Blood Flow Quantitation with Arterial Spin Labeling
Laura M. Parkes
Hanzhang Lu
Esben T. Petersen
Theory
Kinetic Modeling
One of the advantages of arterial spin labeling (ASL) over other techniques for measuring perfusion is the possibility of accurate quantification. Blood perfusion and arterial transit time (time taken for blood to travel from the labeling region to tissue voxel) can be estimated.
We start with the basic difference images (control – label), in which the signal is dependent on perfusion. However, a number of other factors contribute to the difference signal, and these need to be taken into account to provide an accurate estimate of perfusion.
Details of the acquisition protocol can cause unwanted signal in the difference image that is unrelated to perfusion, for example, magnetization transfer differences between label and control images. Likewise, some true perfusion signal can be missed, for example, the passage of nonlabeled blood due to the restricted spatial extent of labeling. For the purposes of this chapter, it is assumed that the signal in the difference image contains contributions from all perfusing blood, and only from perfusing blood (i.e., ignoring the nonperfusion and missed perfusion contributions described above).
The difference signal can be collected at either a single time point after labeling or at multiple time points, as shown in Figure 30.1. This time is often referred to as the inversion time or the postlabeling delay time.
![]() FIGURE 30.1. The difference in image for an adult brain at a range of inversion times. The signal can be seen to move from the large vessels into the capillaries and then decay. |
Bloch Equation Approach
The signal in the difference images as shown in Figure 30.1 is related to perfusion, but also clearly depends on the inversion time. To get an accurate estimate of perfusion, the difference signal as a function of time can be modeled, and the model can be fit to the data in order to estimate perfusion.
The most common approach is to use a single-compartment model (Fig. 30.2), where it is assumed that the labeled water diffuses freely throughout the tissue voxel. One approach to modeling the ASL signal is to modify the Bloch equation.1 The standard Bloch equation describes the decay of longitudinal magnetization, M, by the time constant T1, and is given by:

where M0 is the equilibrium magnetization. The effects of the labeled blood can be incorporated by adding two additional terms to Equation 1: f · Ma describing the flow of magnetization into the voxel and f · Mv describing the reduction in magnetization caused by labeled blood flowing out of the voxel:

where:
M(t) is the total magnetization in the tissue voxel of the labeled image at time t after labeling (units of magnetization per milliliter of tissue)
Ma(t) is the arterial magnetization (units of magnetization per milliliter of blood)
Mv(t) is the venous magnetization (units of magnetization per milliliter of blood), and
f is perfusion (units of milliliters of blood, per unit time per milliliter of tissue).
In the single compartment model, it is assumed that water instantaneously equilibrates throughout the voxel such that the magnetization of the blood leaving the voxel (Mv) is proportional to the average magnetization of the whole voxel (M(t)).
Defining the Blood–Tissue Partition Coefficient: The volume of water in the tissue is however less than that in blood, by a constant known as the blood–brain partition coefficient, λ.
So Mv is greater than M(t) by the same proportion, that is, Mv(t) = M(t)/λ. As discussed later in the chapter, λ can also include a correction factor for tissue density, allowing perfusion to be expressed in terms of milliliter per minute per 100 g. Substituting this into Equation 2, we find:

where

Hence, the signal in the labeled image can be seen to decay with the relaxation time T1app, which is shortened in comparison to T1 due to the presence of labeled blood.
Earlier work considered the application of a continuous labeling pulse,1,2 which was applied until the system reached steady state. In this case, there was found to be a very simple relation between perfusion, f, and the signal in the labeled and control images (see Box 30.1 for derivation):

where ΔM is the difference in signal between the control and labeled images and M0 is the equilibrium signal in the control image. Here, A relates to the difference in arterial magnetization between the label and control images and is defined as 1 for continuous saturation1 and 2 for continuous inversion.2 In practice, A is reduced because of imperfect labeling efficiency, which should be taken into account for accurate quantification. The labeling efficiency can be expressed as α, a number between 0 and 1, where 1 is perfect inversion.
Box 30.1 The Steady State CASL Signal
Although earlier work considered the signal separately in the control and labeled image,1,2 it is simpler to consider the signal directly in the difference image, ΔM (i.e., control – label, rendering the signal positive). In the absence of labeling, the equilibrium magnetization in the difference image is zero, and so the M0 term in Equation 1 can be set to zero. Propagating through to Equation 3 we find that:

