What causes age-related macular degeneration (AMD)? Numerous literature reviews have addressed this question by providing perfunctory tallies of numerous hypothesized risk factors. In so doing, such reviews often fail to present an integrated perspective regarding the etiology of AMD. The complex theoretical concepts of disease causation underlying the field of epidemiology are bypassed in favor of the more quantitative concepts of odds, risks, and rates. This may not be surprising in that there is a certain comfort in numbers, a comfort that perhaps allows the epidemiologist to consider himself a peer to his experimental colleague, for whom the phrase “cause-and-effect” comes much easier. Epidemiology has a vested interest in causation as, despite its numerous and often vague definitions, it is a discipline with the goal of identifying causes of disease (both modifiable and nonmodifiable) so that the disease or its consequences might be prevented. Yet, notions of causation are often not well integrated into the practice of epidemiology. The reasons for this are varied and include the fact that traditional causal inference is largely focused on conditions with a single etiology whose application to complex diseases is problematic. As a byproduct, the neophyte epidemiologist is frequently taught that the word “cause” is to be avoided. And while the principles of causation appear in nearly every introductory epidemiology textbook, this material often appears as a clumsy appendage, not well integrated into the concepts of study design, analysis, and interpretation. Yet there have been significant developments with respect to causation in epidemiology such that the gulf between theoretical and applied epidemiology is easily traversed.
The objective of this editorial is a practical one: that is, to aid the reader with the integration of causation into the practice of epidemiology. This will be accomplished by describing the prevailing models and approaches to causation in the field as well as an emerging tool that serves to link the philosophical notion of causation with everyday epidemiologic research.
Prevailing Models of Causation in Epidemiology
There are a multitude of definitions and models of causation in epidemiology, yet space precludes a thorough presentation of this material herein; fortunately, this topic has been covered in adequate detail elsewhere. Many of the currently debated definitions and models have their roots in the purely deterministic model of disease causation described by Henle and Koch in the late 19th century. They describe 4 criteria necessary to establish a casual relationship between a microbe and a disease; among these was that an exposure must occur in all cases of the disease while being absent in those not so afflicted. Such a requirement represents a significant burden for establishing causation and was quickly abandoned with the recognition that causal relationships are rarely, if ever, monogamous. Over the next hundred years the notion of causation evolved with the recognition that disease etiology is truly multifactorial and encompasses not just the agent but also the host and environment, all acting in a coordinated, web-like manner. Moreover, the appreciation that the definition of agent could be expanded to include nonbiologic entities opened the door to the application of causal models to chronic diseases. The concept of causation in epidemiology continues to evolve, albeit on a more theoretical level and, as some have argued, at a stagnated pace perhaps attributable to the esoteric nature of the current debate.
Adaptations in the concept of causation in epidemiology have been fostered by observations that diseases are attributable to not 1 but a number of unique, perhaps overlapping, causal mechanisms. These mechanisms are composed of multiple components, no 1 of which may be sufficient or necessary to cause a given disease. Rather, it is a mosaic of components acting together (though not necessarily contemporaneously) that are required to produce disease. To illustrate this concept, each of the circles in Figure 1 represents a sufficient cause of disease in an individual. Each hypothetical causal mechanism (ie, sufficient cause) is composed of individual components or factors that, when present, are sufficient to induce disease. No 1 of these components or factors alone is adequate to produce disease and many, if not most, may be unknown. Furthermore, some factors (eg, A) play a role in more than 1 mechanism, whereas others are specific to only 1 (eg, J). A factor or component cause that is present in every sufficient cause is referred to as necessary. Necessary causes are often more readily identified for infectious diseases (eg, tubercles bacillus is a necessary cause for tuberculosis) than for chronic diseases.
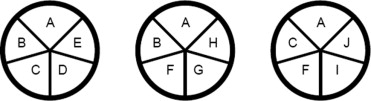
The practical implications of this model of causation are best illustrated with an example. With respect to AMD, an abundance of risk factors or component causes have been postulated and tested. Among these, age and smoking are the most consistently identified risk factors or component causes. Yet these do not reach the level of necessary causes, as not all older adults or smokers develop AMD. Yet, the consistency with which smoking has been observed from 1 study to the next might suggest that it plays a role in a larger number of sufficient causes or causal mechanisms (eg, A), whereas those risk factors with less consistent (or weaker) associations, such as obesity, might be those that play a role in a smaller number of sufficient causes (eg, J). It is important to note that the operationalization of sufficient causes in an epidemiologic investigation is subject to study design limitations (eg, recall and selection bias). These may overwhelm the ability to detect real associations, resulting in heterogeneous results from 1 study to the next. This heterogeneity might also be attributed to the failure to incorporate pertinent component causes or factors into the study or analysis. Thus, role of nutrient intake (eg, B) or genetic predisposition (eg, C) may not become apparent until smoking (eg, A) is taken into account.
