In recent years, significant strides have been made in the field of ocular neoplasm imaging. This dynamic area of research has witnessed the convergence of various advanced imaging modalities and computational techniques, revolutionizing the diagnosis, characterization, and management of ocular malignancies. This article aims to critically examine the latest advances in orbital neoplasm imaging, encompassing the latest developments in imaging instrumentation, image processing algorithms, and clinical applications, with a focus on improving early detection, treatment planning, and prognostication for patients with orbital tumors.
Key points
- •
Diffusion-weighted imaging (DWI) Utility in Orbital Tumors: DWI) complements traditional MRI, aiding in tumor differentiation based on water diffusion patterns, and enhancing diagnostic accuracy and treatment planning.
- •
Artificial intelligence (AI)-assisted diagnosis: AI, particularly deep learning, assists radiologists in accurate tumor identification and classification using MRI and computed tomography scans, improving diagnostic efficiency and consistency.
- •
Advancements in MRI sequences: Dynamic contrast-enhanced MRI and steady-state sequences provide superior diagnostic precision, aiding in the differentiation between benign and malignant lesions, and enhancing information for surgical planning.
- •
Integration of DWI and AI: The combination of DWI and machine learning holds promise for further improving orbital tumor imaging, providing unique insights into tissue characteristics and enhancing diagnostic accuracy through complex data analysis.
Introduction
Recent strides in imaging technology have catalyzed a paradigm shift in the evaluation and treatment of orbital tumors, affording clinician’s unprecedented levels of diagnostic precision and therapeutic guidance. Among the arsenal of imaging modalities, Computed Tomography (CT) and MRI reign supreme, offering complementary insights into the intricate anatomy and pathology of the orbit. CT imaging operates on the principles of radiographs attenuation, wherein a rotating radiographs beam generates cross-sectional images of the orbit by measuring the differential absorption of radiographs by various tissues. This technique excels in delineating bony structures and calcifications within the orbit, making it indispensable for identifying osseous lesions, such as intraosseous tumors or fractures. Furthermore, CT imaging facilitates the assessment of tumor size, margins, and bony erosion, crucial for treatment planning and surgical decision-making. Conversely, MRI harnesses the properties of magnetic fields and radiofrequency pulses to generate high-resolution images of soft tissues within the orbit. By exploiting differences in tissue relaxation times, MRI provides exquisite contrast resolution, enabling the differentiation of orbital tumors from adjacent structures based on their inherent signal characteristics. Moreover, MRI offers multiplanar imaging capabilities, allowing clinicians to visualize tumors from various perspectives and accurately assess their extent and relationship with critical orbital structures. Advanced MRI sequences (including T1-weighted, T2-weighted, and contrast-enhanced imaging) afford comprehensive characterization of orbital masses, elucidating their composition, vascularity, and enhancement patterns [ ].
In essence, understanding the underlying principles of CT and MRI imaging is paramount for clinicians navigating the complex landscape of orbital tumor diagnosis and management. By leveraging the unique strengths of each modality, clinicians can formulate tailored treatment strategies, optimize surgical planning, and enhance patient outcomes in this challenging clinical arena. This article focuses on 3 aspects of recent advances in orbital imaging: the renewed interest in diffusion-weighted imaging (DWI) for orbital tumors, the application of artificial intelligence (AI) and deep-learning models for orbital imaging and other newer MRI sequences and their relevance to orbital tumor imaging.
Use of diffusion-weighted imaging for orbital tumors
While gMRI offers superior contrast resolution for soft tissues, standard sequences may not always effectively differentiate between various orbital masses and pseudotumors. Hence, it is crucial to explore supplementary MRI techniques that offer alternative forms of tissue contrast, potentially assisting in both the identification and further characterization of these lesions. Recently, there has been a lot of interest in the use of DWI to study orbital lesions. DWI is a specialized MRI technique that measures the random motion of water molecules within tissues. It capitalizes on the principle of Brownian motion, where water molecules exhibit random thermal motion in tissues. In DWI, the MRI machine applies a series of magnetic field gradients that sensitize the images to the movement of water molecules. The working of DWI can be simplified into the following headings.
- •
Gradient Application : During DWI, gradient pulses are applied along different directions, altering the magnetic field experienced by water molecules. This process is known as diffusion weighting.
- •
Water Movement Sensitivity : Water molecules in tissues exhibit different levels of diffusion depending on tissue characteristics such as cellularity, membrane integrity, and tissue structure. In areas, where water diffusion is restricted, such as in cellular structures or densely packed tissues, the signal on DWI appears reduced.
- •
Measurement : The MRI scanner measures the degree of signal attenuation caused by diffusion across multiple gradient directions, allowing for the quantification of diffusion within tissues.