In the steady state, it can be assumed that the difference in arterial magnetization between the “control” and “label” image, Ma, is given by A·Ma0, where Ma0 is the equilibrium magnetization of arterial blood. This assumes no longitudinal relaxation of the signal on transit from the labeling plane to the imaging plane. In the steady state, Equation 6 is equal to zero, giving:

This is equivalent to Equation 5 based on the assumption that Ma0 = M0/λ, which is the general definition of the partition coefficient.3
Equation 5 is only applicable for a continuous ASL (CASL) experiment where labeling is continuously applied until a steady state is reached. In addition, it is assumed that the effect of T1-relaxation during the transit of blood from the labeling to the imaging plane is negligible. This is not the case, and furthermore, the transit time to different parts of the image could be different, resulting in errors of relative regional perfusion. Thus, a more general model is required.
A more complex expression for Ma(t) can be incorporated into Equation 6 to include the temporal width of the bolus, τ, which is simply the labeling time in a CASL experiment, and T1 relaxation during the arterial transit time, ta:

This is for the case of a pulsed ASL (PASL) experiment using an inversion pulse at t = 0 with labeling efficiency α. Note here that A as used in Equations 5 and 7 has been
replaced by 2α to describe imperfect (α) inversion (2). The arterial magnetization decays exponentially with the T1 relaxation time of blood, T1a, from the time of labeling; hence, the exponential decay term in Equation 8.
replaced by 2α to describe imperfect (α) inversion (2). The arterial magnetization decays exponentially with the T1 relaxation time of blood, T1a, from the time of labeling; hence, the exponential decay term in Equation 8.
For a CASL experiment, the exponential term includes the arterial transit time ta, as all labeled blood will take the same time, ta, to reach the tissue and the amplitude of the label does not change over the observation interval. This is in contrast to PASL, where the label is applied over a volume in space and the trailing edge will have decayed more than the leading edge as it takes longer to reach the tissue voxel. Figure 30.3 shows the time course of Ma(t) for both a PASL and CASL experiment. Equation 8 can be substituted into Equation 6 and solved to give a more general description of ΔM.4,5 It should be noted that this form of Ma(t) assumes plug flow of the arterial blood from the labeling to the measurement site with no dispersion.
General Kinetic Model
A more intuitive approach is to consider instead a bolus of labeled spins traveling through the vasculature.6 Here, the ASL signal is considered more in terms of a microsphere experiment, delivering a bolus of labeled spins to the tissue. It is conceptually different from the single-compartment Bloch equation approach, as described above, which assumes the labeled water acts as a freely diffusible tracer. It is argued that this approach is more appropriate,7 as, during the time scale of a typical ASL experiment, there is little time for the labeled water to equilibrate throughout the voxel. Neither is there time for appreciable labeled water to leave the voxel, and hence the label can be considered as trapped in the tissue voxel, as in a microsphere experiment.
In this approach, ΔM(t) is given by:

