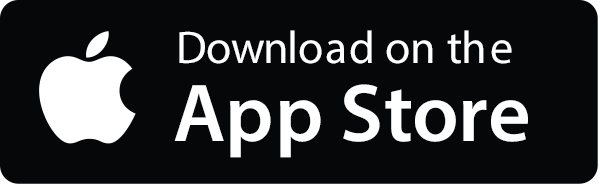

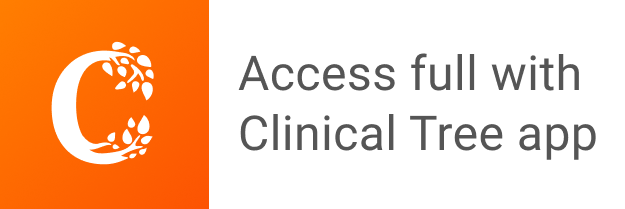