In the context of study design, it aids in the process of cataloging the information that needs to be collected regarding study participants (eg, smoking, nutrient intake, genetic susceptibility). When interpreting analytic results, this model is consistent with the observation that despite a strong association between a given exposure and disease, some diseased individuals remain unexposed. Additionally, when integrating the results of a body of research, the relative strength of association across hypothesized risk factors can aid in depicting the extent to which they participate in multiple causal mechanisms. When combined with the prevalence of the risk factor, measures of effect such as attributable risk, though infrequently used, can serve as a guide as to the relative importance of hypothesized causal factors.
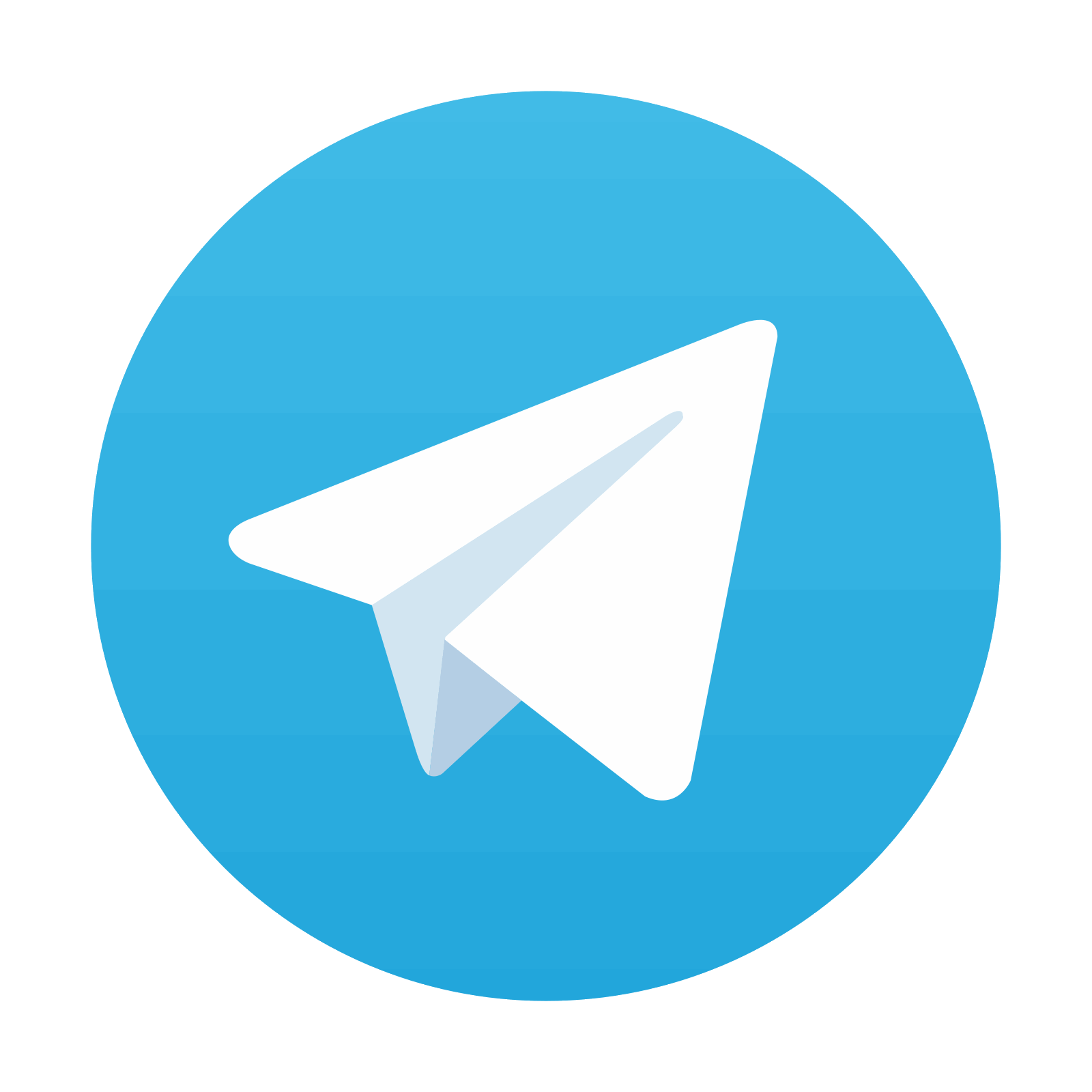
Stay updated, free articles. Join our Telegram channel

Full access? Get Clinical Tree
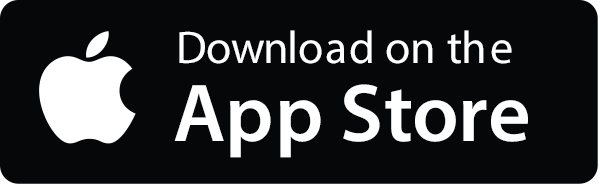