where Ma(t) is again the arterial magnetization, describing the delivery of labeled spins to the tissue voxel; m(t) is a relaxation term describing the fraction of (labeled) magnetization remaining after time t, and r(t) is a residue term describing the fraction of molecules remaining in the voxel after time t (i.e., that have not left the voxel through the venous outlet and can still contribute to the signal).
If single compartment kinetics are assumed, then r(t) = exp(−ft/λ), and m(t) = exp(−t/ T1).6 In this case, the approach is equivalent to the Bloch equation approach and produces the same solutions for ΔM(t).8 In order to solve for ΔM(t), we need to define Ma. As described earlier, a general form for Ma is given in Equation 8.
The reader if referred to Buxton et al. for the mathematical form of the solutions for ΔM(t). The solutions contain eight unknown parameters, namely:
f: perfusion
ta: arterial transit time
τ: bolus width
λ: blood-tissue partition coefficient
Ma0: equilibrium magnetization of arterial blood
T1a: longitudinal relaxation time of blood
T1: longitudinal relaxation time of tissue
α: labeling efficiency
The latter five parameters in the list are all either measured from a separate experiment (sometimes using the ASL data) or literature values are assumed. This leaves three remaining unknown parameters: (a) perfusion, f; (b) arterial transit time, ta; and (c) the bolus width, τ. The forms of the solutions for ΔM(t) for a PASL experiment (Equation 3 from Buxton et al.) are given in Figure 30.4 for varying perfusion (a), arterial transit time (b), and bolus width (c). It can be seen that the signal in the
difference image is sensitive not only to perfusion (a), but also to the arterial transit time (b), and the bolus width (c). If corrections are not made for these parameters, then the perfusion estimate will be inaccurate. The estimation of these parameters will be described in detail later in this chapter.
difference image is sensitive not only to perfusion (a), but also to the arterial transit time (b), and the bolus width (c). If corrections are not made for these parameters, then the perfusion estimate will be inaccurate. The estimation of these parameters will be described in detail later in this chapter.
Multicompartment Models
In reality, the labeled blood water does not exchange immediately with the extravascular water. Labeled water will remain in the vessel for some time before crossing the capillary wall into the extravascular space. This causes two inaccuracies to the single-compartment model.5 First, it is assumed that as soon as labeled water enters the tissue voxel, it relaxes with the T1 relaxation time of tissue. In fact, it will first relax with the T1 relaxation time of blood before moving into the extravascular space (Fig. 30.5). The T1 relaxation time of blood is longer than the T1 relaxation time of tissue, so the signal decay will be slower than predicted by the single-compartment model, causing an overestimation of perfusion (i.e., the T1 effect). Second, the assumption that Mv(t) = M(t)/λ is also incorrect because the exchange of water between blood and tissue may not have reached equilibrium. In fact, some of the labeled water in large vessels will pass directly through the vasculature without exchanging at all, and so the venous magnetization will be greater than predicted by the single-compartment model, causing an underestimation of perfusion (i.e., the outflow effect).