- •
Apparent Diffusion Coefficient (ADC) Mapping : ADC mapping is often performed as a post-processing step in DWI. It calculates a quantitative measure of the rate of water diffusion within tissues. Decreased Brownian motion, which may be caused by cellular swelling or increased density of cells, is captured on DWI as a hyperintense signal. True diffusion restriction is represented by a high DWI signal and corresponding low signal on the ADC map, such as in lymphoma, stroke, cytotoxic, change, or other hypercellular tumors [ ].
DWI is particularly valuable in clinical practice for its ability to detect and characterize various pathologies based on their tissue properties. For example, acute ischemic stroke lesions typically exhibit restricted diffusion because of cytotoxic edema, making DWI an essential tool for early stroke diagnosis. In the context of orbital imaging, DWI can aid in the differentiation of lesions based on their cellular density and tissue composition, providing additional insights into their nature and behavior [ ]. Studies have shown that indicates that DWI and ADC assessments are useful in predicting the malignancy of orbital tumors, distinguishing orbital lymphoma from pseudotumors, and assessing orbital cellulitis ( Fig. 1 ).

In a study by Sepahdari and colleagues, source data from 3 previous studies of orbital mass DWI were aggregated, and additional published data points were gathered. In this study, receiver operating characteristic (ROC) analysis was performed to determine the sensitivity, specificity, and optimal ADC thresholds for distinguishing benign from malignant masses. They reported that while there was no single ADC threshold that characterized orbital masses as benign or malignant with high sensitivity and specificity. An ADC of less than 0.93 × 10 −3 mm 2 /s was more than 90% specific for malignancy, and an ADC of less than 1.35 × 10 −3 mm 2 /s was more than 90% sensitive for malignancy. With these 2 thresholds, 33% of this cohort could be characterized as “likely malignant,” 29% as “likely benign,” and 38% as “indeterminate.” [ ] Additionally, they reported that there were significant differences between benign and malignant lesions, though with notable overlap. However, it was seen that ADC was highly accurate in distinguishing lymphoma from inflammatory disease. Maldonado and colleagues in their more recent study to quantify DWI characteristics of orbital lesions suggested that the use of a cut-off value of 0.99 × 10 −3 mm 2 /s allowed for the better differentiation of the benign lesions and malignant lesions with a sensitivity of 75% and a specificity of 100%. However, the cut-off point of 1.26 × 10 −3 mm 2 /s had a sensitivity of 100% and a specificity of 73% [ ].
Phuttharak and colleagues retrospectively reviewed patients with orbital masses who underwent DWI and had histopathologic results. They, however reported that they found no statistical difference between the ADC values of benign and malignant tumors (1.18 vs 0.99×10 −3 mm 2 /s; P -value 0.200). They concluded based on their data, that the ADC values were useful in differentiating between myxoid versus non-myxoid orbital masses and metastatic non-myxoid versus non-metastatic non-myxoid orbital masses. The myxoid tumors had significantly higher ADC values than the non-myxoid (1.58 vs 0.99; P -value 0.008) tumors. Non-metastatic non-myxoid tumors also had significantly lower ADC values than metastatic non-myxoid tumors (0.73 vs 1.32; P -values 0.006) [ ].
Typically, the effective clinical application of DWI in assessing an orbital mass hinges on the specific diagnostic challenges posed by other clinical and imaging findings. For instance, distinguishing between lymphoma and atypical lymphocytic infiltrate, or other inflammatory orbital conditions, can be a common diagnostic conundrum, wherein DWI demonstrates considerable utility. Similarly, in pediatric patients, discriminating between infantile hemangioma (capillary hemangioma) or vascular malformation and rhabdomyosarcoma can be challenging; in such scenarios, DWI appears particularly well-suited for the task [ ].
In conclusion, DWI emerges as a valuable adjunct to traditional MRI techniques in the evaluation of orbital tumors. Its ability to capture tissue characteristics based on water diffusion patterns provides crucial insights into the nature and behavior of lesions, aiding in their differentiation and characterization. DWI, along with ADC measurements, shows promise in predicting tumor malignancy, distinguishing between various types of orbital masses, and assessing treatment response. While challenges remain, particularly in establishing universal thresholds for malignancy and addressing diagnostic overlaps, DWI offers significant potential in enhancing diagnostic accuracy and guiding treatment decisions. Its integration into clinical practice represents a promising avenue for improving patient care and outcomes in the management of orbital tumors.
