The Bayesian approach to data analysis dates to the Reverend Thomas Bayes, who published the first Bayesian analysis (reprinted in Barnard 1958 ). Initially, Bayesian computations were difficult except for simple examples, and applications of Bayesian methods were uncommon until Adrian F. M. Smith began to spearhead applications of Bayesian methods to real data. Bayesian applications to science and medicine have exploded in the past 20 years (cf Berger 2000 ) because of the development of flexible and robust computational algorithms (Markov chain Monte Carlo ).
Unlike classical statistical methods, Bayesian statistical methods for analysis of ophthalmologic data directly incorporate expert ophthalmologic knowledge in estimating unknown parameters. For example, suppose that in a small sample of glaucoma patients the mean intraocular pressure (IOP) is 30 mm Hg but that it is known a priori that IOP in glaucoma patients is centered on 25 mm Hg. A Bayesian analysis incorporates this information into its inference, and would estimate the population mean to be somewhat less than 30 mm Hg, perhaps 29 mm Hg, a weighted average of the data estimate 30 mm Hg and the expert ophthalmologic knowledge of 25 mm Hg.
Recently I used a Bayesian analysis to investigate an unpublished HIV logistic regression analysis. The original analysis used maximum likelihood, one of several classical approaches to estimation. In the maximum likelihood analysis a particular regression coefficient had an estimate of 4.4 with a standard error of 2.1 corresponding to an odds ratio (OR) of 79.8 and 95% confidence interval (CI) of (1.27, 5014). The result is statistically significant; the question is whether this enormous estimate and gigantic CI reflects a real effect or is an artifact caused by limited data. (The specific application was trying to predict unprotected sex as a function of methamphetamine use and time.)
From long experience, I know a priori that in logistic regression, coefficients of binary (0–1, dichotomous or dummy) predictors are usually in the range −1 to 1 and rarely outside the range (−2 to 2). To encode this particular piece of prior information formally into a Bayesian analysis, a common approach specifies in advance of and independently of the data that the unknown regression coefficient has a Gaussian prior distribution with prior mean 0 and prior standard deviation 1. This prior distribution says that 68% of all logistic regression coefficients should be in the interval (−1,1) and 95% of all logistic regression coefficients should be in the interval (−2,2). Using this prior distribution, the Bayesian analysis estimates the regression coefficient to be .80 with a standard error of .9. The corresponding odds ratio is 2.2 with a 95% CI (.38, 13), a nonsignificant result much more in line with the prior information. The nonsignificant Bayesian result has a smaller standard error, a more believable point estimate, and narrower confidence interval that contains more believable values in the OR confidence interval than the classical maximum likelihood inference. As a sensitivity analysis, I tried a number of other prior standard deviations besides 1, ranging from 1/8 to 2, and all give a nonsignificant result. The Bayesian result is nonsignificant in contrast to the traditional maximum likelihood result; my advice to my colleagues was to not report the original result as it was an artifact caused by limited data.
Bayesian estimation is also called shrinkage estimation and Bayesian methods generally give more stable estimates with smaller standard errors by allowing expert prior information to be incorporated directly into the analysis. In the ophthalmologic example, the IOP sample mean of 30 mm Hg was shrunk towards 25 mm Hg; in the HIV example, the maximum likelihood estimate of 4.4 was shrunk strongly towards the null value of 0.
Statistical modeling requires a scientific question, a relevant data set, and a statistical model that links the data to the scientific issue. Given the Bayesian statistical model and the data, Bayesian inferences follow directly; there is only 1 Bayesian conclusion. In contrast, given a model and data set, classical statisticians must make choices from a bewildering menu of methodologies, not all of which are fully fleshed out or easily explained. Even in the case of comparing 2 groups, should one use a t test, a rank test, a signed rank test, or a robust alternative? It can be simpler to specify and execute a Bayesian inference than a classical inference.
Classical computational software is extremely elaborate. Bayesian software, while much younger and less complicated, tends to be rather flexible and unified; this will no doubt change as Bayesian software matures. Currently, the most popular Bayesian package is WinBUGS, which can fit most models likely to be seen in a 2-year biostatistics master’s degree program. SAS Institute has just released a Proc MCMC (SAS Institute, Cary, North Carolina, USA) to allow general Bayesian modeling, and there are several additional SAS procedures that allow explicit Bayesian modeling. Several recent texts teach Bayesian computation using the high-quality free statistical package R.
Bayesian methods have numerous advantages over classical methods. Small data sets can be successfully analyzed with a concomitant decrease in nonsensible and extreme answers, as with the HIV analysis, and “couldn’t be analyzed” results occur more rarely. That doesn’t mean you will get significant results more often, but small data sets can be investigated for the information they do contain. Hierarchical models for fitting hierarchical and nested data are naturally Bayesian.
Classical statistics has difficulty with inference in many situations. Recent Bayesian successes provide solutions for problems that are difficult for classical approaches, including multiple imputation for missing data, model and variable selection, and hierarchical models. Classical hypothesis testing has many restrictions: it requires specifying a null hypothesis (H 0 : mu = 0) and an alternative hypothesis (H A : mu > 0); the null hypothesis is a limiting or special case of the alternative. Bayesian hypothesis testing can simultaneously consider 2 or more hypotheses all at 1 time (for example, H 1 : mu < 0, H 2 : mu = 0, H 3 : 0 < mu < 10, and H 4 : 10 < mu). Scientific discussions of a particular Bayesian analysis center on what assumptions are sensible and appropriate; classical inference discussions must also include discussions of appropriate statistical methodology; the choice of estimation method can be influential on final conclusions.