There have been a number of approaches attempting to correct for these errors. Most studies have extended the Bloch equation approach, although it is also possible to relax the assumptions of the general kinetic model.6 A relatively simple correction is to consider the inclusion of an arterial blood compartment within the voxel.9 Here, labeled water first enters this compartment, relaxing with the T1 relaxation time of blood, and then crosses into the tissue compartment at a fixed exchange time, tex, correcting for the T1 effect. A more general approach is to incorporate restricted permeability between the blood and tissue compartments.5,10,11 Incorporating restricted permeability is a more accurate description of the system, as water molecules will gradually leave the capillaries over a period of time, whereas a fixed exchange time means instantaneous exchange, albeit at a certain time after the molecules have entered the tissue voxel. These models introduce additional unknown parameters and, given the poor signal-to-noise ratio of the ASL technique, it is difficult to fit for them from the data. The permeability–surface area product (PS) can be incorporated into a two-compartment model5 and PS values, which have been measured using alternative approaches, can be taken from literature. The exchange time, however, is not such a well-defined physiologic parameter and literature values are not available.
In certain cases, the assumption of zero exchange of water across the capillary wall may be more accurate than the assumption of free exchange—a single blood compartment model. This produces very simple solutions for ΔM(t)5 and allows perfusion estimation without the need for a measurement of T1 relaxation times of tissue. The central assumption is that the measurement is made before the labeled water leaves the blood, which has been shown to occur at approximately 2 seconds in the adult human brain.12 Hence, this approach will only be accurate for techniques with a short (i.e., less than 2 second) measurement time, such as pulsed techniques.
Although an improvement on the single-compartment model, the two-compartment models described above are still inaccurate because of the assumption of a well-mixed blood compartment. Two studies13,14 have tackled this by allowing the concentration of labeled water in the blood compartment to change as a function of both time and distance along the capillary.
The accuracy of the various models depends on a number of factors, namely, (a) the field strength, (b) the tissue type, (c) the measurement time (i.e., postlabeling delay time), and (d) the perfusion rate itself. With increasing field strength, T1 relaxation becomes slower and so the magnetization carried away by the venous blood is larger, hence the outflow effect is more significant. In white matter, the T1 effect is larger than for gray matter because there is a larger difference between the T1 relaxation times of blood and tissue in this case. It is also important to note that as the measurement time increases, both the T1 effect and the outflow effect become more significant as labeled water has more chance of (a) crossing the capillary wall and (b) flowing out of the voxel. Finally, the perfusion rate itself will also affect the accuracy of the model. Higher perfusion rates reduce the
transit time through the voxel and, thus, increase the outflow effect.
transit time through the voxel and, thus, increase the outflow effect.
In general, for human studies of cerebral gray matter with short measurement times (<3 seconds postlabeling delay time), at 1.5T or 3T, it is reasonably accurate to use the single compartment model.
Model Free Approach
As an alternative to the kinetic modeling described above, a model-free approach can be used.15 The philosophy behind model-free perfusion quantification is that, instead of prior assumptions about bolus shape and the tissue’s residue function, the actual bolus or arterial input function is measured along with the tissue signal. This allows for extraction of the tissue residue function and thereby perfusion by means of deconvolution, a concept extensively used in dynamic contrast-agent based methods.16 A requirement is that temporal information of both the arterial signal as well as the tissue signal exists. In general, the tissue concentration of a tracer in the voxel under observation as a function of time can be described by the following convolution integral:

where, Ct(t) is the tissue concentration, ft the tissue flow, Ca(t) is the arterial tracer concentration entering the voxel, and R(t) is the residue function describing the fraction of tracer molecules remaining in the voxel after time t (i.e., the difference between arterial influx and venous outflow at each point in time). Ca(t) is also called the arterial input function (AIF). This definition naturally implies that R(t) must start with 100% of the arriving tracer available at the beginning (R(t = 0) = 1) and that this amount of tracer subsequently decreases toward zero as the tracer washes out of the observed voxel through the venous outlet. The term ftR(t) can therefore be found by deconvolving Ct(t) by Ca(t) and the tissue’s flow Ft subsequently found from ftR(t = 0) due to the aforementioned property of the residue function being 1 at t = 0. The neat property of this deconvolution procedure is that no prior assumptions about the residue function are required, making it model free as long as both the arterial signal feeding the voxel and the actual tissue signal are measured.
Note that for arterial spin labeling, these tracer concentrations are essentially magnetization differences between the “control” and “label” experiments seen over time. If we look at Equation 9, it can be seen that the tissue concentration, ΔM(t), is described by the same convolution integral as shown in Equation 10. Here, ΔM(t) is the result of the convolution of the arterial signal, Ma(t), with the combined residue function r(t) and m(t). The additional term m(t) is added to account for the fact that that our magnetic labeled tracer decays over time. This is contrary to gadolinium-based perfusion methods, where the tracer mass remains constant during the entire experiment. We do not have conservation of “tracer mass” in our ASL experiment. Nevertheless, the same properties apply to r(t)m(t) as for R(t) that r(0)m(0) = 1. The perfusion, f, can therefore be found in a similar manner by deconvolution on a voxel-by-voxel basis.
The extraction of the information needed for this procedure, ΔM(t) and Ma(t), requires a bit more than what is measured by most standard available ASL sequences. Traditionally, only a single inversion time point ΔM(TI) after labeling is imaged and perfusion is estimated by assuming the characteristics of Ma(t), r(t), and m(t). The inversion time TI is chosen such that the bolus will have enough time to clear from the arterial compartment such that only the tissue perfusion is measured. For the model-free approach, multiple time points are needed, and this is typically achieved using a fast Look-Locker–based approach to ensure feasible scan times. A caveat of a multiple time point acquisition is that the arterial blood would not have had time to leave the arterial compartment when short TIs are used, meaning that instead of only measuring the tissue contribution, ΔM(t), it is ΔM(t) + Ma(t) · aBV, which is actually measured (Fig. 30.6). Here, aBV is the arterial blood volume fraction (not to be confused with total blood volume) in the measured voxel. However, the vascular contribution can be eliminated from the signal by the use of vascular crusher gradients. They are essentially bipolar diffusion-encoding gradients that dephase the signal from labeled spins moving faster than a preset velocity threshold.17 Spins moving perpendicular to the crusher gradient direction will not be dephased and therefore crushing in multiple directions is performed and averaged to keep the arterial contribution at a
minimum. Note that venous signal can be neglected as by the time the label arrives here it will have fully decayed. This means that a crushed multiple time point experiment measures the tissue signal, ΔM(t), and a noncrushed experiment measures ΔM(t) + Ma(t) · aBV, thereby allowing the extraction of the arterial signal Ma(t) · aBV from the subtraction of the two experiments. Weighting Ma(t) by aBV is done to get the concentrations right as the spins are located in the 1% to 2% blood volume. We will call this the fractional arterial input function fAIF(t) = Ma(t) · aBV. However, for absolute flow quantification, it is AIF(t) = Ma(t), which is needed (Equations 6 through 8) and therefore scaling with aBV is necessary. The aBV can be estimated by comparing the area under the measured fAIF(t) curve with the area under the calculated curve for Ma(t), given in Equation 8, provided the T1 relaxation time of blood, the equilibrium magnetization of arterial blood Ma0, the labeling efficiency α, and the bolus duration τ are known.
minimum. Note that venous signal can be neglected as by the time the label arrives here it will have fully decayed. This means that a crushed multiple time point experiment measures the tissue signal, ΔM(t), and a noncrushed experiment measures ΔM(t) + Ma(t) · aBV, thereby allowing the extraction of the arterial signal Ma(t) · aBV from the subtraction of the two experiments. Weighting Ma(t) by aBV is done to get the concentrations right as the spins are located in the 1% to 2% blood volume. We will call this the fractional arterial input function fAIF(t) = Ma(t) · aBV. However, for absolute flow quantification, it is AIF(t) = Ma(t), which is needed (Equations 6 through 8) and therefore scaling with aBV is necessary. The aBV can be estimated by comparing the area under the measured fAIF(t) curve with the area under the calculated curve for Ma(t), given in Equation 8, provided the T1 relaxation time of blood, the equilibrium magnetization of arterial blood Ma0, the labeling efficiency α, and the bolus duration τ are known.
A sequence that takes advantage of this is the quantitative STAR labeling of arterial regions (QUASAR) implementation,15,18 which interleaves both crushed and noncrushed control-label pairs at multiple time points. After the separation of ΔM(t) and Ma(t), the flow is found by deconvolution, typically using the singular value decomposition method, along with some regularization to keep the solution sensible.16 The presence of added acquisition and physiologic noise in the signal often makes the matrix inversion, which is part of the deconvolution, an ill-posed problem.
![]() FIGURE 30.7. Average maps from the QUASAR reproducibility study18 for (A) cerebral blood flow (CBF), (B) arterial blood volume (aBV), (C) arterial transit time (ATT), and (D) Gaussian dispersion (σ). |
An advantage of the multiple time point acquisition and in particular the interleaved acquisition of crushed and noncrushed data is the added information available in addition to the blood flow. From Figure 30.6 it can be seen that we have information about the arterial transit time (ta). From the time the bolus arrives at the voxel, at ta, it travels farther through smaller and smaller vessels toward the capillary network. The time it takes to reach vessels with flow velocities less than the preset crusher velocity can be measured as the upslope of the crushed experiment. We could call this the microvascular transit time (tm). Because the fractional arterial input function is measured on a voxel-by-voxel basis, then we can also extract the arterial blood volume, aBV, simply by integrating the area under fAIF(t) and correct it for the T1 decay of arterial blood15:

During the blood’s passage through the vasculature, it is exposed to resistance from the vasculature itself. As a result, the labeled bolus will get dispersed or blurred during its passage of the vascular tree and the farther or the narrower vessels the bolus has to traverse, the more dispersed it becomes. Therefore, different regions have different degrees of dispersion, and the effect is known to worsen especially in patients with vascular disease. By assuming some patterns of dispersion, such as the ones proposed by Hrabe et al.,19 one can investigate the amount of dispersion in different brain regions. An example of such a dispersion map fitted from the Ma(t) maps is shown in Figure 30.7.20 It should be noted that ta, tm, aBV, and the dispersion maps all can be extracted without the need of deconvolution, but rather using simple subtraction, integration, fitting, and slope detection algorithms. The results of the deconvolution, r(t)m(t), also include information about the kinetic properties r(t) of the system
in addition to cerebral blood flow (CBF). However, the relaxation properties m(t) are mixed up with it so the interpretation is not straightforward. Future studies will need to be done to investigate the clinical usefulness of this information.
in addition to cerebral blood flow (CBF). However, the relaxation properties m(t) are mixed up with it so the interpretation is not straightforward. Future studies will need to be done to investigate the clinical usefulness of this information.
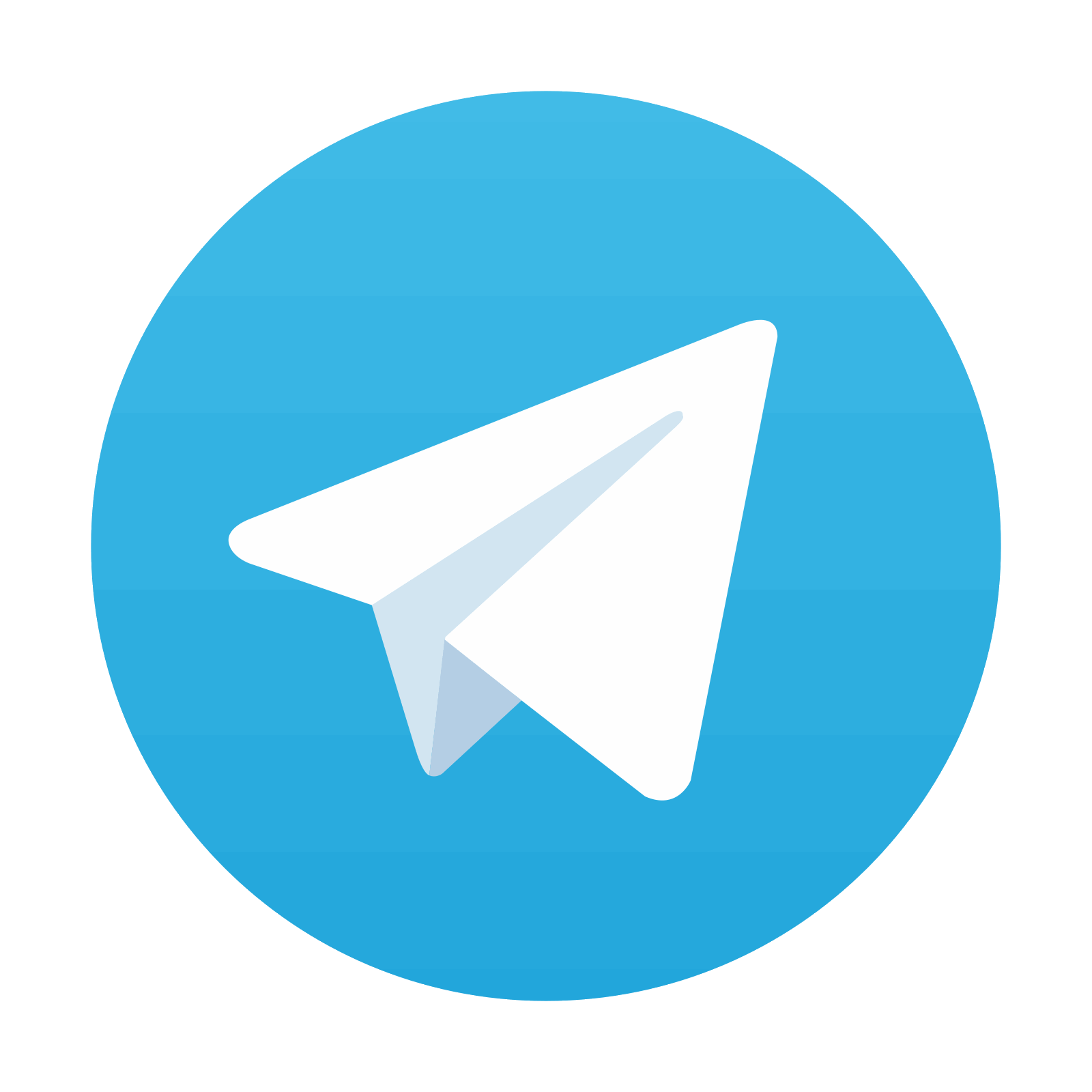
Stay updated, free articles. Join our Telegram channel

Full access? Get Clinical Tree
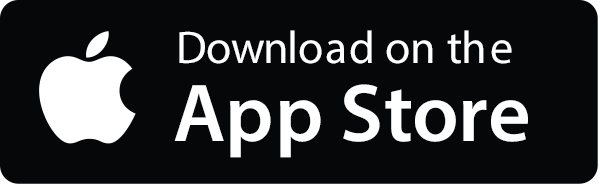