Artificial intelligence and deep-learning
AI has emerged as a transformative tool in the field of radiology, particularly in the interpretation and diagnosis of medical imaging studies such as MRI and CT scans. With advancements in machine learning algorithms, particularly deep learning (DL) techniques like convolutional neural networks (CNNs), AI systems can analyze vast amounts of imaging data with remarkable accuracy and efficiency. In the context of MRI and CT scans, AI-powered computer-assisted diagnosis (CAD) systems aid radiologists in detecting, characterizing, and diagnosing various pathologies, including tumors, lesions, and abnormalities. These systems typically operate through several stages, including image pre-processing, feature extraction, and classification, leveraging AI algorithms to recognize patterns and anomalies within the imaging data. CAD systems in radiology serve as invaluable decision support tools, assisting radiologists in interpreting complex imaging studies and providing more accurate and timely diagnoses. By automating repetitive tasks and flagging areas of concern, AI enhances radiologists’ productivity and enables them to focus on critical cases requiring their expertise. Moreover, AI-based CAD systems have the potential to improve diagnostic accuracy and consistency, reduce interpretation errors, and enhance patient outcomes. Through continuous learning and refinement, these systems adapt and evolve, incorporating new data and insights to further enhance their performance over time.
Allam and colleagues introduced a CAD system designed to identify various types of orbital tumors using CNNs. The system comprises 3 main stages: pre-processing, data augmentation, and classification. The effectiveness of the proposed system was assessed using 2 datasets of MRI images, totalling 1404 MRI T1-weighted images and 1560 MRI T2-weighted images. The findings demonstrate the system’s ability to accurately detect and classify tumors in both types of images, achieving a recognition rate of 98% for T1-weighted images and 97% for T2-weighted images [ ].
Nakagawa and colleagues assessed the usefulness of a CNN-based deep learning technique for the diagnosis of orbital invasion, using CT images. In this study, a total of 168 lesions associated with malignant nasal or sinonasal tumors were categorized into a training set (n = 119) and a separate test set (n = 49). The definitive diagnosis (either invasion-positive or -negative) was established by experienced radiologists who meticulously examined all CT images. Employing a CNN-based deep learning approach, a section of the square target region containing the orbital bone wall was extracted and utilized in a deep-learning training session to construct a diagnostic model. This process involved transfer learning with the Visual Geometry Group 16 model. Subsequently, the test set underwent evaluation with CNN-based diagnostic models, as well as by 2 other radiologists with non-specialization in head and neck radiology. Approximately 2 months after the initial reading session, the same 2 radiologists re-evaluated all images within the test set, considering the diagnoses provided by the trained CNN-based diagnostic model. The results showed that the CNN-based diagnostic models achieved a diagnostic accuracy of 0.92, whereas the 2 radiologists’ diagnostic accuracies during the initial reading session were 0.49 and 0.45, respectively. In the subsequent reading session, with the radiologists aided by the CNN-based diagnostic model, significant improvements in diagnostic accuracy were noted, with values of 0.94 and 1.00, respectively [ ].
A common clinical dilemma for physicians is being able to differentiate radiologically between a cavernous hemangiomas or orbital cavernous venous malformations (OCVM) and other similar well-circumscribed tumors such as solitary fibrous tumors or orbital schwannomas ( Figs. 2 and 3 ). There have been recent attempts at harnessing the power of machine learning to help differentiate these 2 groups of lesions. Han and colleagues published the outcomes of their study, which was a study aimed to assess the utility of non-enhanced CT images in distinguishing between OCVM and non-OCVM, and to determine the most effective radiomics-based machine learning (ML) algorithm for this task. A retrospective analysis of 215 OCVM cases and 120 non-OCVM cases was conducted. The data were divided into training and testing sets, and various feature selection techniques and ML models were evaluated to develop an optimal classification model. Statistically, significant differences were found in density and tumor location between OCVM and non-OCVM groups. Linear regression and Stochastic Gradient Descent classifiers, with f-test and L1-based feature selection, demonstrated the best performance. Support vector machine (SVM), Random Forest, and eXtreme Gradient Boosting classifiers, combined with the F-test method, also showed strong performance. The study concludes that non-enhanced CT images can assist ophthalmologists in lesion identification and localization, and radiomics-based ML algorithms enhance qualitative diagnosis in OCVM cases [ ].
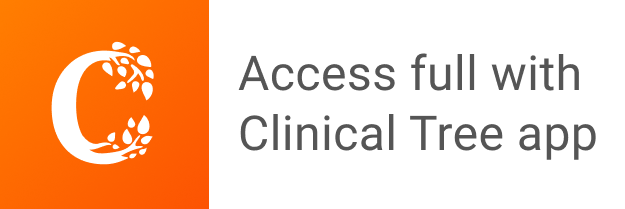