Bayesian methods are not a panacea. What model to use in a given analysis can be subject to intense discussion and dispute in both Bayesian and classical inference. Two statisticians may well disagree about the best approach for a given data set and as knowledge and experience in an area expands, model complexity will likely expand. For complicated data sets, the appropriate model may be incompletely understood. Bayesian and classical analyses are subject to modeling choices made for convenience; unthinking usage of a given Bayesian model is just as bad as unthinking usage of a classical model.
In Bayesian analysis, expert scientific opinion is encoded in a probability distribution for the unknown parameters; this distribution is called the prior distribution . The data are modeled as coming from a sampling distribution given the unknown parameters. The conclusion of the analysis is the posterior distribution , a compromise between the prior information and the data information. In addition to previous citations, there are other popular advanced Bayesian texts. Ophthalmology has plenty of opportunities for active application of Bayesian methods, and collaboration with a statistician expert both in Bayesian methods and in the particular models and data set under analysis can be extremely helpful. Grab a Bayesian and get to work!
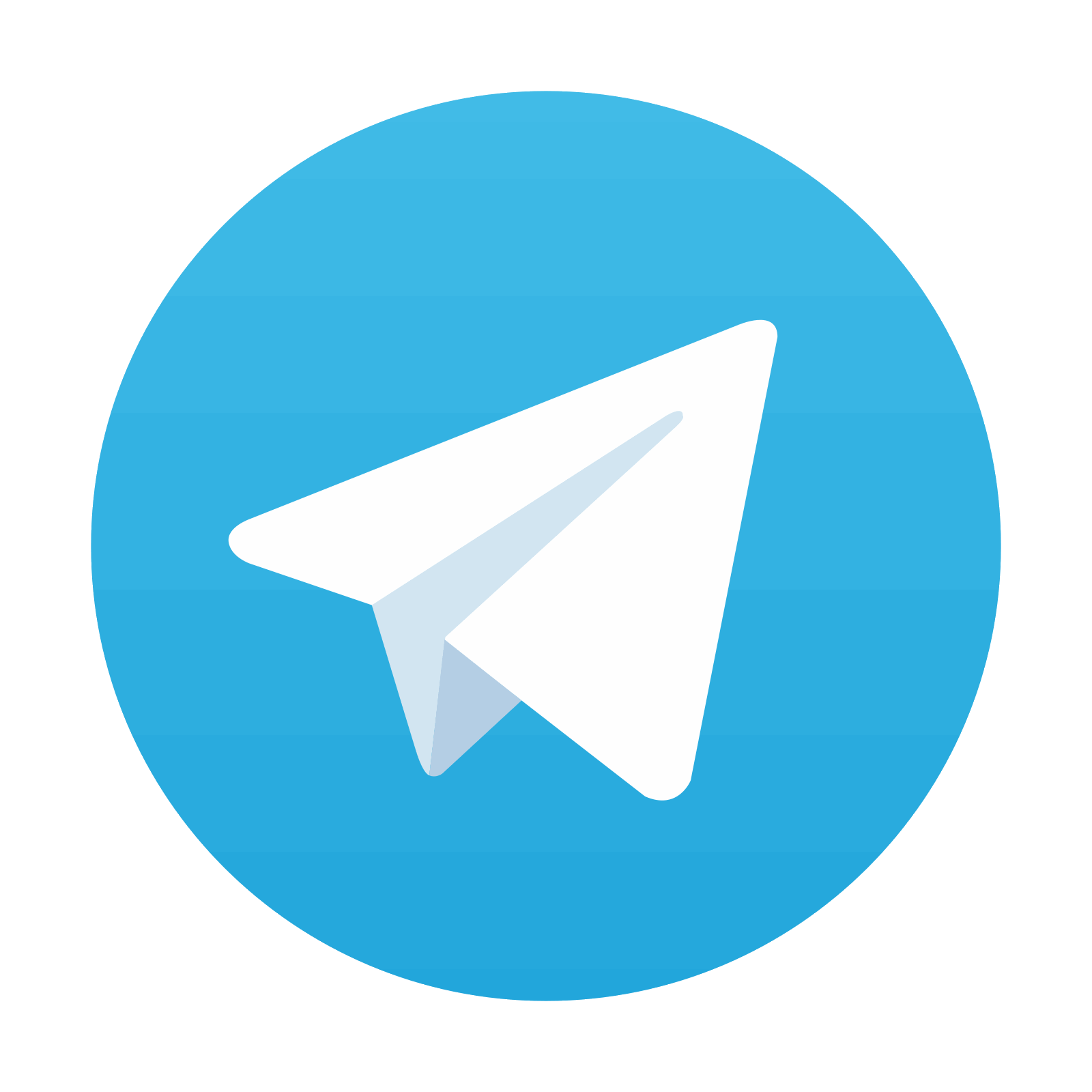
Stay updated, free articles. Join our Telegram channel

Full access? Get Clinical Tree
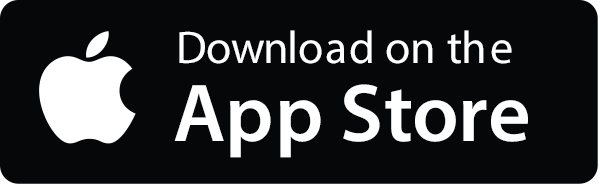
